Exploring the Depths of Artificial Intelligence
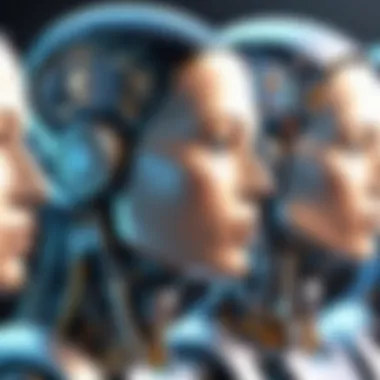
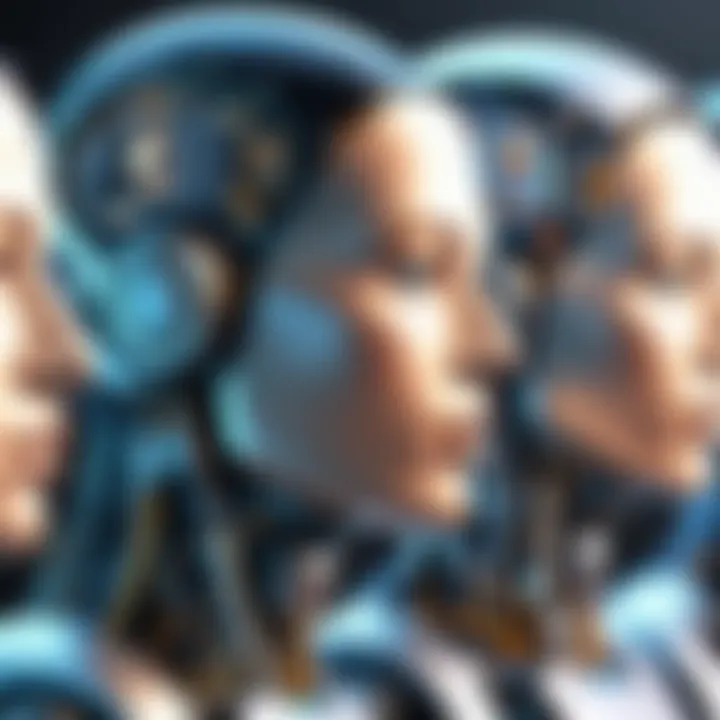
Intro
Artificial Intelligence, often abbreviated as AI, has evolved from a theoretical concept into a driving force shaping many aspects of our daily lives. In this exploration, we'll traverse the timeline of AI's journey, from its early conceptual days to its current applications that permeate various sectors including healthcare, finance, entertainment, and beyond. Each twist and turn illuminates the innovations that have propelled AI forward, and raises questions about its implications on society.
The recent surge in AI has been fueled by several technological advancements, such as improved algorithms, enhanced computing power, and the advent of massive datasets. As we delve deeper into this sprawling realm, it’s critical to dissect not just the technological advancements but also the underlying ethical debates, societal impacts, and future projections that accompany the proliferation of AI technologies.
By highlighting insights from industry experts and analyzing the latest trends, this article aims to paint a vivid picture of what AI has become while also posing important questions about where it's headed.
Let’s venture forth into the first sector, where we'll uncover the latest tech trends that characterize this dynamic and ever-evolving field.
Preface to Artificial Intelligence
Artificial Intelligence (AI) is not just a trend; it’s a revolution that is reshaping the very fabric of our society. The importance of delving into this first section is clear. As tech-savvy individuals, understanding AI’s fundamental aspects equips us to navigate a world increasingly influenced by algorithms and automated systems. The insights provided here serve as the bedrock for engaged discussions throughout the article.
AI stands at the intersection of computer science, mathematics, and cognitive psychology. Its definition encompasses a broad spectrum, from basic decision-making tasks to complex problem-solving abilities. Thus, grasping its scope allows us to appreciate both its potential and its limitations. This understanding opens the floor to critical questions about reliability, effectiveness, and ethical considerations.
Moreover, examining AI through a historical lens sheds light on its development and evolution, revealing how past challenges shape its current trajectory. The nuances of AI's journey teach us not only about the technology itself but also about our adaptability to change and innovation.
Definition and Scope
To begin, we need a solid definition of AI. At its core, Artificial Intelligence refers to systems or machines that mimic human cognitive functions such as learning and problem-solving. This definition encapsulates various technologies, from basic rule-based systems to advanced learning algorithms. More robust systems harness data to improve outcomes over time, which is vital in today's data-rich environments.
In exploring its scope, we encounter several categories, including narrow AI, designed for specific tasks like facial recognition or voice assistance, and general AI, which aims to handle any intellectual task a human can perform. Although we primarily see narrow AI today, the pursuit of general AI lingers at the edge of research discussions, creating a fruitful ground for exploration and speculation.
