Navigating the Complexities of Artificial Intelligence Implementation in Healthcare
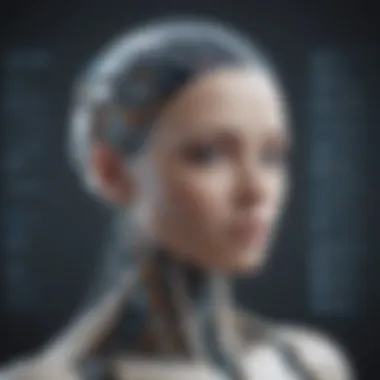
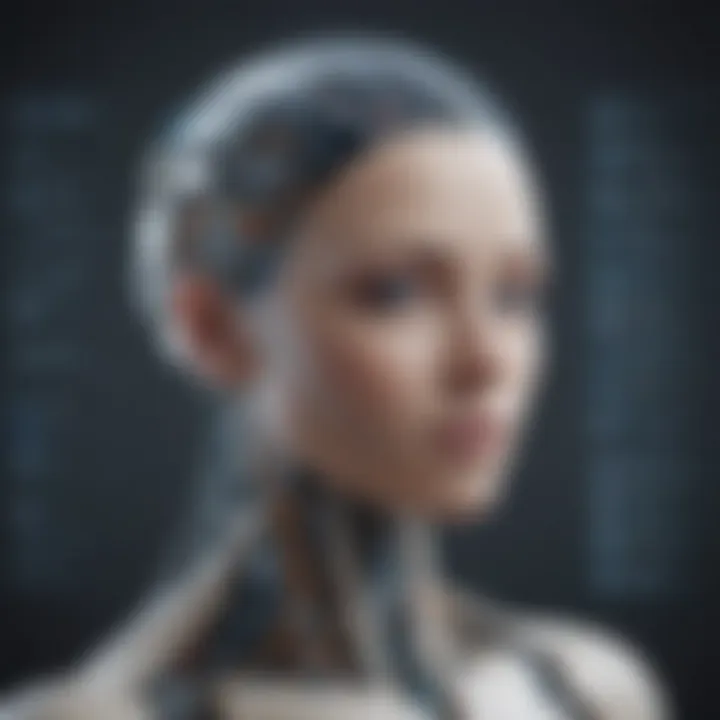
Technology Insights
Artificial Intelligence (AI) is revolutionizing the healthcare sector with its potential to enhance patient care and operational efficiency. However, the implementation of AI in healthcare comes with a myriad of challenges. From data privacy concerns to regulatory hurdles, healthcare providers face complex obstacles when integrating AI technologies into their systems. It is crucial to navigate these challenges effectively to leverage the full benefits of AI in healthcare.
Latest Tech Trends
In the realm of artificial intelligence in healthcare, staying updated on the latest tech trends is essential. Advancements such as machine learning algorithms for medical diagnostics and natural language processing for patient data analysis are shaping the landscape of healthcare AI. Understanding these trends and their implications is paramount for healthcare organizations looking to adopt AI solutions successfully.
Innovation in Tech
As AI continues to evolve, innovative solutions are emerging to address specific healthcare challenges. Robotics in surgery, predictive analytics for disease prevention, and virtual health assistants are just a few examples of how technology is revolutionizing healthcare delivery. Embracing these innovations requires healthcare providers to navigate technical complexities while ensuring compliance with regulatory standards.
Product Reviews
Evaluating and selecting AI products tailored for healthcare settings is a critical step in the implementation process. Product reviews analyzing the performance, scalability, and security of AI solutions can guide healthcare decision-makers in choosing the most suitable technologies for their organizations. Comprehensive product assessments play a crucial role in mitigating risks and maximizing the benefits of AI integration in healthcare.
