Analyzing Data Trends in Technology and Design
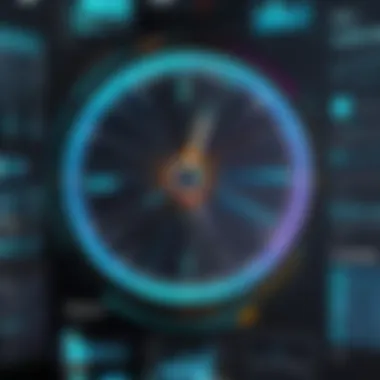
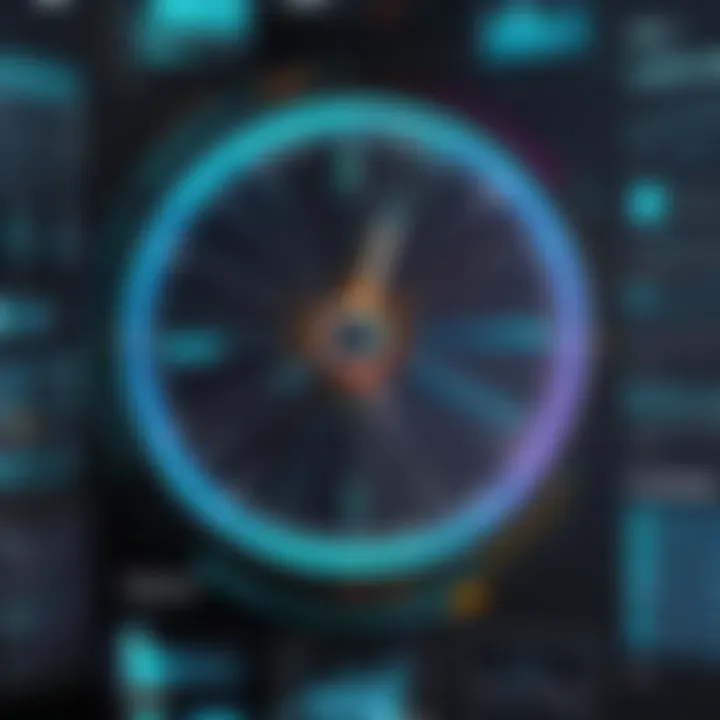
Intro
As we delve deeper, we will touch upon the latest advancements and challenges. Data serves as the backbone of decision-making, guiding professionals in crafting future possibilities. The goal here is not just to present data, but to distill it into meaningful insights that resonate with tech-savvy individuals and early adopters who thrive on being ahead of the curve.
"In the era of big data, it’s not what you have, but how you interpret it that makes the difference."
This exploration is designed to inform and inspire, sparking informed discourse within these dynamic realms. Readers will discover how data analysis illuminates crucial aspects of technology trends, from the rise of artificial intelligence to the latest gadget launches. Furthermore, they will encounter the shifting landscapes of entertainment, revealing what captures audiences in a digitally dominated world. Lastly, design will be examined, showcasing creativity and innovation that adapt to an ever-changing environment.
So, buckle up as we chart a path through the intricate dance between data and contemporary trends.
Intro to Data Analysis
In a world awash with data, it becomes paramount to understand the mechanisms that underpin data analysis. This section serves as the cornerstone for grasping how data informs a multitude of contemporary trends. The sheer volume of data generated daily presents both challenges and opportunities, fostering a landscape where understanding data is not just beneficial but necessary. From tech innovations to shifting cultural paradigms, the way we analyze and interpret data shapes our perceptions and decisions in remarkable ways.
As data demands our attention, the question fundamentally shifts from simply collecting it to knowing how to leverage it properly. The benefits of effective data analysis are manifold; organizations can spot patterns, gauge audience sentiment, and ultimately enhance their decision-making processes. Without a doubt, neglecting the power of data could mean missing out on crucial insights, hindering progress in various fields.
Defining Data in Context
Data does not exist in a vacuum; its significance is derived from the context in which it is applied. Understanding data requires an awareness of the surrounding factors that give it meaning. Contextualizing data involves examining aspects like the source of the data, the methodology used for collection, and the intended audience. For instance, customer purchase histories provide invaluable insights for businesses, yet their utility is contingent on the businesses grasping the context of consumer behavior. If a company simply looks at data points without acknowledging broader trends in societal behavior, it risks formulating strategies that miss the mark.
