Artificial Intelligence's Impact on Biotechnology
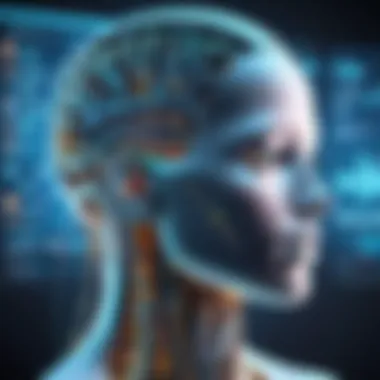
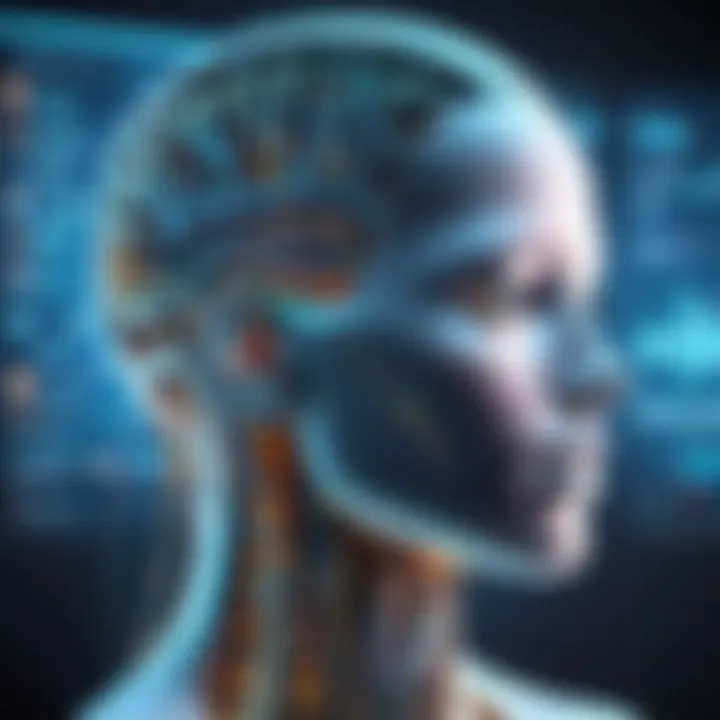
Intro
Artificial Intelligence (AI) is no longer just a buzzword; it's becoming the backbone of many industries. In biotechnology, a field that's constantly evolving, AI stands out as a major force driving innovation. From drug discovery to personalized medicine, the integration of AI promises a revolutionary shift, helping professionals unlock new potentials and approach challenges with unprecedented efficiency.
In this article, weāll delve into the various ways AI influences biotechnology. We'll explore current applications, ethical considerations, and what the future might hold, giving professionals and curious readers a thorough understanding of where we stand today and where weāre headed.
As we navigate this complex landscape, itās essential to grasp the nuances that make AI a game changer in biotech. Letās unravel the core components, trends, and innovations shaping this dynamic field.
Foreword to Biotechnology
Biotechnology is at the forefront of scientific innovation, carving new pathways in health, agriculture, and environmental sustainability. This article aims to illuminate the intricate ties between biotechnology and artificial intelligence, showing how they blend to create a brighter future. From providing plagues to the pharmaceutical world to fostering sustainable farming practices, biotechnology is indispensable in tackling some of the most pressing problems today.
Understanding biotechnology means diving into how biological systemsābe it cellular processes or genetic mechanismsācan be harnessed for real-world applications. At its core, biotechnology connects biology with technology, and the possibilities it offers are nothing short of astounding.
Definition of Biotechnology
Biotechnology refers to the manipulation of living organisms or their components to develop products or solve problems. This can range from using yeast to brew beer to the complex synthesis of insulin in labs. The definition expands as we explore how we can harness the abilities of microbes, plants, and animal cells for diverse applications.
In light of modern advances, biotechnology has evolved, encompassing areas like genomics, molecular biology, and tissue engineering. For instance, farmers now use genetically modified crops that resist pests, reducing the need for harmful pesticides. This not only benefits the environment but also boosts food security, showcasing the dual triumph of science and nature.
Historical Context
The roots of biotechnology run deep, stretching back thousands of years. The ancient Egyptians relied on fermentation processes to make beer and bread, demonstrating primitive forms of biotechnology. Fast forward to the early 20th century, scientists like Gregor Mendel laid the groundwork for genetics, observing how traits pass through generations.