Introduction
In the realm of healthcare, the integration of artificial intelligence (AI) presents a myriad of challenges that necessitate careful navigation. This section serves as a gateway to the complex landscape of AI implementation in healthcare. By delving into the multifaceted issues surrounding AI adoption, stakeholders gain a nuanced understanding of the hurdles and opportunities that arise in leveraging AI technologies for patient care and operational enhancements.
Overview of Artificial Intelligence in Healthcare
Impact of AI on Healthcare
Exploring the impact of AI on healthcare unveils a transformational shift in the industry's modus operandi. The integration of AI algorithms and machine learning capabilities revolutionizes diagnostic accuracy, treatment outcomes, and forecasting of patient health trajectories. This disruptive technology streamlines processes, enabling healthcare providers to deliver personalized and efficient care.
Rapid Advancements in AI Technology
The rapid evolution of AI technology reshapes healthcare delivery paradigms. Innovations in AI algorithms, neural networks, and deep learning mechanisms propel the sector towards predictive analytics, image recognition, and natural language processing applications. With AI's exponential growth, healthcare entities can harness predictive modeling and real-time insights for enhanced decision-making and patient outcomes.
Significance of AI in Transforming Healthcare
Improving Diagnosis and Treatment
AI's role in diagnosis and treatment optimization cannot be overstated. By leveraging AI-powered tools for data analysis and pattern recognition, healthcare professionals can expedite accurate diagnoses and tailor treatment plans according to individual patient profiles. The precision and efficiency achieved through AI optimize clinical pathways, leading to improved patient outcomes.
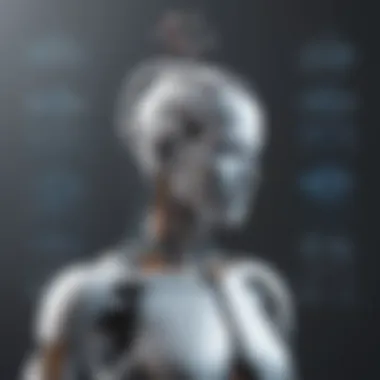
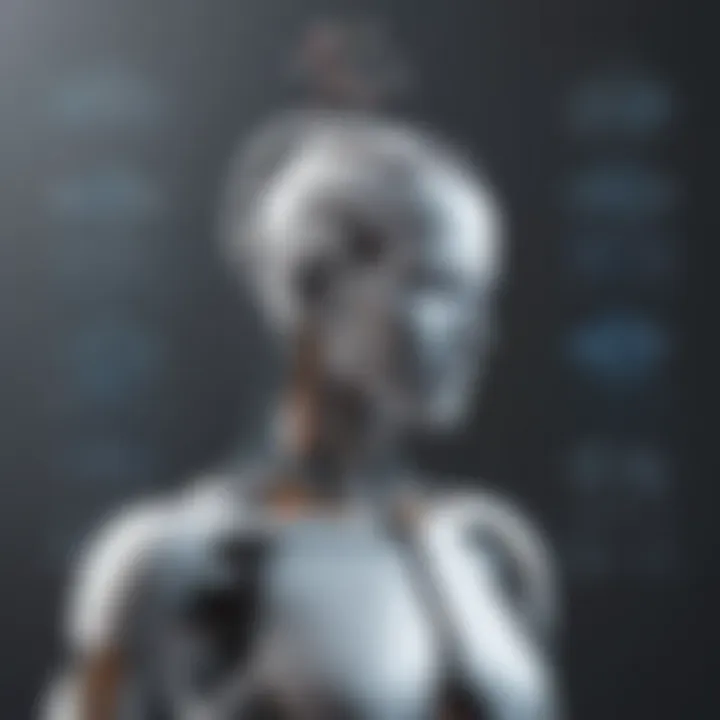
Enhancing Operational Efficiency
The integration of AI technologies streamlines healthcare operations, enhancing efficiency and resource allocation. AI-driven solutions automate administrative tasks, optimize scheduling processes, and facilitate inventory management, enabling healthcare facilities to operate seamlessly. Through AI's operational enhancements, healthcare organizations optimize workflows, reduce redundancies, and improve patient care delivery.