Historical Context
The Birth of AI
The inception of AI germinated in the mid-20th century, primarily through the works of pioneers like Alan Turing, who posed profound questions about machine intelligence. Turing’s concepts laid the groundwork for future developments, including the Turing Test, which evaluates a machine’s ability to exhibit intelligent behavior indistinguishable from that of a human. This early focus on emulating human thought paved the way for advances in machine learning and natural language understanding.
What’s crucial in this context is recognizing how the birth of AI shaped technological aspirations and set the stage for ambitious projects that followed. The key innovation was not merely creating machines but instilling them with autonomy and adaptability. Such ambitions, while offering promise, also brought significant challenges — balancing desired capabilities with ethical considerations.
Milestones in AI Development
Several groundbreaking moments punctuate AI's history, setting benchmarks that most current systems still strive to reach. In the 1950s, programs like the Logic Theorist demonstrated the ability to solve mathematical problems, proving that machines could indeed think — to a degree. The 1980s saw an influx of interest known as the first ‘AI boom,’ which culminated in expert systems that advised on everything from medical diagnoses to corporate strategy.
One key characteristic is the cyclical nature of its evolution. With each milestone, grounded expectations grew higher, often leading to disillusionment that could stall progress. Nevertheless, these milestones solidified foundational technologies that currently define AI's capabilities and applications.
The AI Winter Phenomenon
As the excitement surrounding AI reached its peak, it inevitably faced setbacks. This was most visible during the AI winters, periods marked by reduced funding and interest. The first AI winter in the late 1970s was triggered by unmet expectations, primarily due to the limitations of then-current technologies. During this time, researchers encountered the difficult reality that their systems had significant shortcomings in understanding complex human behaviors.
The key takeaway from the AI winter phenomenon lies in its stark lesson: progress isn’t always linear. Addressing challenges head-on became vital, allowing researchers to refine approaches and set realistic expectations. In hindsight, these periods of stagnation also offered opportunities for reflection and growth in the field.
As we move forward in this article, it’s important to consider all aspects explored here to form a solid foundation for deeper conversations regarding AI’s role today and in the future.
"Artificial Intelligence is the new electricity." — Andrew Ng
Through this historical immersion, we illuminate current discussions on AI technologies and their profound implications on various sectors, paving the way for a broader examination of its core technologies and industrial applications.
Core Technologies Behind AI
The backbone of artificial intelligence (AI) rests on a robust foundation of core technologies. These elements not only enhance the capabilities of AI systems but also determine their effectiveness across various applications. By grasping these technologies, readers can appreciate their significance in the broader landscape of AI development.
Machine learning, deep learning, and natural language processing are pivotal in driving innovations and efficiencies in countless industries. They serve distinct purposes, yet they interconnect seamlessly, much like the gears in a clock, ensuring that the entire mechanism runs smoothly. Each of these core technologies contributes unique features and benefits that align with the ultimate goal of mimicking human cognitive abilities—improving our interaction with machines, optimizing operations, and creating unprecedented efficiencies.
But it's not all smooth sailing. As much as these technologies offer astonishing potential, there are challenges too. Issues related to data quality, algorithm robustness, and the ethical implications of automation come into play, prompting discussions that can't be overlooked in the journey of AI advancement. Let's dive into the details of these technologies.
Machine Learning Fundamentals
Supervised Learning
Supervised learning stands out in the realm of machine learning due to its structured approach. It involves training models on labeled data, meaning the algorithm learns to make predictions or classifications based on input-output pairs. This characteristic gives it a leg up when it comes to tasks requiring accuracy, like medical diagnosis or credit scoring. What's noteworthy here is that supervised learning relies heavily on vast amounts of high-quality data, which can be both a strength and a weakness.
The advantage is its ability to provide precise outcomes by identifying patterns in historical data. However, its unique feature—dependency on labeled examples—can be limiting, especially in scenarios where such data isn't readily available or expensive to procure. In this context, supervised learning becomes a popular choice in situations where transparency and interpretability of outcomes are paramount.
Unsupervised Learning
Unsupervised learning takes a different tack. Instead of relying on labeled data, it works with input data that has no explicit outputs. The model's goal is to identify underlying patterns through clustering or association. This approach shines in exploratory data analysis, even revealing hidden structures that human analysts might overlook.
The beauty of unsupervised learning lies in its ability to work with vast amounts of unrefined data, making it invaluable in areas like customer segmentation or anomaly detection. However, a notable downside is the potential for misinterpretation of results, since the algorithm might highlight spurious correlations. This unpredictability can lead to decisions based on misleading data patterns, an important consideration for any practitioner.
