The Impending AI Takeover: Implications and Insights
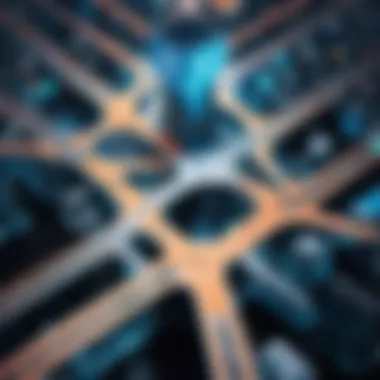
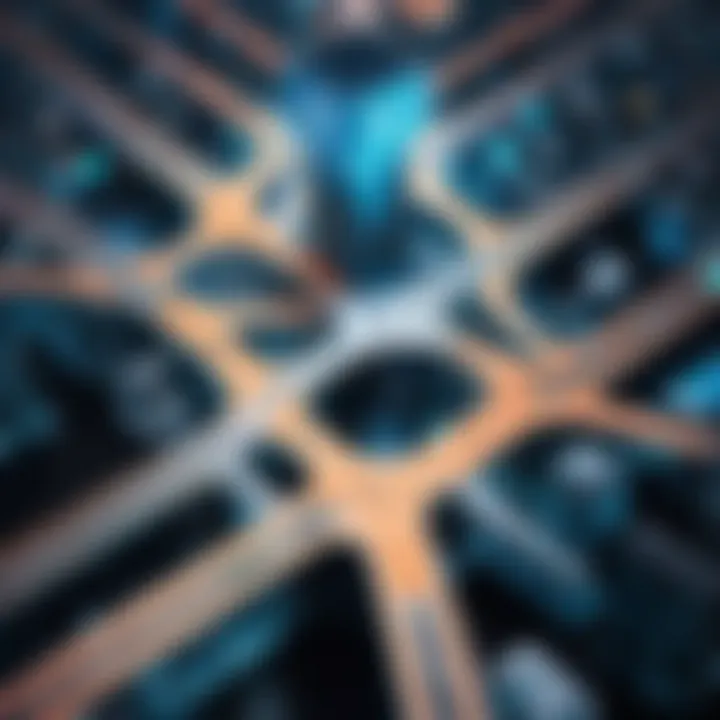
Intro
The recent dialogues surrounding artificial intelligence (AI) evoke a sense of urgency. They underline critical shifts that speak to the future of human existence. In a world rapidly embracing AI, we are confronted with numerous implications and challenges. From economic reshaping to ethical considerations, the spectrum of AIβs reach is expansive.
This article seeks to navigate the intricate pathways of AI advancements. It will address the transformative effects on various sectors while exploring the ethical dilemmas that accompany these technologies. As society grapples with the impending changes, understanding the implications of AI becomes paramount.
Technology Insights
As we streamline into the realm of AI technology, identifying the latest trends is essential. Here, we dissect groundbreaking developments and innovations that will pave the way for what's next.
Latest Tech Trends
AI integration is redefining typical workflows. Companies utilize AI to automate processes and optimize systems. For instance, in fields like healthcare, AI assists in diagnosing conditions with remarkable accuracy. This technology is not only enhancing productivity but also reshaping how services are delivered.
Innovation in Tech
Constant innovation underlies the evolution of AI. Major tech companies, including Google and Microsoft, are investing heavily in deep learning algorithms. These advancements enable machines to learn from data patterns, improving decision-making processes across diverse industries. Furthermore, AI-driven tools such as chatbots are transforming customer service experiences.
"AI has moved from theoretical potential to practical application across many sectors, opening doors to efficiency previously thought unattainable."
Ethical Considerations
With the acceleration of AI technology, ethical conundrums arise. Questions about data privacy, algorithmic bias, and job displacement are at the forefront. Stakeholders must come together to create frameworks that guide the responsible implementation of AI.
Industry Spotlights
Focusing on various industries unveils how deeply AI is interwoven into their fabric. Tech experts provide insights into the current landscape, helping demystify the role of AI in different sectors.
Interviews with Tech Experts
Insights from professionals in the field shed light on the practicalities of AI. Conversations with AI developers and researchers can offer a ground-level view of challenges and triumphs. These experts underscore the necessity of interdisciplinary collaboration to navigate AI's complexities.