Ethical Concerns
When delving into the intricacies of artificial intelligence implementation in the realm of healthcare, the prominent theme that emerges is the ethically charged landscape in which these advancements operate. Ethical concerns play a crucial role in shaping the narrative of AI integration in healthcare - they are the moral compass guiding decisions and actions. One of the paramount elements that necessitate intense scrutiny within this context is the ethical implications surrounding patient data privacy and security, a facet that demands meticulous attention and robust safeguards to protect sensitive information.
Data Privacy and Security
Issues of Patient Confidentiality
Patient confidentiality emerges as a pivotal concern within the broader spectrum of data privacy and security in healthcare AI applications. The sacred trust between healthcare providers and patients hinges on safeguarding sensitive medical information. Issues of patient confidentiality reflect the core essence of ethical responsibility and care, underscoring the need for stringent measures to uphold patient privacy rights. The critical characteristic of maintaining patient confidentiality lies in fostering trust and integrity within healthcare relationships, ensuring that patient information is shielded from unauthorized access or breaches.
Risk of Data Breaches
Addressing the risk of data breaches stands as a critical pillar in fortifying the security framework of AI-driven healthcare systems. The looming threat of data breaches poses a substantial challenge to maintaining the confidentiality and integrity of patient data. The key characteristic of mitigating the risk of data breaches lies in implementing robust encryption protocols and access controls, reducing vulnerabilities that could lead to inadvertent exposure of sensitive patient information. Despite the advantages of digitizing healthcare data for streamlined access and analysis, the susceptibility to data breaches necessitates stringent cybersecurity measures and preemptive strategies to avert potential breaches.
Bias and Fairness
Unintended Discrimination
The issue of unintended discrimination amplifies the ethical dimension of AI applications in healthcare, shedding light on the inadvertent biases embedded within algorithmic decision-making processes. The critical characteristic of addressing unintended discrimination lies in recognizing and rectifying algorithmic biases that may perpetuate disparities in healthcare outcomes. Circumventing unintended discrimination mandates a comprehensive assessment of algorithmic inputs and outcomes to rectify entrenched biases and ensure equitable care delivery across diverse patient populations.
Ensuring Equitable AI Algorithms
Equity in AI algorithms forms the bedrock of fostering fair and unbiased healthcare systems, emphasizing the importance of designing algorithms that prioritize fairness and eliminate discriminatory patterns. The unique feature of ensuring equitable AI algorithms lies in promoting transparency and accountability in algorithmic decision-making, dismantling barriers that impede equitable healthcare delivery. By championing fairness and impartiality in algorithmic design, healthcare stakeholders can cultivate a culture of inclusivity and equality, mitigating the risk of perpetuating systemic biases in healthcare services.
Technical Challenges
In the realm of implementing Artificial Intelligence (AI) in healthcare, technical challenges play a pivotal role in shaping the efficacy and practicality of AI-driven solutions. These challenges encompass a spectrum of considerations that impact the integration, performance, and outcomes of AI applications within healthcare systems. Addressing technical challenges is paramount to ensure the seamless operation and optimization of AI technologies for improved patient care and operational efficiency.
Interoperability Issues
Integration with Existing Systems
Integration with existing systems stands as a foundational aspect of addressing interoperability challenges in healthcare AI implementation. This process involves the seamless assimilation of AI technologies with pre-existing infrastructures and software used in healthcare settings. The key characteristic of integration lies in its ability to streamline processes, enhance data accessibility, and facilitate cross-system communication, ultimately leading to a more cohesive and interconnected healthcare ecosystem. The adoption of integration practices is a significant choice in this article due to its role in overcoming siloed data structures and enabling comprehensive data analysis for informed decision-making. While integration brings about improved system efficiency and data interoperability, it may introduce complexities in system configuration and require meticulous planning to avoid disruptions in workflow.