By the 1970s, the advent of molecular cloning heralded a new chapter, enabling the direct manipulation of DNA. A notable landmark was the creation of the first genetically engineered organism. This paved the way for transformative developments, such as the production of human insulin using genetically modified bacteria.
Biotechnology is not merely a discipline; it's a narrative flowing through time, linking history to the present and future, continually challenging our perceptions of life itself.
As we reflect on these milestones, one can appreciate how biotechnology has morphed into a complex interplay of scientific inquiry and practical application, setting the stage for the significant role that AI will play in advancing this exciting field. The synergy between biotechnology and artificial intelligence isnāt just a trend; it holds the promise to redefine our understanding of both biology and technology.
Understanding Artificial Intelligence
Artificial Intelligence (AI) is a cornerstone that propels biotechnology into the future. The intersection of these two fields has gained immense attention over recent years, as the capacity of AI to analyze vast datasets, recognize patterns, and make informed predictions is reshaping how researchers approach biological challenges. Grasping the essence of AI is vital for anyone looking to understand its transformative implications in biotechnology. It is not just a tool; itās a game changer, bringing forth opportunities and challenges that require careful consideration.
Definition of Artificial Intelligence
Artificial Intelligence refers to the simulation of human intelligence processes by machines, particularly computer systems. It encompasses a broad suite of technologies that enable computers to perform tasks that typically require human-like cognitive functions such as understanding language, learning from experience, reasoning, and self-correction. In biotechnology, AI plays a critical role, assisting in everything from data analysis to the design of new biological systems. The crucial point here is that AI isn't merely an accessory; itās integral to innovation ā enabling biotechnologists to sift through enormous amounts of data that would be insurmountable for the human brain alone.
Types of AI Technologies Used in Biotechnology
As we delve deeper into the relationship between AI and biotechnology, it's essential to explore the various AI technologies that are making waves in this sector. Three prominent types include:
- Machine Learning
Machine learning, a subset of AI, focuses on the development of algorithms that allow computers to learn and improve from experience without explicit programming. It is the backbone of many biotechnological advances today. For example, in drug discovery, machine learning models can predict the effectiveness of compounds based on their molecular structure. The key characteristic of machine learning is its reliance on data ā the more it has, the better it performs.The unique feature of machine learning is its adaptability. Its ability to refine its algorithms as it processes more data means that its predictions can become increasingly accurate over time. This is particularly beneficial in a field where precision is paramount. However, one notable disadvantage is the requirement of vast datasets, which can be a hurdle in certain specific research areas where data may not be readily available. - Natural Language Processing
Natural Language Processing (NLP) involves the capability of machines to understand and interpret human language as it is spoken or written. In the realm of biotechnology, NLP can aggregate and synthesize information from countless research articles, grant proposals, and clinical trial data. This ability to process language means that relevant information can be gleaned quickly, which is critical given the rapid pace of discovery in the field.The strength of NLP lies in its efficiency; it saves enormous amounts of time that scientists would otherwise spend sifting through text. However, this technology is not without its weaknesses ā nuances in language can sometimes lead to misunderstandings or misinterpretations, complicating the extraction of knowledge from written sources. - Computer Vision
Computer vision allows machines to interpret and understand visual information from the world. In biotechnology, this technology is crucial for analyzing visual data from biological experiments, such as imaging from cellular structures or high-content screening in drug development. The core feature of computer vision is its capacity to recognize patterns autonomously from images, which is significant for accelerating processes like quality control in manufacturing biological products.One advantage of computer vision is that it automates tasks that would otherwise be manual and time-consuming, significantly speeding up research workflows. However, it can be resource-intensive and may require high-quality image data; poor-quality images can lead to inaccurate results, making it less effective in scenarios where data quality is suspect.
In summary, understanding the types of AI technologies utilized in biotechnology allows us to appreciate how they contribute to solving complex biological problems, streamlining processes, and fostering innovation, while also considering their limitations.
AI in Drug Discovery
The landscape of drug discovery has undergone a significant transformation, largely due to the contributions of artificial intelligence. AI serves as an essential tool in streamlining an otherwise complex and often time-consuming process of bringing new pharmaceutical interventions to market. This section will thoroughly explore the combination of computational prowess and biopharmaceutical innovation that AI offers, shedding light on its essential elements, advantages, and key considerations.