Reinforcement Learning
Reinforcement learning adds an adaptive layer to machine learning. Here, an agent learns to make decisions through trial and error, receiving rewards or penalties based on its actions. The key characteristic of this approach is its incorporation of feedback loops, resulting in continuous improvement over time. It's particularly beneficial in dynamic environments, such as game playing or robotics, where strategies evolve based on past experiences.
The unique aspect of reinforcement learning is its ability to operate in environments without predetermined outcomes, but this can also lead to challenges. For the agent, the path to optimal behavior may be fraught with exploration versus exploitation dilemmas, where the agent must balance between trying new strategies and utilizing known effective strategies.
Deep Learning Explained
Neural Networks Overview
Neural networks are the bread and butter of deep learning, offering a layered structure that mimics human brain connectivity. These networks consist of nodes (or neurons) organized into layers, enabling them to process information similarly to how humans do. They excel in handling complex tasks, such as image and speech recognition, making them an attractive option for developers.
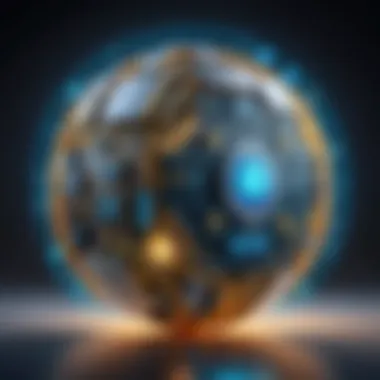
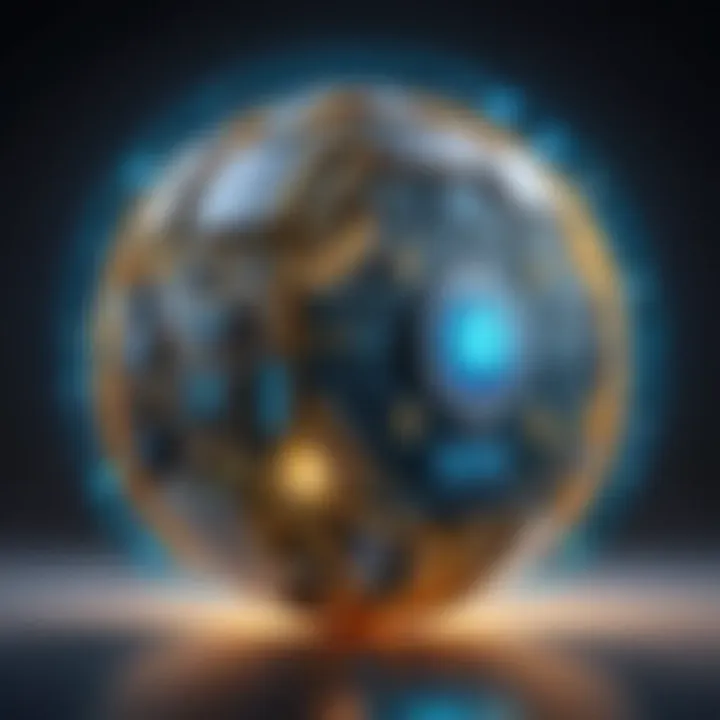
What sets neural networks apart is their ability to learn hierarchical representations of data. This means they can automatically learn features from raw data without precise manual feature extraction—a remarkable shift from traditional models. Yet, their complexity can also be a double-edged sword; training these networks requires significant computing power and can lead to challenges in interpretability, especially in critical applications like healthcare.
Applications of Deep Learning
The applications of deep learning are immense and varied. From enhancing virtual assistants to revolutionizing autonomous vehicles, deep learning taps into diverse domains. For instance, in healthcare, deep learning algorithms can analyze medical images with astounding accuracy, supporting physicians in diagnoses more efficiently.
This benefit hinges on the capacity of deep learning models to excel at pattern recognition across large datasets. However, the reliance on data can amplify challenges, including the risk of overfitting, where a model performs well on training data but poorly on unseen data. Such pitfalls can impede real-world effectiveness and underscore the necessity for rigorous validation practices.
Challenges in Deep Learning
As advanced as deep learning is, it’s not without its hurdles. The sheer amount of data it needs can be burdensome, especially when considering privacy concerns in data collection. Moreover, interpretability remains a significant challenge; deep learning models often act like black boxes, producing outputs without explaining how they arrived at those conclusions. This lack of transparency makes it harder for stakeholders to trust AI systems in sensitive scenarios, such as legal or medical contexts.
Understanding these challenges is vital. They necessitate a balanced approach towards employing deep learning, focusing not only on the power of the models but also on considerations for ethical, legal, and social implications.
Natural Language Processing
Understanding Human Language
Natural language processing (NLP) stands at the intersection of linguistics and computer science, aiming to enable machines to understand and interpret human language in a way that is both meaningful and useful. One of its main contributions to AI is its ability to bridge communication gaps between humans and machines. By enabling machines to comprehend text, speech, and contextual clues, NLP enhances user experiences across countless applications.