Different domains interpret data in varied ways based on their specific objectives. In the healthcare sector, clinical trial data is meticulously analyzed to ensure patient safety and treatment effectiveness. Conversely, marketing teams might focus on engagement metrics to determine content strategy. Understanding these nuances ensures that data is harnessed effectively and responsibly.
Importance of Data in the Digital Age
A digital age is, by its very definition, a data-driven epoch. The importance of data has only magnified with the explosion of technology and access to information. Businesses can now make decisions grounded in quantitative evidence rather than gut feelings, which is fundamentally transformative.
Consider how social media platforms are real-time data goldmines. Each interaction—be it a like, share, or comment—provides explicit feedback on audience preferences and behavior. This wealth of information allows companies to tailor products and services, ensuring alignment with user expectations. The strategic insights derived from data not only guide marketing campaigns but also inform product development and audience engagement strategies.
"Data is the new oil"—its value lies not just in its existence but in its refinement into actionable insights.
To thrive in today’s landscape, organizations must prioritize data literacy among employees, embedding data-focused practices into their cultures. It is this synthesis of analysis and application that will determine future success, paving the way for informed strategies that resonate within the ever-evolving marketplace.
Overview of Data Collection Techniques
Data collection forms the backbone of data analysis. It's like digging a well; if you don’t tap into the right sources, your insights will run dry. Understanding the different ways data can be gathered is key for practitioners interested in uncovering trends, identifying patterns, or spotting anomalies in a sea of raw numbers. This section will explore various techniques that are essential in obtaining qualitative and quantitative data. Here, we’ll delve into popular methods such as surveys, questionnaires, and user tracking as well as the distinct merits and challenges inherent to each.
Effective data collection not only enhances the quality of the insights derived but also minimizes the risks of potential bias. Choosing the right method is akin to choosing the right tool for a job—the outcome relies heavily on this decision.
Qualitative vs. Quantitative Data
When considering data collection strategies, two primary types emerge: qualitative and quantitative data. Both of these approaches serve different purposes, fitting into the broader framework of analysis like pieces of a puzzle.
- Qualitative Data: This type involves subjective information that can unravel the complexities of human experience. It encompasses insights collected through interviews, open-ended surveys, or focus groups. It’s not just about what people say; it’s about understanding the context behind their responses. For example, consider a tech company interested in user satisfaction. By conducting in-depth interviews, they might discover why users prefer certain features, revealing motivations behind their choices that mere numbers would never show.
- Quantitative Data: On the flip side, quantitative data is numerical and can be statistically analyzed. Think of it as the concrete evidence in a courtroom—hard facts that can stand on their own. This type of data is often collected through structured surveys with predefined options or through devices that capture metrics in real-time. A practical example is an e-commerce site analyzing sales figures to identify trends over a quarter. Here, the numbers tell a clear story about what is or isn’t working.
Both qualitative and quantitative data are vital, offering a rounded perspective that informs decision-making. Only understanding one side of the coin leaves a gap, potentially letting crucial insights slip through the cracks.
Surveys and Questionnaires
Surveys and questionnaires are time-tested techniques for gathering data across different demographics. These instruments can be finely tuned to extract specific information, making them versatile and efficient. However, crafting effective questions is key.
- Benefits: Surveys can reach a vast audience in a relatively short amount of time, and when done correctly, they can yield high response rates. Utilizing online platforms enables businesses to aggregate data quickly, providing real-time insights that help to pivot strategies on the fly.
- Considerations: Poorly designed questions can lead to skewed data. Yes-no questions can oversimplify complex ideas, while jargon-filled queries may confuse respondents.
In best practices, questions should be clear, concise, and aimed at eliciting honest responses. Consider a company that wants to evaluate user feedback on a new software feature. A survey can include direct questions like "How satisfied are you with the new feature?" alongside open-ended options like "What improvements would you suggest?" This blend taps into both qualitative and quantitative data effectively.
Web Analytics and User Behavior Tracking
In today’s digital age, understanding user behavior online is paramount. Web analytics tools collect a wealth of data daily, transforming visitor interactions into actionable insights. This method tracks clicks, time spent on pages, and conversion rates.
- Platforms: Tools like Google Analytics or Adobe Analytics allow companies to monitor traffic flow to their websites. They offer a treasure trove of metrics, from user demographics to the path a user takes before making a purchase.