Accelerating the Drug Development Process
Drug development is traditionally marked by lengthy and costly trials, with studies indicating it can take over a decade and billions of dollars to develop a single new drug. Here's where AI comes into play, drastically altering the timeline and expense involved. By applying advanced algorithms, researchers can swiftly analyze vast datasetsāfrom genomics to chemical librariesāto identify potential drug candidates.
- Data Integration: AI tools can manage and draw insights from various data sources. They can aggregate clinical data, biological information, and even patient feedback, providing a holistic view that informs decisions.
- Informed Decisions: Algorithms that utilize machine learning can predict compounds' efficacy much faster than classic methods. This predictive capability allows researchers to focus resources on the most promising candidates much earlier in the development cycle.
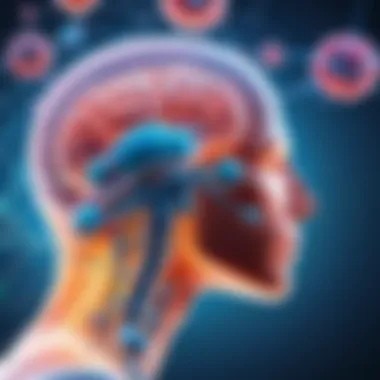
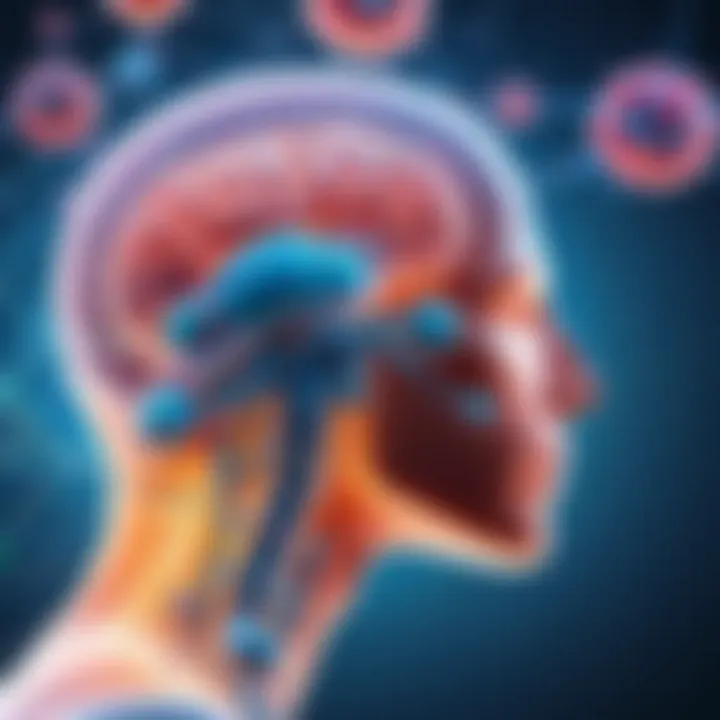
It's noteworthy that in the current landscape, machine learning models are not just augmenting human capabilities but are often driving preliminary decisions, thus allowing researchers to concentrate on refining leads rather than searching for them.
Predictive Modeling in Pharmacology
Predictive modeling has surfaced as one of the standout applications of AI in pharmacology. Instead of relying on trial and error, scientists can lean on models that project how different compounds might interact within biological systems.
This approach involves utilizing historical data to train algorithms that predict drug behavior, side effects, and interactions. Some impactful elements of predictive modeling include:
- Toxicity Prediction: Early identification of potential toxicity can save years of development time.
- Bioactivity Forecasting: AI significantly enhances the ability to predict how compounds affect various targets, which aids in tailoring drug designs for specific ailments more effectively.
- Patient Varieties: Understanding the genetic variations among patients provides another layer of predictive modeling, allowing researchers to anticipate how different patients will respond to a given drug.
By using these predictive models, biopharmaceutical organizations not only mitigate risks but also enhance the safety profile of the compounds being developed.
Case Studies of Successful AI Applications
The integration of AI into drug discovery is not just theoretical; there are tangible examples highlighting its effectiveness.
- Atomwise: This company utilizes deep learning algorithms to analyze molecular structures and guide drug discovery. Their AI system has been successful in identifying promising compounds against various diseases, including Ebola and multiple sclerosis.