What’s crucial here is the development of algorithms that work with the nuances of language—like idioms, slang, and emotional tones. This makes NLP a popular area of exploration as developers seek to create tools that resonate with real-world human interactions.
Applications in Text and Speech
The realm of NLP has seen significant advancements in applications such as translation services, sentiment analysis, and chatbots. These technologies have burgeoned into everyday use, enabling organizations to analyze consumer feedback, automate customer service inquiries, and even assist in language learning.
A standout characteristic of these applications is their adaptability across diverse fields. Yet, the unique feature of NLP applications lies in their dependency on the continuous development of language models, which can sometimes struggle with dialect variations, leading to inaccuracies. Thus, while automation and efficiency are undeniable benefits, practitioners must remain observant of these limitations.
Current Limitations
Despite its growth, NLP faces considerable hurdles. The primary challenge centers around understanding context and semantics, particularly in idiomatic expressions and cultural references. While models have improved, they can still falter when discerning a subtle joke or sarcasm, often resulting in a misinterpretation of intent.
Moreover, issues such as biased training data can perpetuate stereotypes or misrepresentational outcomes. Addressing these limitations isn't just beneficial; it's essential for ensuring that NLP applications serve all users equitably.
In summary, while core technologies in AI, including machine learning, deep learning, and natural language processing, offer exceptional opportunities, recognizing their inherent challenges and limitations is equally crucial. This approach allows for more nuanced discussions around their implementation and fosters responsible advancements in the field.
AI in Various Industries
Artificial Intelligence has become a cornerstone in various sectors, reshaping how businesses operate and deliver services. The role of AI is not merely supplementary; it's transformative. From healthcare innovations that save lives to financial algorithms that guide investment strategies, AI is embedded in numerous processes. The benefits range from enhanced efficiency to improved accuracy and decision-making capabilities. However, the integration of AI comes with its own set of considerations, calling for an in-depth examination of its impact across different industries.
Healthcare Innovations
Diagnostic Tools
Diagnostic tools powered by AI are revolutionizing the medical field. These tools utilize data and algorithms to identify diseases at early stages, enhancing patient outcomes. The key characteristic here is their ability to analyze vast datasets quickly, often outperforming human capabilities in accuracy. For instance, AI tools can process images from MRIs or X-rays and detect anomalies that a radiologist might overlook.
A significant benefit of these diagnostic tools is their consistency. Unlike human practitioners who might experience fatigue, an AI system remains equally precise irrespective of workload. However, a downside lies in the potential overreliance on technology, possibly delegating critical decisions to machines without sufficient oversight.
Personalized Medicine
Personalized medicine is an emerging area where AI plays a pivotal role. Its focus is to tailor treatment plans based on individual patient data, thus increasing effectiveness. Here, the power of AI is harnessed to analyze genetic information, lifestyle, and environmental factors to determine the best course of action for a patient.
The standout feature of personalized medicine is its adaptability; treatments can evolve based on the patient's response. While this approach promises a higher success rate, it raises concerns about data privacy, as extensive personal information is required. Handling this sensitive data responsibly is crucial in maintaining trust between patients and healthcare providers.
AI in Research and Development
AI's involvement in research and development presents an intriguing shift. It accelerates the discovery of new drugs and treatments, streamlining processes once deemed time-consuming. By simulating potential drug interactions or disease pathways, AI can predict outcomes much faster than traditional methods.
The key advantage of using AI in R&D is its ability to sift through and analyze enormous amounts of data. However, this also means there's an increased risk of data inaccuracies leading to skewed results. Maintaining robust checks and balances becomes essential to mitigate such risks.
Finance and AI
Algorithmic Trading
Algorithmic trading represents a significant advancement in financial markets, with algorithms performing trades at lightning speed. These systems analyze market trends to execute buy and sell orders, often in milliseconds. The major advantage is efficiency—these algorithms can process information far quicker than human traders, maximizing potential gains.
Despite the upsides, algorithmic trading can lead to market volatility. Automated decisions based on flawed data or sudden market shifts could spiral into a financial crisis, making it critical to set safeguards against such scenarios.
Fraud Detection
Fraud detection is another area where AI shines brightly. AI systems can scrutinize transactions in real-time, flagging unusual patterns that may indicate fraudulent activity. This capability is paramount for safeguarding against losses in the financial sector.
The unique selling point of AI in fraud detection is its learning ability. Over time, these systems refine their algorithms based on historical data, becoming increasingly adept at identifying fraud. On the flip side, the reliance on algorithms may overlook human insight, which could also be essential in complex decisions.