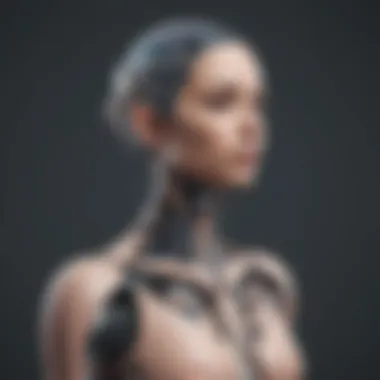
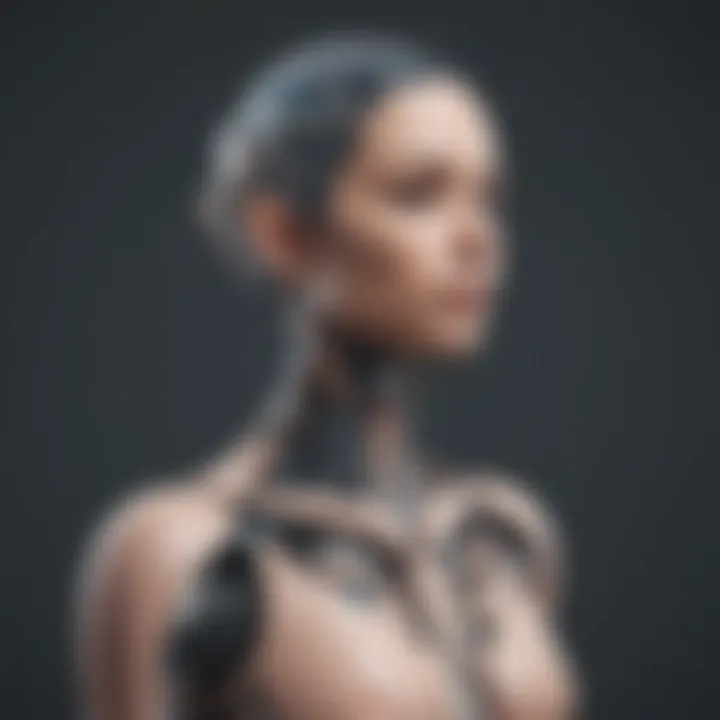
Standardization of Data Formats
Standardization of data formats emerges as a critical element in mitigating interoperability challenges within healthcare AI implementation. This practice involves establishing uniform data structures, coding systems, and information exchange protocols to ensure seamless data transmission and compatibility across different platforms and systems. The key characteristic of data format standardization lies in its capacity to promote data consistency, improve data accuracy, and enable harmonized data exchange between disparate systems. Standardizing data formats is a favorable choice for this article as it fosters data harmonization, simplifies data integration processes, and facilitates interoperability among diverse healthcare IT systems. Despite its benefits, data format standardization may pose challenges related to data migration, data conversion, and compatibility issues when interfacing with non-standardized systems, necessitating robust data governance measures and ongoing maintenance to sustain effective data standardization practices.
Lack of Transparency
Lack of transparency presents a notable challenge in the implementation of AI in healthcare, raising concerns about the opacity surrounding AI algorithms, decision-making processes, and outcomes. This lack of transparency hampers the interpretability and accountability of AI systems, posing ethical and practical dilemmas within healthcare settings. Addressing transparency issues is crucial to instill trust, foster clinical acceptance, and ensure the ethical use of AI technologies for patient care and healthcare operations.
Black Box Problem
The black box problem characterizes the inherent opacity of AI algorithms, where the decision-making processes of AI systems are not easily interpretable or explainable to end-users. This lack of insight into how AI systems arrive at specific conclusions or recommendations undermines trust, transparency, and accountability in healthcare AI applications. The key characteristic of the black box problem is its enigmatic nature, which complicates clinicians' and patients' understanding of AI-generated insights and treatments. While the black box problem offers computational efficiency and robust performance in AI models, it poses challenges in justifying decisions, validating outcomes, and ensuring error detection and correction within healthcare contexts.