- Exscientia: Known for its work in automated drug design, Exscientia has achieved milestones in creating new drugs significantly faster than traditional methods. Their AI-driven approach resulted in the discovery of a potential treatment for obsessive-compulsive disorder, showcasing the real-world efficiency AI can bring to drug discovery.
- Insilico Medicine: This firm developed an algorithm that can design a new drug compound in a matter of weeks. They completed a full cycle from drug design to clinical trials in under 18 months for a disease with no current treatmentsāan impressive feat by any standard.
These case studies illustrate just how valuable AI can be in the sector, emphasizing that its application leads to accelerated timelines and reduced costs matched with improved drug effectiveness.
"AI is transforming the drug discovery process, making it faster and more efficient than ever before."
As we continue to witness advancements in AI technologies, the pharmaceutical industry stands on the brink of unprecedented innovation driven by data.
In summary, the intersection of artificial intelligence and drug discovery lays a foundation for a future where the development of life-saving drugs can occur at an astonishing pace, substantially benefiting society.
Genetic Engineering and AI
The intertwining of genetic engineering and AI marks a significant chapter in the narrative of biotechnology. Genetic engineering pertains to the direct manipulation of an organism's genes, allowing scientists to alter traits and enhance capabilities. As we've stepped into the age of big data and advanced computational methods, artificial intelligence introduces powerful tools that revolutionize how we approach and execute genetic modifications. The synergy between these fields facilitates precision, accelerates research, and paves the way for groundbreaking applications.
The Role of AI in CRISPR Technology
AI plays a strategic role in refining the CRISPR (Clustered Regularly Interspaced Short Palindromic Repeats) technology, which has gained attention for its ability to edit genomes with exceptional accuracy. Machine learning algorithms sift through vast amounts of genomic data to identify patterns that human researchers might overlook. This means fewer off-target effects ā those pesky unintended edits that can lead to unforeseen consequences.
Some notable applications include:
- Target Identification: AI enables researchers to discover the most effective target sites for CRISPR interventions, enhancing success rates.
- Optimized Guide RNA Design: Through predictive modeling, AI enhances the selection of guide RNAs crucial for directing the CRISPR machinery to the right location in the genome.
- Species-specific Strategies: Custom AI models can cater to particular organisms, maximizing the efficacy of CRISPR tailored for each unique genetic makeup.
By employing these AI-driven strategies, researchers are embarking on a more reliable path in genetic modifications, leading to efficient solutions in treating genetic disorders and enhancing crop resilience.
"AI is the future of CRISPR technology. It turns complicated data into actionable insights, making genetic engineering smarter and safer."
Enhancing Precision in Gene Editing
Precision in gene editing is paramount. With AI's support, this precision reaches new heights. Not only does AI help reduce errors, but it enables a more strategic approach to gene modifications. Advanced bioinformatics tools powered by AI analyze millions of potential gene edits in record time, isolating the ones most likely to yield success.
The advantages of heightened precision include effective therapeutic strategies and less potential for harmful side effects in medical applications. In agriculture, this means developing crops that withstand disease or evolve to thrive in harsh climates without disrupting the ecosystem.
Key benefits include:
- Increased Efficiency: Automated workflows can process genetic data faster than traditional methods.
- Reduced Risk: AI enables in silico testing, allowing researchers to simulate modifications before actual laboratory work, reducing trial and error.
- Enhanced Customization: Tailored approaches foster a more individualized strategy for therapeutic applications, addressing specific patient needs.
As genetic engineering leans into AI technology, the future looks promising for both healthcare innovations and agricultural advancements.