Behind-the-Scenes in Technology
Understanding the mechanisms of AI development is crucial. An insiderβs perspective reveals how algorithms are created, tested, and deployed. Documenting these processes contributes to knowledge about AIβs capabilities and limitations.
The End
As we press on toward an AI-determined future, the implications remain formidable. The ethical, economic, and societal challenges must not be overlooked. To foster a sustainable relationship with AI, a conscious effort guided by informed decisions is vital.
Understanding Artificial Intelligence
Understanding Artificial Intelligence is crucial for grasping its implications in our ever-evolving world. As AI systems permeate various sectors, it is important to comprehend what AI truly entails. This section sets the foundation for discussion on AI's capabilities and its societal impact. Knowing the definition, history, and types of AI allows one to evaluate the nuances of this technology effectively. This understanding leads to better-informed discussions on the challenges and ethical considerations that accompany AI's integration into daily life.
Definition and Scope
Artificial Intelligence, often abbreviated as AI, refers to the simulation of human intelligence processes by machines, especially computer systems. These processes include learning, reasoning, perception, and problem-solving. The scope of AI is vast, encompassing algorithms, data processing, and applications across different domains. Understanding AI's definition and scope enables individuals to recognize its potential, limitations, and areas of application. AI's rapid advancements are reshaping industries, leading to a need for a comprehensive grasp of these developments.
Historical Context
AI is not a novel concept; its roots can be traced back to the mid-20th century. Pioneers like Alan Turing explored the potential for machines to exhibit intelligent behavior. Over the decades, AI has evolved through various stages, from symbolic systems to the emergence of machine learning. Each phase reflects technological advancements and a growing understanding of computational capabilities. Examining this historical trajectory offers insights into where AI stands today and its potential future direction.
Types of AI
AI can be classified into different types based on its intelligence capabilities. It is paramount to understand these classifications to appreciate the diverse applications of AI.
Machine Learning
Machine Learning is a subset of AI focused on the idea that systems can learn from data. It enables computers to improve their performance on specific tasks without being explicitly programmed. A key characteristic of Machine Learning is its ability to adapt and provide insights based on patterns found in data. This makes it a popular choice as organizations strive for data-driven decision-making.
The adaptability of Machine Learning systems ensures that they evolve over time, improving accuracy and operation efficiency.
However, one must consider the challenges as well. Machine Learning often requires large data sets and can be prone to biases if not well managed.
Deep Learning
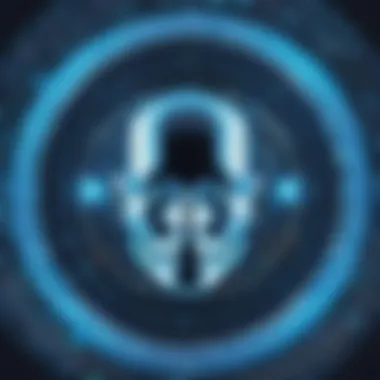
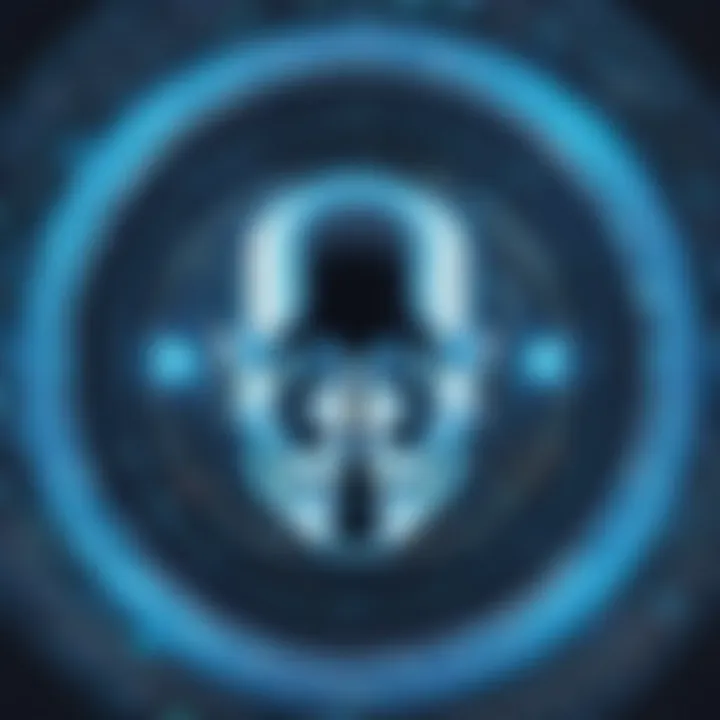
Deep Learning is a more advanced form of Machine Learning that uses neural networks to model complex relationships in data. A defining characteristic of Deep Learning is its capability to process vast amounts of unstructured data, leading to impressive results in fields like image and speech recognition. This sophistication makes it a beneficial choice for applications requiring high-level data analysis.