Understanding AI Decision-Making
Enhancing understanding of AI decision-making processes is vital to address transparency challenges in healthcare AI implementation. This involves deciphering the logic, reasoning, and factors influencing AI algorithms' decisions to enhance interpretability, accountability, and trust in AI-driven healthcare solutions. The key characteristic of understanding AI decision-making lies in elucidating the rationale behind AI-driven decisions, promoting transparency, and enabling stakeholders to comprehend and validate AI-generated outputs effectively. Leveraging insights into AI decision-making is a beneficial choice for this article as it empowers clinicians, administrators, and patients to engage meaningfully with AI technologies, make informed decisions, and ensure ethical AI practices in healthcare delivery. Despite its benefits, understanding AI decision-making may demand sophisticated interpretive tools, algorithmic explainability strategies, and rigorous validation methods to enhance transparency and foster user confidence in AI-driven healthcare interventions.
Regulatory and Legal Hurdles
In the realm of artificial intelligence implementation in healthcare, Regulatory and Legal Hurdles stand out as pivotal challenges that demand meticulous consideration and navigation. These obstacles play a critical role in shaping the landscape of AI integration within the healthcare sector, offering both opportunities and barriers for advancement. When delving into Regulatory and Legal Hurdles, it is essential to comprehend the intricate web of regulations and laws governing the use of AI technologies in healthcare settings.
Compliance with Regulations
FDA Approval Process
The FDA Approval Process holds significant importance within the domain of AI implementation in healthcare. This process serves as a regulatory framework to evaluate the safety and efficacy of AI-driven healthcare solutions before they enter the market. Its primary objective is to safeguard patient well-being and ensure that AI technologies meet stringent quality standards. The FDA Approval Process acts as a gatekeeper, filtering out potentially harmful or ineffective AI applications, thus enhancing the overall quality of healthcare services. Despite its rigorous nature, the FDA Approval Process provides a streamlined pathway for legitimate AI developers to introduce innovative solutions with confidence and credibility in the healthcare arena. This process adds a layer of assurance for both healthcare providers and patients, fostering trust in AI-driven healthcare initiatives.
HIPPA and Data Protection Laws
HIPAA and Data Protection Laws play a vital role in safeguarding patient confidentiality and data security in the context of AI implementation in healthcare. These laws establish strict guidelines for the collection, storage, and sharing of patient data, ensuring utmost privacy and protection against unauthorized access. By adhering to HIPAA regulations and data protection laws, healthcare organizations can mitigate the risks of data breaches and uphold the trust of patients in AI-powered healthcare services. One of the key characteristics of HIPAA and data protection laws is their proactive approach to anticipating and addressing potential vulnerabilities in AI systems, minimizing the likelihood of data misuse or exposure. While compliance with these regulations may pose challenges in terms of operational workflows and technical configurations, the benefits of data security and patient trust far outweigh the complexities involved.
Liability and Accountability
Responsibility for AI Errors
The concept of Responsibility for AI Errors highlights the importance of establishing clear lines of accountability in cases where AI technologies make critical errors. This aspect underscores the need for defining roles and responsibilities among stakeholders, including developers, healthcare providers, and regulatory bodies, to address and rectify AI-related errors promptly. By delineating specific responsibilities for AI errors, the healthcare industry can better manage the risks associated with AI implementation and foster a culture of accountability and continuous improvement. Emphasizing responsibility for AI errors not only mitigates potential harms to patients but also encourages transparency and learning from mistakes to refine AI algorithms and applications continuously.