Personalized Medicine Through AI
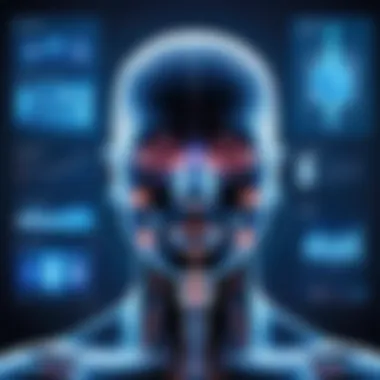
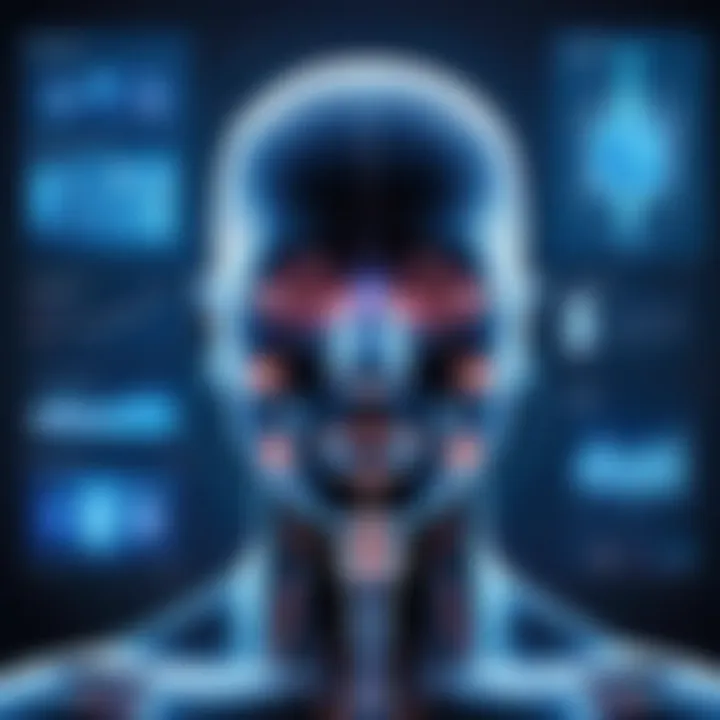
The integration of artificial intelligence into personalized medicine is rapidly evolving how healthcare professionals approach treatment plans. Traditional medicine often takes a one-size-fits-all approach, where drugs and therapies are prescribed based on generalized guidelines. However, AI brings a transformative capability of turning patient-specific data into tailored treatments, resulting in improved outcomes and decreased adverse effects. In this section, we will unpack the various facets of personalized medicine influenced by AI, emphasizing its significance, advantages, and crucial considerations in practice.
Tailored Treatment Plans
AI technologies are enabling the development of tailored treatment plans, which take into account individual patient characteristics such as genetics, lifestyle, and response to previous treatments. With access to big data and advanced algorithms, healthcare providers can analyze vast datasets to identify patterns and make predictions about which treatment protocols will work best for a specific individual.
Some key benefits of tailored treatment plans include:
- Enhanced Efficacy: By predicting how a patient will respond to a particular medication, doctors can prescribe more effective regimens, increasing the likelihood of successful treatment.
- Reduced Side Effects: With a better understanding of a patientās unique biology, it is possible to minimize drug interactions and unwanted side effects, improving overall patient quality of life.
- Cost-Effectiveness: While personalized medicine may seem costly upfront, it can save healthcare systems money in the long run by reducing the trial-and-error approach commonly seen in traditional medicine.
AI's Role in Understanding Patient Data
Artificial intelligence plays a pivotal role in gathering and interpreting complex patient data. Instead of relying solely on human analysis, AI systems enable faster data integration from various sources, including electronic health records, genetic testing, and wearable health technology. These systems utilize machine learning techniques to sift through mountains of data, identifying crucial information that wouldnāt be readily apparent.
The integration of AI helps in several essential areas:
- Predictive Analytics: Through historical data and algorithms, AI can forecast which patients may develop certain conditions or complications based on their current health status and genetics.
- Personal Health Records: AI allows for personalized health records that are dynamic and can adapt with new information. This keeps healthcare providers informed and agile in addressing patient needs.
- Real-Time Monitoring: AI algorithms can analyze data collected from wearable devices to monitor patient vitals continuously. This encourages proactive management of conditions, preventing emergencies before they arise.
"AI in personalized medicine is not just about technology. Itās about understanding the patient as a whole, leading to better health outcomes and optimized care strategies."
In summary, the convergence of AI and personalized medicine is set to redefine healthcareās future. By harnessing patient data more effectively and formulating tailored treatment strategies, advancements are being made that not only promise improved health outcomes but also enhance patient satisfaction and safety.
AI in Synthetic Biology
The intersection of artificial intelligence and synthetic biology represents a pivotal frontier in the scientific landscape. As researchers and innovators push the boundaries of what is achievable through biological manipulation, the infusion of AI technologies brings a new dimension of insight and capability. Synthetic biology aims to redesign organisms for useful purposes by engineering them to produce desired substances. However, the complexity of biological systems presents substantial challenges that AI is uniquely positioned to address.