Risk Management
In the context of risk management, AI helps companies forecast risk levels by analyzing various economic factors, guiding decisions on investments and operational strategies. The standout characteristic of AI risk management tools is their predictive analytics capability, providing organizations with a clearer picture of potential pitfalls.
However, while AI can enhance risk assessment, it's crucial to integrate human judgment, particularly in high-stakes scenarios. Excessive trust in automation may lead to strategic errors, particularly in uncertain markets.
Transforming Manufacturing
Automation and Robotics
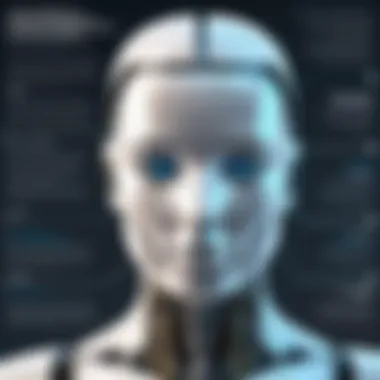
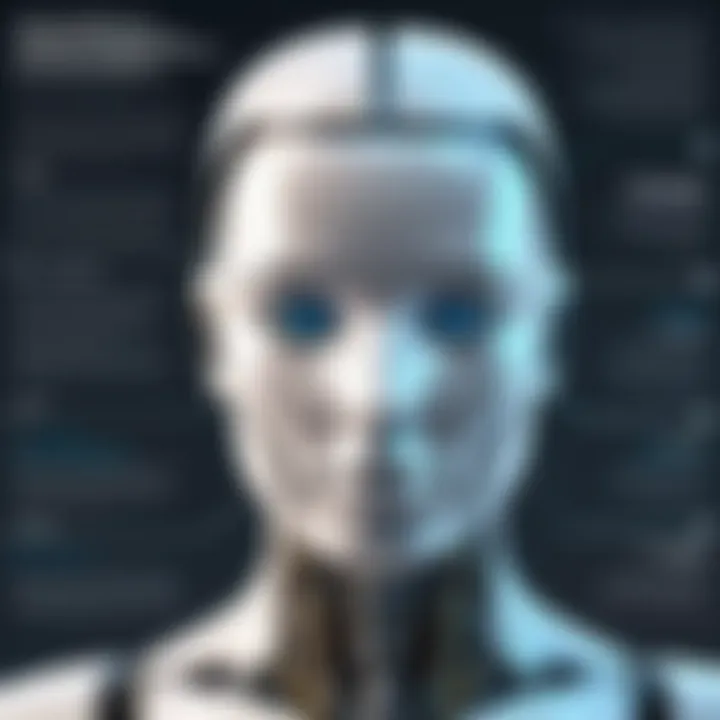
The introduction of automation and robotics into manufacturing has not only improved productivity but also optimized resource management. These AI-driven machines can handle repetitive tasks without error, freeing human workers for complex problem-solving and oversight roles.
One of the key traits of automation is its scalability; companies can adjust robotic operations to match production requirements swiftly. However, the initial investment in such technologies can be substantial, and the transition can disrupt worker dynamics, necessitating adjustment periods.
Supply Chain Optimization
AI aids in supply chain optimization, where it analyzes data to forecast demand and improve supply management. This application can drastically reduce waste and enhance efficiency across the board.
The major benefit of implementing AI here is its ability to respond to real-time data, which helps firms react swiftly to market changes. On the downside, overdependence on AI for logistics might make companies vulnerable to systemic failures if the technology encounters glitches.
Predictive Maintenance
Predictive maintenance utilizes AI to forecast equipment failures before they occur. By analyzing operational data, companies can schedule maintenance activities only when necessary, essentially sidestepping unexpected breakdowns.
This approach reduces downtime, leading to significant cost savings. Meanwhile, the flip side of this technology is that inaccuracies in data interpretation can result in unnecessary maintenance or, conversely, crucial missed repairs.
AI in Entertainment
Content Creation Algorithms
AI's role in content creation algorithms is reshaping how media is produced. These algorithms can generate articles, videos, and even music, all tailored to target audiences.
The main advantage is efficiency; content can be generated at scale, reducing costs and time. However, a significant concern is originality; there are questions about the creative merit of AI-generated content, which can sometimes lack the nuance of human emotion.
Recommendation Systems
Recommendation systems, like those used by platforms such as Netflix or Spotify, employ AI to analyze user preferences and suggest personalized content accordingly.
Notably, this feature enhances user experience by making recommendations that keep viewers engaged. Conversely, it also poses a challenge of creating echo chambers, where users only receive suggestions that align with their existing preferences, limiting exposure to diverse content.
Gaming Innovations
AI has paved new roads in gaming, introducing smarter characters and more engaging narratives. Interestingly, the implementation of AI allows for dynamic storylines that adapt based on a player’s decisions.