- Benefits: Real-time data tracking empowers businesses to adjust their content and marketing strategies swiftly, tailoring to audience needs. For instance, if analytics reveal that users abandon shopping carts at a particular stage, the business can investigate why and address those pain points.
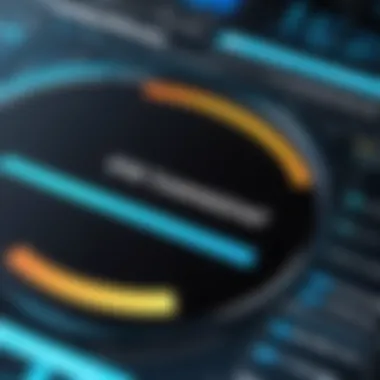
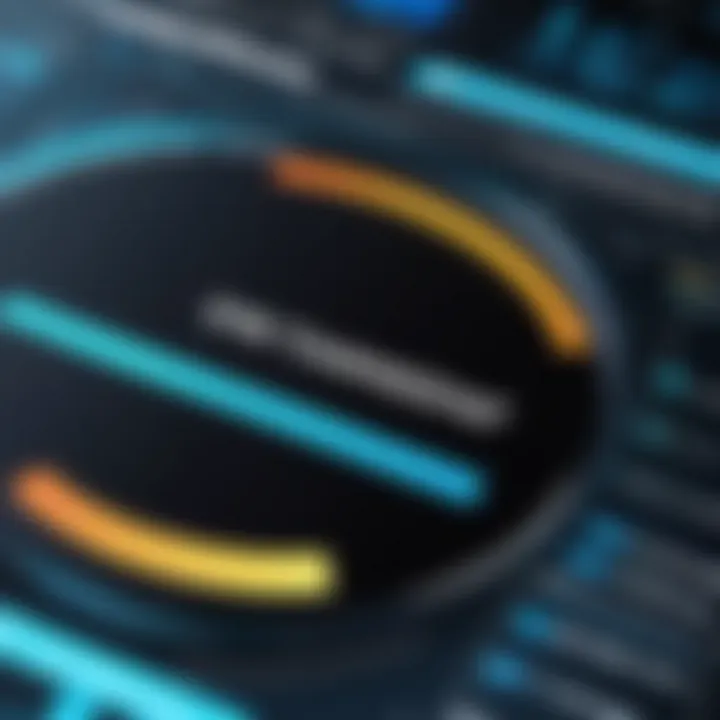
However, there are challenges too. Relying solely on quantitative metrics might overlook nuances in user experience that interviews or feedback can unveil. Here, employing a multi-faceted approach blends the quantitative analytics with qualitative feedback, leading to a more comprehensive understanding of user experience.
Discovering data is not just about sight; it's about vision. Wise decisions stem from a harmonious blend of figures and context.
Interpreting Data: Methodologies and Frameworks
Interpreting data effectively lies at the heart of transforming raw numbers into meaningful insights. Methodologies and frameworks utilized in data analysis not only determine how conclusions are drawn but also influence the overall impact of data on decision-making processes. Understanding these methodologies is especially crucial in today’s computational world, where stakes are high, and the dependence on accurate information is paramount. By embracing diverse methodologies, analysts can navigate through complex datasets, enabling them to extract actionable knowledge that informs strategic decisions across various sectors. The major benefits of a comprehensive approach to data interpretation include enhanced accuracy of insights, improved decision-making capabilities, and the ability to predict future trends based on historical data.
Statistical Methods for Analysis
Statistical methods serve as the backbone of data analysis, equipping analysts with the tools necessary for sound reasoning from data. In the realm of statistics, it’s essential to distinguish between descriptive and inferential statistics. Descriptive statistics summarize data sets, providing insights through measures such as mean, median, and mode as well as visual representations such as histograms and bar charts.
On the other hand, inferential statistics involve making predictions or generalizations about a larger population based on a sample. Techniques such as regression analysis and hypothesis testing fall into this category. For example, regression analysis reveals relationships between variables, allowing businesses to forecast sales trends based on historical marketing data. Additionally, hypothesis testing can help determine whether a marketing campaign had a statistically significant impact on customer engagement.