Designing Biological Systems
One of the most notable roles of AI in synthetic biology is in the design and optimization of biological systems. Traditional approaches to biological design often relied on trial and error, which could be inefficient and time-consuming. However, AI can analyze vast datasets to predict how genetic changes will affect organism behavior. This capability significantly reduces the design cycle.
For instance, by utilizing machine learning algorithms, researchers can model biological pathways and predict outcomes of genetic modifications with a high degree of accuracy. This approach not only speeds up the design process but also helps in identifying unexpected interactions within the biological systems. Techniques such as deep learning can process complex genetic information, allowing scientists to make informed decisions rapidly.
Additionally, AI assists in automating the design process through tools like genetic circuit simulators, which use algorithms to create effective designs for organisms tailored for specific tasks, such as drug production or biofuel synthesis.
"AI is not just a tool but a partner in rethinking the potential of synthetic biology, enabling us to craft living systems with unprecedented precision."
AI's Contribution to Bioengineering
The contributions of AI extend into the broader realm of bioengineering, where it plays a critical role in various applications. By enhancing predictive capabilities, AI allows bioengineers to simulate biological processes before physically modifying organisms. This simulation can highlight the most promising pathways worth investigating, saving time and resources.
Moreover, AI enhances the precision of bioengineering through predictive analytics. One particular highlight is its application in metabolic engineering, where AI can help optimize metabolic pathways in engineered microorganisms to improve yield and efficiency when producing complex molecules. Machine learning algorithms can analyze existing fermentation data to find correlations between genetic alterations and product yields, thus optimizing processes for industrial applications.
Here are some key benefits of AI in bioengineering:
- Enhanced Precision: AI tools refine bioengineering methods, leading to more accurate results.
- Faster Integration: AI enables quicker adaptation of engineered systems in real-world applications.
- Data-Driven Insights: AI provides actionable insights derived from large data sets, guiding experimental designs.
- Cost Efficiency: Streamlining processes with AI leads to significant savings in materials and labor.
In summary, the integration of AI in synthetic biology and bioengineering not only transforms the methodologies employed but also expands the horizons of what can be achieved biologically. As the potential for innovation grows, so too does the necessity for thoughtful consideration of the ethical implications of these technologies.
Challenges of Integrating AI in Biotechnology
As artificial intelligence continues to reshape numerous sectors, its incorporation into biotechnology is not without hurdles. While the potential benefits are immense, recognizing and addressing the challenges is vital for progress. From data privacy concerns to regulatory compliance and ethical dilemmas, these obstacles not only impact innovation but also the trust stakeholders and consumers place in AI-driven methods.
Data Privacy Concerns
In an age where personal data is both a commodity and a vulnerability, the biotechnology sector faces significant scrutiny regarding patient and research data privacy. The integration of AI systems often necessitates the collection and analysis of extensive datasets. For instance, machine learning algorithms require vast amounts of data to recognize patterns and improve their predictions. This means sensitive data, such as genetic information or medical histories, must be handled judiciously.