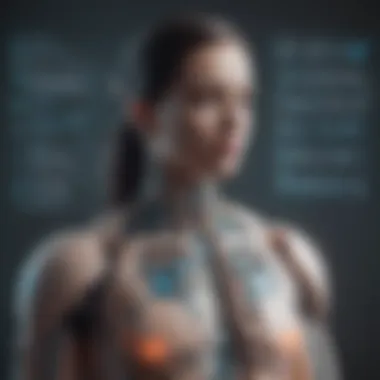
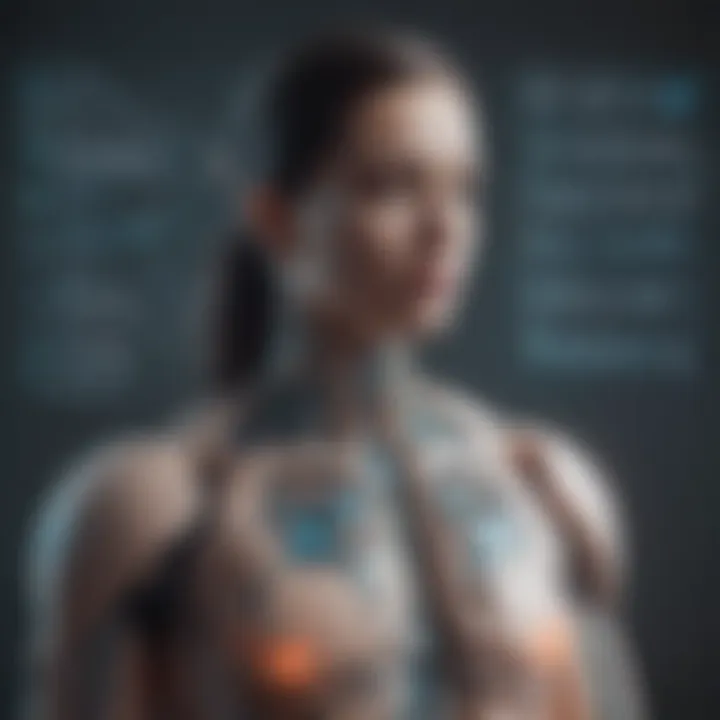
Lack of Clear Legal Frameworks
The Lack of Clear Legal Frameworks poses a multifaceted challenge in the realm of AI implementation in healthcare. This issue revolves around the ambiguity and gaps in existing legal structures to address emerging ethical and regulatory dilemmas inherent to AI technologies. The absence of clear guidelines and frameworks creates uncertainties regarding liability, data governance, and decision-making processes within AI-powered healthcare systems. While this challenge complicates the implementation of AI solutions, it also highlights the pressing need for collaborative efforts among lawmakers, industry experts, and stakeholders to develop robust legal frameworks that align with the evolving landscape of AI in healthcare. Despite its complexities, addressing the lack of clear legal frameworks is imperative to ensure accountability, fairness, and ethical conduct in leveraging AI for healthcare benefits.
User Acceptance
In the realm of artificial intelligence implementation in healthcare, the topic of User Acceptance holds paramount significance. It delves into how healthcare professionals, particularly physicians, embrace or resist AI technologies in their daily practices. User Acceptance not only impacts the successful incorporation of AI systems but also influences the quality of patient care and operational efficiency within medical settings.
Understanding the elements of User Acceptance is crucial. Factors such as user interface design, ease of integration with existing workflows, and compatibility with clinical autonomy play pivotal roles in determining how readily healthcare providers adopt AI solutions. Ensuring smooth User Acceptance leads to enhanced collaboration between physicians and AI technologies, ultimately leading to improved healthcare outcomes and patient experiences.
Physician Resistance
Impact on Clinical Autonomy:
The Impact on Clinical Autonomy is a critical aspect of Physician Resistance towards AI implementation in healthcare. Physicians value their ability to make autonomous decisions based on their expertise and experience. When AI recommendations challenge or override these decisions, it can lead to a sense of loss of control and professional judgment, impacting the overall quality of care delivered to patients.