The ability to employ these methods accurately is crucial. Missteps in statistical interpretation can lead to misguided decisions, potentially jeopardizing outcomes. As a case in point, consider a company using biased sampling methods to gauge customer satisfaction. The results could reflect a skewed perspective, leading to poor strategic choices.
Data Visualization Techniques
Data visualization is more than just an aesthetic choice; it’s a fundamental component that enhances comprehension and fosters communication. Visual tools like dashboards, heat maps, and scatter plots allow intricate datasets to be distilled into digestible formats, which is particularly helpful when conveying information to stakeholders who may not have a technical background.
For instance, employing a heat map can illustrate customer interactions on a website, thereby guiding designers in optimizing user experience. By visualizing data trends, stakeholders can quickly identify patterns and outliers, which is oftentimes harder to detect within plain datasets.
Moreover, the principles of visual hierarchy play an important role in design. Elements such as color, size, and placement can emphasize key findings without overwhelming the audience with data overload. Effective data visualization essentially acts as a bridge between analysis and understanding, ensuring vital insights are communicated clearly and persuasively.
"Data visualization is like a coherent narrative, transforming complex stories into visual metaphors that anyone can appreciate."
Machine Learning in Data Analysis
Machine learning is revolutionizing the landscape of data analysis, bringing forth sophisticated methods to interpret and leverage data. Unlike traditional statistical approaches that often rely on human intervention, machine learning can derive insights autonomously by recognizing patterns within vast datasets. Techniques such as supervised and unsupervised learning enable systems to learn from data and make informed predictions or classifications without explicit programming for every scenario.
For example, supervised learning can be pivotal in predicting customer churn by analyzing previous behavior patterns, allowing businesses to implement preventative measures. Conversely, unsupervised learning techniques uncover hidden patterns within data, which can lead to new market segmentation strategies distinguishable from existing classifications.
Incorporating machine learning requires careful consideration of the data’s quality and quantity since biased or incomplete data can result in unreliable predictions. This potential for bias underscores the necessity for ethical guidelines in machine learning applications, emphasizing the importance of transparency in algorithmic decisions.
As technology advances, the fusion of machine learning with traditional methods promises to enhance our understanding of contemporary trends. By navigating through algorithms and data pipelines, analysts can unveil layers of implications, driving innovation that could influence future developments across industries.
The Role of Data in Technology
In an age where technology is at the core of every industry, the role of data cannot be overstated. Data serves as the backbone for innovations, steering the direction in which tech companies navigate. From influencing product design to shaping marketing strategies, understanding data's role is crucial for businesses aiming to stay ahead of the curve.
In many ways, data can be likened to a compass, guiding organizations through the complexity of modern markets. Its ability to offer insights into consumer behavior, operational efficiency, and market trends helps companies make informed decisions that ultimately drive success.
Data-Driven Decision Making in Tech Companies
The advent of data has changed the decision-making process within tech companies dramatically. Companies like Google and Amazon leverage vast amounts of data to refine their services and enhance user experiences. By utilizing analytics software, they can parse consumer data, identifying patterns and behaviors that inform everything from product development to customer service enhancements.
- Enhanced Customer Experience: Companies tailor their offerings based on user feedback and usage data to meet customer expectations better.
- Operational Efficiency: Data analysis helps optimize supply chains by predicting demand fluctuations, minimizing waste and costs.
- Innovation and Testing: By collecting data during product testing phases, companies can iterate quickly, adopting features that resonate with consumers—the mantra here being: “fail fast, learn faster.”
Despite numerous advantages, data-driven decision-making must navigate several pitfalls, including over-reliance on data without contextual understanding. Thus, human intuition still holds significance in the equation.
Emerging Technologies Impacting Data Analysis
As we look closer to the horizon, emerging technologies are reshaping the landscape of data analysis. Artificial Intelligence and Big Data analytics are leading the charge, presenting new benchmarks for how data can be interpreted and utilized.
The integration of AI enables companies to process massive datasets at lightning speed, delivering insights that were once impossible to uncover. For instance, machine learning algorithms learn from historical data to predict future patterns, revolutionizing industries like finance and healthcare. Furthermore, cloud computing has democratized access to advanced analytical capabilities, making it easier for smaller companies to harness the power of data without hefty infrastructure investments.
- AI and Machine Learning: Autom ate data classification, predictive analytics, and even offer personalized recommendations for users.