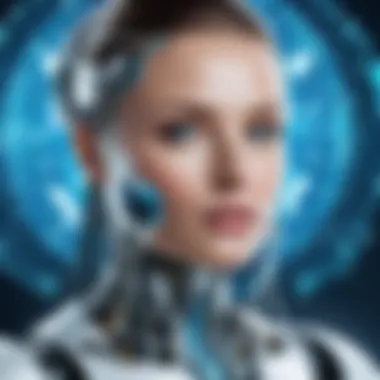
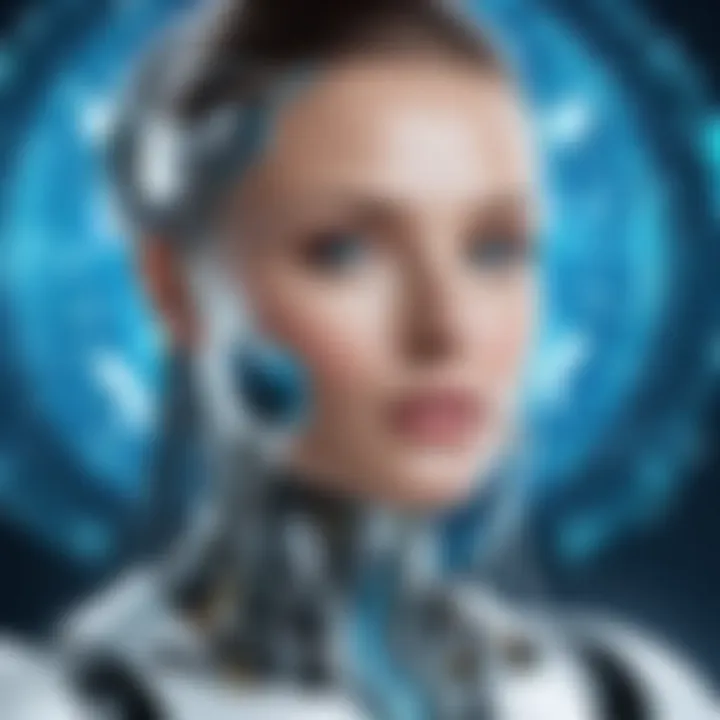
The stakes are high. Any breach or misuse of data could lead to severe consequencesānot just for individuals, but also for institutions. A simple lapse can erode public trust. To illustrate, consider a biotechnological firm using AI for drug discovery. If their systems were compromised, sensitive patient information could be leaked, causing irreparable damage to the company's reputation and paving the way for legal repercussions.
Actors in this space must implement robust cybersecurity measures and transparent data handling practices to mitigate these risks. Examples include encryption protocols and anonymization techniques to protect individual identities while still leveraging data for research purposes.
Regulatory and Ethical Issues
The rapid evolution of AI technologies has outpaced the establishment of comprehensive regulatory frameworks, presenting another layer of complexity in the integration process. Governments and regulatory bodies find themselves scrambling to develop guidelines that address the unique challenges AI presents in the biotechnology landscape.
For example, when a new AI-driven therapy emerges, determining its safety and efficacy is paramount. Yet, the regulatory processes currently in place may not be suitable for evaluating AI systems that constantly learn and evolve. This brings about a bleak situation: innovations could be stalled in bureaucratic limbo or, conversely, inadequately assessed, leading to potential public health risks.
Moreover, ethical questions arise surrounding bias in AI algorithms. If datasets are not representative or diverse, AI applications can inadvertently favor certain demographics over others, disproportionately affecting underrepresented populations. For example, algorithms trained predominantly on data from one ethnic group may falter when applied to others, resulting in less effective treatments or misdiagnoses.
In essence, while the fusion of AI and biotechnology promises groundbreaking advancements, it must be approached with a healthy dose of caution. Stakeholders across sectors must collaborate to establish governance frameworks that prioritize not only innovation but also the ethical considerations necessary for widespread acceptance.
"As we increasingly rely on AI in biotechnology, it is imperative that we safeguard against data misuse and craft regulations that align with the rapid pace of technological advancements."
Future Perspectives on AI and Biotechnology
The landscape of biotechnology is undergoing a significant transformation, driven largely by the integration of artificial intelligence. This intersection holds the potential for groundbreaking advancements, reshaping how we approach challenges in healthcare, agriculture, and environmental sustainability. Understanding the future perspectives on AI in biotechnology is vital as it not only illuminates the benefits but also the ethical considerations we must navigate as we advance.
In the coming years, we can expect several pivotal changes. For instance, as AI continues to evolve, its applications in predictive analytics will enhance our capacity for precision medicine. By crunching vast datasets, AI can uncover patterns that inform treatment strategies tailored specifically to individual patients, leading to improved outcomes and a reduction in trial and error.
Thereās also the potential for AI to greatly improve the efficiency of drug discovery processes. By automating tasks that previously took scientists weeks or months, AI-driven technologies can shorten the development timeline from years to mere days. This no only speeds up the process but could also lead to a more dynamic response to emerging health crises, such as pandemics.
Furthermore, as biotechnical innovations advance, the role of AI in managing complex biological data becomes even more indispensable. Innovations like AI-assisted genomic sequencing could facilitate more in-depth understanding of genetic disorders or even enhance crop resilience through better monitoring of plant health. This might help farmers react swiftly to diseases or pest outbreaks.
Emerging Trends in AI Technologies
Emerging trends highlight the growing synergy between artificial intelligence and biotechnology. Some key trends here include:
- Neural Networks in Genomics: Utilizing deep learning algorithms to analyze genomic data is becoming increasingly prevalent. This approach offers insights into rare genetic conditions and may reveal novel therapeutic targets.