This adaptability is a key feature of modern gaming, making experiences immersive. However, a potential downside could be the increased complexity that new players might face, potentially alienating casual gamers.
Ethical Considerations
In the ever-evolving landscape of artificial intelligence, the ethical implications stand as a salient concern. As AI technologies become woven into the fabric of our society, it's crucial to examine the moral ramifications and implications of their deployment. Engaging with ethical considerations will help ensure that AI serves humanity positively and contributes to the greater good.
Bias in AI Systems
Sources of Bias
One of the foremost issues in AI today revolves around bias. Bias can seep into AI systems from various sources, including the data used during the training phase. The very nature of data itself can reflect human prejudices, which can perpetuate discrimination in the outcomes that AI produces. For instance, if a dataset predominantly features certain demographics, the AI may become proficient at recognizing and serving those traits, while neglecting others.
This selection of biased datasets highlights an essential trait: the importance of scrutinizing data origins and how it’s compiled. It is beneficial for creating a more balanced and inclusive AI, yet it poses challenges, as not all perspectives may be adequately represented. Thus, ensuring diversity in the data resources becomes a critical avenue for solving the bias problem.
Impact on Decision Making
The impact of biases in AI systems extends into everyday decision-making processes, influencing industries from hiring practices to criminal justice. Take, for example, AI tools used in hiring. If a system is trained primarily on data from a particular demographic, it might favor similar candidates, thus perpetuating a cycle of exclusion.
Understanding this key characteristic of AI bias is paramount. A well-informed choice can drastically reshape the landscape of hiring and lending practices. It's crucial to bear in mind that biased AI can lead to misjudgments with serious real-world consequences, making it essential to invest time and resources in assessing the algorithms and models at play.
Strategies to Mitigate Bias
Recognizing bias is only half the battle; companies and developers must implement strategies to mitigate bias to foster fairer AI systems. Approaches such as adopting diverse datasets, regularly auditing algorithms, and involving interdisciplinary teams can lead to more reliable outcomes. The unique advantage of these strategies lies in their potential for creating a more ethical AI practice, moving towards inclusiveness in technology.
However, there are disadvantages; such strategies often require dedicated resources and financial investment. Hence, the challenge remains balancing the pursuit of ethical standards while addressing immediate business pressures.
Privacy and Surveillance
Data Collection Practices
In today's digital age, collecting data has become a cornerstone of AI. How data is harvested plays a critical role in developing AI technologies. Transparent data collection practices are essential for ensuring that users are informed about what data is being collected and how it is being used.
A notable feature here is the extent to which organizations can collect and analyze user data. While beneficial for enhancing service personalization, it can also lead to ethical dilemmas regarding informed consent. Striking a balance between effectively utilizing data and respecting user feelings makes this an important topic for our understanding of AI ethics.
Implications for Personal Privacy
The implications for personal privacy stemming from data collection practices are nothing short of substantial. As AI systems increasingly rely on vast amounts of personal data, concerns about surveillance grow. The key characteristic of these implications is rooted in the tension between convenience and privacy.
While many AI-driven applications offer unparalleled ease of use, they often do so at the expense of user privacy, stirring distrust. The unique feature here is the realization that convenience may overshadows ethical considerations, thus it's pivotal to discuss and develop frameworks that safeguard individuals’ privacy rights without hampering technological advancements.
Regulatory Frameworks
To navigate this intricate landscape, regulatory frameworks are essential. These legal structures aim to provide guidelines for ethical data usage and protect user information better. Robust frameworks can foster transparency in data practices, ensuring respect for individual privacy while maintaining trust in AI technologies.
However, the challenge lies in developing regulations that keep pace with rapid technological progress. A potential disadvantage might be the stifling effect overly rigid regulations could have on innovative advancements within the field.