- Cloud Computing: Facilitates real-time data processing and storage, making it scalable for businesses of all sizes.
The convergence of these technologies signifies a shift towards predictive insights over reactive. Thus, organizations can not only envision future trends but actively prepare for them.
Case Studies: Successful Data Utilization
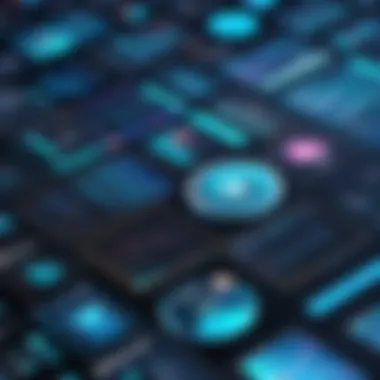
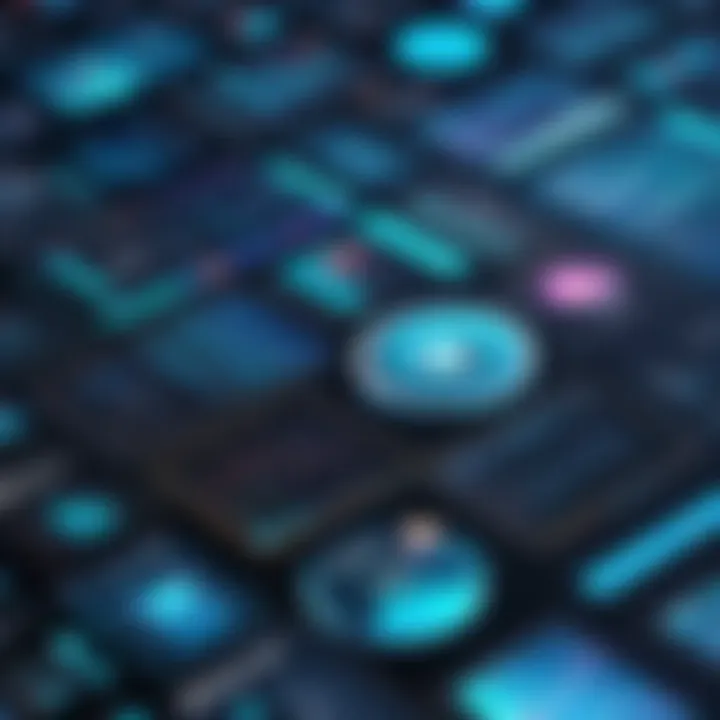
Examining real-world examples illustrates the tangible benefits of data utilization in technology.
- Netflix: They utilize viewing data and algorithms to not only recommend shows but also to decide which original content to produce. This user-centric approach has led to high engagement and subscriber retention.
- Spotify: The music streaming giant employs data analysis to curate personalized playlists based on user preferences, shaping how people discover music today.
- Tesla: Using data from their fleet, Tesla gathers insights that inform updates to software and improve vehicle performance, demonstrating how data can foster ongoing innovation.
These companies exemplify how strategic data utilization can pave the way for substantial business growth and customer satisfaction. Data is not just a resource; it becomes a competitive differentiator that can elevate a firm from being just a player in the market to a leader.
"In the world of technology, those who leverage data well often write the rules of the game."
Embracing data as a strategic asset is not just a trend but a necessity for companies desiring to innovate and thrive in the contemporary tech landscape.
Impacts of Data on Entertainment
Data plays a pivotal role in shaping the entertainment landscape today. With the rise of streaming platforms, social media, and digital content, data analysis becomes crucial in understanding audience preferences and content effectiveness. This section explores how data influences various facets of entertainment, from audience engagement to content creation strategies.
Audience Analysis and Content Creation
Understanding viewers' preferences is like holding a mirror up to society. Through sophisticated analytics, entertainment providers gather insights on what captures the attention of their audience. Demographics, viewing habits, and online behavior provide valuable clues.
- Demographic information helps in tailoring content to age, gender, and geographical location. For instance, a streaming service may realize that older viewers prefer classic movies while younger audiences lean towards modern thrillers. By analyzing these trends, producers can craft content that resonates more effectively with target demographics.
- Viewing habits are also telling. Many platforms track how long viewers engage with a specific genre or a particular series. Do they binge-watch or prefer weekly episodes? Such findings help creators understand the optimal number of episodes and the frequency of content release, ensuring audience retention over time.