The key characteristic of Impact on Clinical Autonomy lies in the delicate balance between leveraging AI insights for better outcomes and maintaining the doctor's autonomy in crucial medical decisions. While AI can offer data-driven suggestions, physicians must retain the authority to interpret and apply these recommendations in a way that aligns with their clinical judgment and patient-centered care.
The unique feature of Impact on Clinical Autonomy is its potential to streamline decision-making processes and improve diagnostic accuracy. However, this increased reliance on AI algorithms may raise concerns about the erosion of traditional medical values, leading to potential conflicts between the human touch in medicine and the precision of AI technologies.
Cognitive Dissonance with AI Recommendations:
Cognitive Dissonance with AI Recommendations reflects another aspect of Physician Resistance in adopting artificial intelligence in healthcare. This phenomenon occurs when doctors encounter discrepancies between their own assessments and the suggestions provided by AI systems, leading to psychological discomfort and skepticism towards the technology.
The key characteristic of Cognitive Dissonance with AI Recommendations lies in the clash between human intuition and machine-generated insights. Physicians may struggle to trust AI algorithms fully, particularly in complex medical scenarios where empathy, subjective judgment, and contextual understanding play crucial roles in decision-making.
The unique feature of Cognitive Dissonance with AI Recommendations is its potential to trigger valuable discussions on the limitations and potentials of AI tools in healthcare. While AI can analyze vast amounts of data and offer insights, reconciling these insights with the nuances of individual patient cases remains a challenge that demands further exploration and refinement in AI-physician collaboration in healthcare settings.
Conclusion
Addressing the Challenges
Implementing Ethical AI Practices
Delving into the abstruse realm of Implementing Ethical AI Practices within the healthcare milieu, one unearths a profound necessity for ethical fortitude. This facet embarks on the journey of infusing moral rectitude into the algorithmic fabric of AI, engendering a symphony of ethical prowess. The intrinsic value of Implementing Ethical AI Practices lies in its capacity to engender trust and credibility within the labyrinthine corridors of healthcare AI. Its unique attribute lies in its ability to foster a culture of transparency and integrity, thus serving as a fulcrum for ethical AI deployment. However, amidst the virtuous aura that surrounds this practice, one must not overlook the inherent challenges and complexities that accompany ethical AI implementation, paving the way for a nuanced dialogue on the coalescence of ethics and technological innovation.
Collaboration between Stakeholders
The symbiotic intertwining of Collaboration between Stakeholders within the realm of AI assimilation in healthcare unveils a tapestry of interconnected synergies. This aspect accentuates the essence of collective effort and shared vision towards harnessing the transformative power of AI in healthcare. At its core, Collaboration between Stakeholders serves as a beacon of unity, aligning divergent interests towards a common goal of optimizing patient care through technological augmentation. Its distinctive nature lies in the harmonization of disparate voices and perspectives, culminating in a collective symphony of progress and innovation. Yet, beneath the veneer of collaborative utopia, one cannot dismiss the intricacies and challenges inherent in stakeholder engagement, navigating through a maze of opinions and interests to forge a cohesive path forward in the realm of AI healthcare integration.
Awareness and Training Programs
Engaging in a meticulous examination of Awareness and Training Programs within the ambit of AI penetration in healthcare unravels a tapestry of educational intricacies. This dimension accentuates the pivotal role of knowledge dissemination and skill enhancement in fostering a culture of AI literacy within the healthcare fraternity. The hallmark characteristic of Awareness and Training Programs lies in their ability to empower healthcare professionals with the requisite skill set and awareness to navigate the AI-centric healthcare landscape adeptly. Their unique feature encompasses a dual-pronged approach, combining theoretical acumen with practical training methodologies to equip healthcare stakeholders with the tools necessary for symbiotic coexistence with AI technologies. The advantages of such programs resonate in the amplification of AI proficiency amongst healthcare personnel; however, the nuanced landscape of educational initiatives also bears inherent challenges in ensuring comprehensive adoption and sustained learning amidst the dynamic evolution of AI in healthcare.