AI and Job Displacement
Skills Gap and Workforce Adaptation
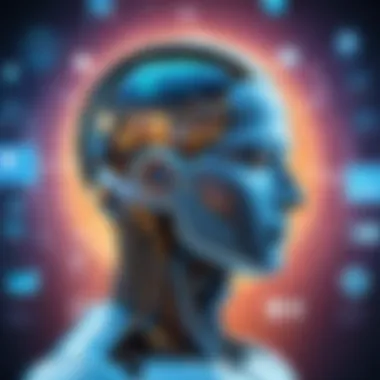
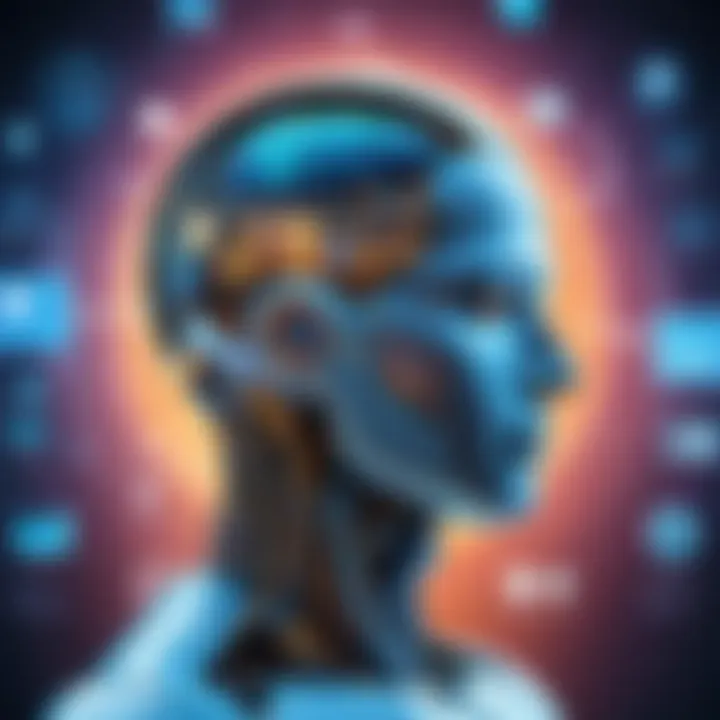
Artificial intelligence is reshaping industries, but it also brings challenges like job displacement. The skills gap is a significant aspect of this transition, highlighting the mismatch between existing workforce skills and the requirements for new jobs created by AI. The lack of appropriate training programs can lead to widespread unemployment.
Recognizing this distinctive characteristic is important. Before adopting AI technologies, businesses must consider investing in employee training and upskilling initiatives to prepare their workforce for changing roles.
Future of Work
AI doesn’t just threaten existing jobs; it reshapes the very concept of work itself. Emerging roles require adaptability and a tech-savvy mindset. The future of work reflects the need for a workforce that is flexible and willing to learn continuously.
This evolving landscape poses both challenges and opportunities; businesses that can pivot promptly may find they have a competitive advantage over stagnant competitors.
Policy Recommendations
To address these challenges proactively, policymakers must craft regulations that protect workers while facilitating technological growth. These policies could support upskilling initiatives, provide social safety nets, and stimulate job creation in emerging sectors.
Innovative policy frameworks can serve as robust solutions that reconcile technology's rapid advancement with society's need for stable employment. The unique feature of this approach is that it addresses the potential economic disruption through foresight and planning, rather than reaction.
The Future of Artificial Intelligence
The future of artificial intelligence (AI) is a topic that deserves careful examination, as it holds significant implications for numerous aspects of our daily lives, economies, and global stability. As technologies evolve, understanding these future trends and challenges becomes more crucial. In essence, the interplay of emerging technologies and ethical considerations shapes AI's journey ahead.
Emerging Trends
AI and Quantum Computing
AI and quantum computing represent a merging of extraordinary technologies that promise to push the boundaries of what is possible. At its core, quantum computing uses the principles of quantum mechanics to tackle complex problems at speeds beyond current supercomputers. This offers a potent opportunity for AI, enabling it to process vast data sets and discover patterns or insights previously out of reach. The key characteristic of quantum computing is its ability to explore multiple possibilities simultaneously, a feature that can be harnessed to improve machine learning algorithms significantly.
However, that is not without its challenges. The practical application of quantum computing remains in its infancy, with substantial investment required for research and development. Organizations like IBM and Google are working diligently to make universal quantum computers a reality. Yet, as these technologies develop, the balance between potential benefits and uncertainties regarding performance and accessibility will invariably influence AI’s integration into various domains.
Augmented Reality and AI
Augmented reality (AR) in tandem with AI offers artistic possibilities, providing immersive experiences that blend digital content with the physical world. This trend is witnessing rapid adoption, particularly in sectors like retail and education. AR enables users to interact with unfamiliar environments in engaging and informative ways, enhancing understanding and retention.
One unique aspect of AR combined with AI is its ability to analyze user interactions and adapt the experience in real-time. For instance, a clothing retailer might integrate AR technology, allowing customers to virtually try on outfits. This characteristic not only boosts sales but enriches customer experiences, making shopping efficient and enjoyable. Therefore, merchants can offer personal recommendations based on individual preferences, which further ties customer engagement to increased revenue.
Nevertheless, as AR expands, concerns regarding data privacy and user experiences must be rigorously addressed. The balance between delivering innovative solutions and ensuring user trust remains delicate.
Ethical AI Developments
Ethical AI development has emerged as a central focus in discussions about the future of artificial intelligence. The fast-paced growth of AI technologies leads to important questions regarding accountability, bias, and transparency. The key aspect of ethical AI is ensuring algorithms are designed to be fair and equitable, minimizing unintended biases inherent in data.