- Online behavior is another key piece of the puzzle. Companies analyze social media interactions and online discussions to gauge audience sentiments. This feedback loop allows them to adjust their marketing strategies or even modify ongoing shows based on viewer reactions. For example, a show that captures a fandom’s imagination might see increased merchandise sales or even spin-offs.
"Data fuels creativity by bridging the gap between what creators want to produce and what audiences actually want to consume."
Streaming Services and Data Insights
Streaming services, such as Netflix and Hulu, rely heavily on data insights to maintain their competitive edge. These platforms utilize advanced algorithms to suggest content based on viewer habits, creating a personalized viewing experience. Think of it like a friendly concierge, always ready with the next great recommendation.
- Recommendation algorithms analyze millions of user interactions to suggest titles that viewers are most likely to enjoy. This not only enhances user experience but also drives longer viewing sessions, which is beneficial for platforms’ engagement metrics.
- Data analytics also extends to anticipating trends in content consumption. By keeping tabs on what’s popular or what is gaining traction on social platforms, services can greenlight new shows or movies that align with emerging viewer interests. A recent example includes the success of documentaries focused on social issues, which surged in popularity thanks to user demand insights.
- Conversely, data can also reveal when interest is waning. A drop in viewership can prompt the swift cancellation of a low-performing series, freeing resources for fresher content. This agile approach ensures that streams remain stocked with offerings that keep viewers tuned in.
In sum, the integration of data into entertainment profoundly reshapes how content is created and consumed. Through meticulous analysis, companies not only cater to immediate audience tastes but also predict future trends to stay ahead of the curve.
Data's Influence on Design
Data plays a pivotal role in shaping design today. With technological advancements and a deluge of information at our fingertips, designers are no longer relying solely on intuition. Instead, they harness data to make informed decisions about user experience and aesthetic choices. This synergy between data and design is transforming industries, ensuring that products not only look good but also resonate with users.
User Experience (UX) and Data Feedback
In the realm of design, user experience is the gold standard. It's not just about what looks pretty; it’s about how users interact with a product. Data feedback provides designers with invaluable insights into user behavior.
For example, consider a mobile app. By analyzing usage patterns through tools like Google Analytics, designers can grasp how users navigate the app, where they stumble, and what features are most engaging. This feedback loop allows for iterative design improvements, ensuring that each version of the app is better suited to user needs.
- Key Benefits:
- Informed Redesign: If surveys reveal that users struggle with a particular interface, that information drives design tweaks to improve usability.
- Feature Prioritization: Understanding what users love fosters developers to prioritize those features, ultimately enhancing user satisfaction.
- Predictive Improvements: Machine learning models analyze user data and anticipate future needs, making design proactive rather than reactive.
A fine example of this is Airbnb. They leverage extensive data from user interactions to guide their design decisions, optimizing everything from navigation to how listings are presented. As a result, they achieve a smoother user experience while maintaining a visually appealing platform.
Trends in Design Informed by Data
Trends in design increasingly reflect the insights gleaned from data analysis. Over the years, a clear shift has occurred: design is now very much a data-informed practice.
Several prominent trends have emerged as a direct consequence of data analysis:
- Personalization:
With data from user preferences and behavior, designs can be tailored to individual tastes. For instance, e-commerce websites utilize browsing history to recommend products, creating a custom shopping experience for users. - Responsive Design:
Designers are now utilizing data about devices used to access content. They adjust layouts for better viewing on mobiles versus desktop screens—making responsiveness a necessary aspect of modern design. - Minimalism:
Heavy analytics often show that users prefer clean, uncluttered interfaces. Therefore, many design trends now favor simplicity, steering away from overly complex layouts.
Designers who utilize tools like Figma or Sketch can integrate real-time data, ensuring ongoing relevance and appeal of their products. This hands-on approach means that design no longer exists in a vacuum but thrives in the vibrant interplay of data and design.
As we look ahead, the influence of data in design will likely only intensify, pushing boundaries and inspiring innovative solutions.