- AI-Powered Bioinformatics: By employing advanced machine learning tools, bioinformatics can process and analyze biological data at a scale unimaginable before, unlocking new avenues in research and therapy development.
- Robotic Automation in Labs: Robotics, further enhanced by AI, are streamlining the repetitive and meticulous tasks in laboratories, allowing scientists to focus more on interpretation rather than data processing.
- Natural Language Processing for Research: AI that understands and synthesizes vast amounts of scholarly articles and data will empower researchers, providing them with a clearer direction and reducing the time spent on literature reviews.
Potential Breakthroughs and Innovations
Looking at the potential breakthroughs powered by AI, a few key areas stand out:
- Advanced Drug Formulations: With AI's capacity to run simulations and predict outcomes, new drug formulations can be developed and tested rapidly, paving the way for treatments that are safer and more effective.
- Smart Therapeutics: AI could lead to the design of 'smart drugs' that adjust their activity based on individual patient reactions, a significant leap toward personalized healthcare.
- Precision Agriculture: AI technologies may spur the next era of agriculture where crops are cultivated with minimal waste and maximum yield, utilizing resources more efficiently while reducing environmental impact.
- Vaccine Customization: AI may facilitate rapid vaccine optimization, potentially leading to custom vaccines for various strains of viruses or bacteria, tailored to specific populations or even individuals based on genetic makeup.
"In the world of biotechnology, the question is not if AI will play a role, but rather how profound that role will be in reshaping the future of health and sustainability."
As we stand on the cusp of these potential advancements, the dialogue between biotechnology and AI must also consider the ethical implications accompanying these technologies. We need to ensure responsible development and deployment in a way that maximizes benefits while minimizing risks. The next few years will be crucial as we translate potential into practice, paving the way for truly remarkable developments in how biotechnology is harnessed for global good.
Epilogue
The integration of artificial intelligence into biotechnology marks a critical juncture that not only revolutionizes existing processes but also promises to redefine the very landscape of the life sciences. Understanding the implications of AI within this realm is vital for a host of stakeholders, from researchers to healthcare professionals and policy-makers. The benefits derived from this synergy are both far-reaching and compelling, particularly in enhancing efficiency, precision, and personalized approaches in various biological applications.
Summary of Key Insights
Throughout this article, several key insights regarding the role of AI in biotechnology have emerged:
- Accelerated Drug Discovery: AI technologies streamline the drug development process by enabling rapid analysis of vast datasets. This enhances the ability to identify potential drug candidates with greater accuracy, thereby speeding up their journey from the lab to the market.
- Improved Genetic Engineering: AI-driven tools enhance the precision of gene editing techniques, particularly CRISPR. These advancements facilitate targeted modifications in genomic sequences, which is crucial for therapeutic applications.
- Personalized Medicine: AI plays an essential role in tailoring treatment strategies to individual patient profiles. By analyzing diverse datasets, AI models predict how various patients respond to specific medications, enabling highly personalized care.
- Ethical Considerations: The intersection of AI and biotechnology raises important ethical questions, particularly concerning privacy and data security. Addressing these concerns is indispensable for fostering public trust and ensuring compliance with regulations.
In essence, the collaboration between artificial intelligence and biotechnology leads to innovations that promise to tackle some of the most pressing health challenges of our time.
Final Thoughts on the Future of AI in Biotechnology
Looking ahead, the horizon is rich with possibilities as AI continues to evolve and intertwine with biotechnological advancements. The potential breakthroughs could open doors to new therapies, more efficient production methods for biologics, and even predictive models for epidemic outbreaks. However, it is also essential to remain vigilant about the ethical frameworks surrounding these technologies. As the tools of AI become more sophisticated, their application in biotechnology could have profound implications on health equity and access.
Furthermore, ongoing collaboration among scientists, ethicists, and technologists will be crucial. This collective effort can ensure that the integration of AI into biotechnology not only leads to groundbreaking innovations but also adheres to principles of ethical integrity and social responsibility.
In summary, as we stand on the cusp of this technological revolution, the engagement between AI and biotechnology holds unprecedented promise. The strides taken in these fields will foster advancements that could transform healthcare as we know it, making it imperative for all stakeholders to stay at the forefront of this evolution.