Organizations are increasingly aware that trust in AI systems can catalyze adoption rates significantly. For example, initiatives that emphasize fairness tackle concerns about algorithmic discrimination, protecting vulnerable populations. More industries are assessing the impact of their AI implementations, fostering greater responsibility amongst developers to produce systems that prioritize user welfare.
However, forming a consensus on ethical standards presents hurdles. Policymakers and technology leaders may have divergent views about what constitutes ethical development. This fragmentation can delay meaningful advancements, placing the responsibility squarely on innovators to advocate best practices in AI.
Global Impact
AI in Developing Economies
In emerging markets, AI can be a cornerstone for innovation and growth, providing opportunities for economic advancement in ways rarely seen in history. The ability to automate routine tasks enhances productivity, reshaping industries and creating new job prospects.
A noteworthy feature of AI in developing economies is the potential for leapfrogging traditional industrial growth. For instance, mobile banking technologies have gained traction in regions lacking established banking systems, offering financial services to millions who were previously unbanked. The integration of AI could improve these services by personalizing offerings and optimizing financial management.
Nevertheless, these benefits come with caveats; access to technology and education remains uneven, risking deepening existing inequalities. Policymakers must ensure equitable access and empower the workforce to adapt.
AI, Climate Change, and Sustainability
AI holds promise as a vital player in the fight against climate change and promoting sustainable practices. Its analytical prowess helps optimize energy consumption in various sectors such as manufacturing and transportation. Furthermore, AI systems are capable of predicting weather patterns, aiding in disaster preparedness, and improving resource management.
The unique characteristic of AI’s application to sustainability lies in its capability to analyze massive datasets. For example, using AI-driven predictive analytics can result in reduced carbon emissions through better route planning for shipping and logistics.
However, there are hurdles to tackle. The carbon footprint of data centers and the energy consumption associated with large AI models must also be considered when discussing the technology’s sustainability prospects. Balancing innovations with environmental responsibilities presents a complex challenge.
The Role of AI in Global Security
Artificial intelligence is increasingly vital in global security, offering tools to address complex security challenges faced by nations worldwide. AI's ability to process and analyze information at unparalleled speeds is reshaping the defense landscape.
The essential feature of AI in security is its application to real-time threat assessment and response. For instance, AI-powered surveillance systems can detect anomalies in public spaces, triggering alerts for potential threats much quicker than human oversight could. This capability is a powerful asset for law enforcement and defense agencies alike.
However, the rapid adoption of AI in security also poses dilemmas. The potential for misuse, such as intrusive surveillance and erosion of privacy rights, raises alarms. Hence, global consensus on ethical guidelines for AI use in security must be prioritized to safeguard democratic values.
In essence, the future of artificial intelligence is poised at the intersection of innovation and ethics, demanding a coherent response from all stakeholders to truly unlock its potential.
Ending
To round off our exploration into artificial intelligence, it’s crucial to underscore its potential impact on our world. As we've traversed through the various landscapes of AI, from foundational technologies to ethical implications, one thing stands clear: AI is not just a tool – it's becoming a core component of our societal fabric.
Recap of Key Insights
In essence, we have ventured through key aspects including:
- Historical Development: Understanding how AI has evolved from simple machines to complex algorithms that can now simulate human cognition.
- Core Technologies: Delving into machine learning, deep learning, and natural language processing; these are not merely buzzwords but the engines driving today’s innovation.
- Industry Impact: Observing how sectors like healthcare, finance, and manufacturing are transforming under AI's influence, revealing both opportunities and challenges.
- Ethics: Acknowledging biases inherent in AI systems, the serious implications for privacy and the uncertain future of jobs in the wake of automation is essential for shaping any future discourse on AI.
- Future Directions: As we discussed emerging trends and the global impact of AI, it’s evident that these transformations require careful consideration and proactive governance.
Final Thoughts
Reflecting on the intricacies we've discussed, it’s apparent that the future of artificial intelligence is ripe with promise yet fraught with challenges. For tech-savvy individuals and early adopters, staying abreast of these developments is crucial. As competition heats up across industries, understanding AI's capabilities will prove beneficial to not just leverage it effectively, but to navigate its complexities responsibly.
Ultimately, a thoughtful approach to AI can lead to solutions that enhance human well-being while addressing the ethical considerations that accompany this powerful technology. It is imperative that as we continue the dialogue around AI, each individual, technologist, and policymaker reflects on their role in shaping this dynamic landscape.
"AI is a relentless tide that is reshaping our shores. Those who understand its currents will find fortune; those who do not, may risk being swept away."
In sum, the exploration of artificial intelligence is ongoing. As we stand on the cusp of yet more breakthroughs, the responsibility lies with us to ensure that these developments are both progressive and humane.