"Design is not just what it looks like and feels like. Design is how it works." — Steve Jobs
Ethical Considerations in Data Usage
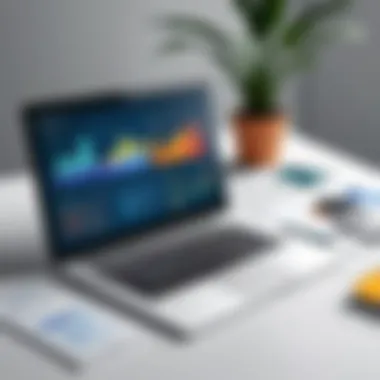
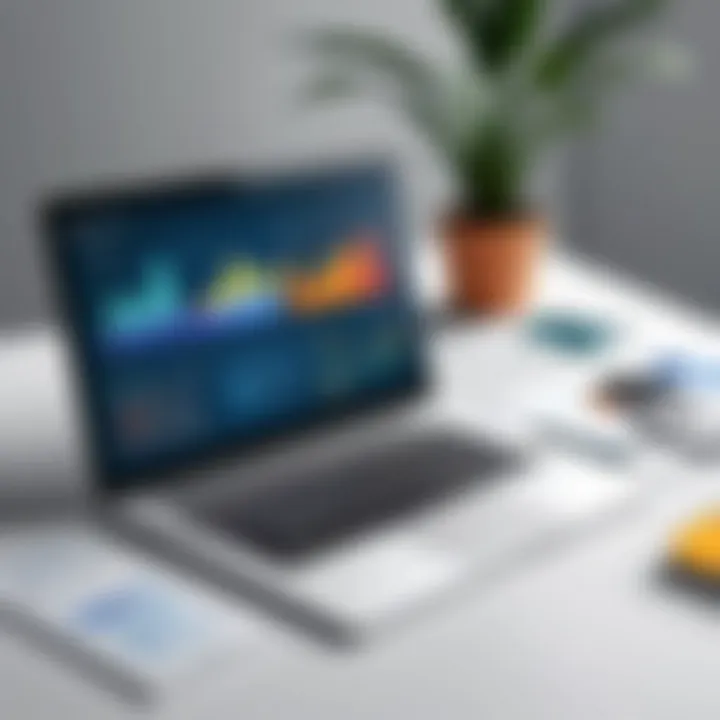
In the realm of data analysis, ethical considerations play a vital role. It's not simply about crunching numbers and drawing conclusions; it’s about understanding the implications of those actions on society. The principles of transparency, integrity, and accountability have never been more crucial.
Privacy Concerns and Data Security
When it comes to data, privacy is a cornerstone. In today’s data-driven world, individuals often trade their personal information for convenience. For instance, when signing up for news websites or social media platforms, users frequently provide their email addresses or phone numbers and potentially more sensitive data. But what happens to that data? With the multitude of breaches and leaks reported, privacy isn’t just a buzzword; it’s a substantial concern. Companies must ensure robust data security measures against unauthorized access. This ties into several key practices:
- Data Minimization: Only collect what’s necessary. When services gather excessive information, they not only increase vulnerabilities but also cross ethical boundaries.
- Informed Consent: Users need to understand how their data will be used. Transparent practices foster trust.
- Anonymization Techniques: Companies can use these methods to analyze data without compromising personal identities.
"Data privacy is not just a tech issue; it's a trust issue. Without trust, data loses its value."
Bias in Data Interpretation
Consider data interpretation, which often skews more towards the subjective than many care to admit. Every dataset reflects the biases of its collectors. For example, a survey on consumer preferences may overrepresent voices from urban areas while excluding rural perspectives. Such biases can distort the findings, leading to misinformed decisions that may have a far-reaching impact, from marketing strategies to user experience designs.
The role of bias in data analysis calls for critical reflection:
- Diverse Data Sources: Using varied sources can mitigate bias, ensuring more comprehensive analysis.
- Continuous Training: Data analysts need consistent education on recognizing their biases during interpretation.
- Employing Ethical Frameworks: Guidelines should be established to ensure that data analysis adheres to ethical standards.
Addressing these biases isn't just about accuracy; it’s about fairness. The potential fallout of ignoring these issues can lead to negative consequences affecting real lives. Thus, harmonizing ethical considerations with data usage is not just preferable—it's imperative.
Future Trends in Data Analysis
In the ever-evolving landscape of technology, keeping an eye on future trends in data analysis is crucial. It gives insight into how industries adapt and transform, paving the way for innovation and efficiency. This section digs into the exciting possibilities ahead, shedding light on integrating artificial intelligence and the changes in data sources. Such insights help stakeholders navigate the complexities of data in a digital era.
Integrating AI with Data Analysis
Artificial Intelligence has not just been a buzzword; it’s reshaping how data is processed and interpreted. This marriage of AI with data analysis brings forth capabilities that were merely dreams not long ago. Think of it as turbocharging the analytical process. With AI algorithms, businesses can sift through mountains of data almost instantaneously, uncovering trends and patterns that human analysts might overlook.
For example, in retail, AI can analyze purchasing behaviors, frequently used to predict future buying habits with astounding accuracy. Imagine an online store that suggests products based on customer preferences and trends recognized through vast data analysis. This is not a theoretical application anymore, but a reality seen in companies like Amazon, which employs sophisticated AI to enhance user experience.
Furthermore, AI-driven tools enable predictive analytics, providing companies a roadmap for future strategies. With machine learning models in play, they can learn from historical data and improve decision-making processes continuously. The implications are profound: more personalized services, optimized business models, and ultimately, enhanced customer satisfaction.
The Evolution of Data Sources
The sources from which we gather data have changed dramatically, and they continue to evolve. Gone are the days when data was solely collected through surveys or government reports. The rise of smart devices has transformed everyday life into a constant stream of data generation. Smartphones, wearables, and IoT devices are now essential contributors to data ecosystems.
Data can be categorized into various sources:
- Social Media: Platforms like Facebook and Twitter share quick, real-time insights on trends and sentiments.
- Mobile Applications: Apps gather user behavior data, assisting in nuanced user experience optimization.
- Public Sources: Government databases have also been digitized, allowing keen access to demographic information.
Each data source offers a unique advantage but also presents challenges. For instance, while social media data reflects real-time public sentiment, it can be noisy and biased. Understanding the nuances of these evolving sources is essential for accurate analysis.
Remember: The quality of analysis is only as good as the data used. Staying attuned to changing sources ensures insights remain valid and actionable.
As data sources proliferate, integrating these diverse datasets will be paramount for gaining holistic insights. Data analysts and businesses must stay agile, adapting their strategies to harness new sources effectively. Whether it’s refining customer journeys or enhancing operational effectiveness, the ability to leverage diverse data streams will be a game changer in upcoming years.
End: The Path Ahead
As we steer into an increasingly data-driven future, it becomes more crucial to distill the implications of our findings and adapt our approaches accordingly. This article's exploration of data analysis has depicted a tapestry woven with myriad trends impacting technology, entertainment, and design. Here, we synthesize the insights garnered, reinforcing the takeaways that inform our understanding and actions going forward.
Summarizing Key Insights
Throughout this article, we have illuminated several essential points:
- The Integral Role of Data: In every facet, from technological innovations to designing compelling user experiences, data underpins decision-making processes. Organizations tapping into data analytics can refine their strategies, enhancing engagement and effectiveness.
- Diverse Data Collection Methods: Understanding the merits of various collection techniques—be it quantitative surveys or qualitative interviews—helps in acquiring relevant insights tailored for specific needs.
- Ethical Considerations: The conversation surrounding data and ethics has never been more essential. Balancing data utilization with user privacy creates a dynamic tension that warrants constant vigilance and strategy adjustments.
- Evolving Technologies: With advancements like machine learning and AI playing increasingly pivotal roles, organizations must stay ahead of the curve, integrating these technologies into their frameworks for a competitive edge.
"Data is not just numbers; it's the bedrock of informed decision-making. The more we derive from it, the sharper our vision for the future."
Recognizing these insights offers a structured understanding of how data alters landscapes across sectors and equips us to face challenges head-on.
The Importance of Continued Analysis
Data analysis isn't a one-and-done deal. Instead, it necessitates ongoing scrutiny. Continuous analysis ensures adaptability amidst changing trends and user preferences. By regularly assessing data derived from consumer behaviors, organizations can pivot quickly, developing relevant and impactful strategies.
Some benefits of sustained analysis include:
- Enhanced Responsiveness: Organizations become more agile, able to adjust operations based on real-time data insights.
- Long-Term Patterns: Ongoing analysis unveils trends over time, granting deeper insights that can lead to informed forecasting and strategy refinement.
- Cross-Sector Interplay: With various industries increasingly influencing each other, understanding data across contexts can uncover innovative ideas and applications.