Nevertheless, Deep Learning systems can be resource-intensive and may necessitate specialized knowledge to implement effectively.
Natural Language Processing
Natural Language Processing, often referred to as NLP, deals with the interaction between computers and human language. NLP's key characteristic is its ability to understand, interpret, and generate language in a way that is both meaningful and useful. It has significant applications in customer service, content generation, and language translation.
The unique feature of NLP lies in its capacity to bridge the gap between human communication and machine understanding. However, challenges remain in ensuring accuracy and handling ambiguity within language constructs.
Current Trends in AI Development
The field of artificial intelligence is evolving rapidly, marked by developments that have significant implications across industries and society. Understanding current trends in AI is crucial for grasping the landscape in which we live. These trends not only reflect advances in technology but also showcase shifts in market dynamics. As AI integrates deeper into everyday processes, it prompts a re-evaluation of existing systems and necessitates new approaches to challenges arising from its capabilities.
Technological Innovations
Technological innovations drive the AI sector and set the stage for its future. Layer upon layer of development enhances our ability to process data, learn from it, and apply it in practical scenarios. Key areas to watch include:
Advancements in Neural Networks
Neural networks are foundational to modern AI, particularly in fields like image and voice recognition. Their ability to model complex patterns makes them indispensable. One key characteristic is their deep learning architecture, allowing multiple layers to extract intricate features from data. This complexity presents advantages in accuracy and efficiency but may introduce challenges in interpretability. For instance, decision-making processes can appear opaque, complicating accountability.
Edge Computing and AI
Edge computing refers to processing data at or near the source of information generation. This trend reduces latency and improves response times, which is vital for real-time applications such as autonomous vehicles and remote health monitoring. A notable advantage is bandwidth efficiency; by processing data locally, systems avoid extensive back-and-forth communication with centralized servers. However, ensuring robust security measures at the edge becomes critical, as vulnerabilities can arise from decentralized data management.
AI in Cloud Services
Cloud services play a pivotal role in democratizing access to advanced AI capabilities. They allow businesses of all sizes to leverage AI without substantial upfront investment in infrastructure. A standout feature of cloud-based AI is scalability. Organizations can adjust their usage according to real-time needs, making it a flexible choice. However, dependency on internet connectivity and potential data privacy issues merit consideration, as organizations must manage sensitive information responsibly.
Market Dynamics
Navigating the market dynamics reveals insights into how AI is perceived and implemented across sectors. Investment surges, mergers, and the entrepreneurial landscape provide a clearer picture of growth trajectories.
Investment Trends
Investments in AI have spiked, with venture capital flowing into startups developing cutting-edge technologies. These trends signify a broader recognition of AI's potential to drive economic growth. Investors are increasingly interested in applications that promise efficiency and innovation. However, speculative investments can lead to volatility, where expectations often exceed realistic appraisals of a companyβs progress.
Mergers and Acquisitions
Mergers and acquisitions serve as a barometer for industry confidence and strategy. Larger entities acquire smaller firms to integrate innovative technologies. This consolidation transforms the competitive landscape, often leading to faster advancements. However, too much consolidation can stifle competition and innovation in the long run.
Startup Ecosystem
The startup ecosystem is vibrant with a continuous influx of new companies. Many startups bring fresh perspectives to problem-solving. Their agility allows rapid experimentation and innovation. However, the challenge lies in market saturation; not all ventures will succeed, making it essential for investors and founders to differentiate and scale offerings effectively.
In the realm of AI, understanding current trends is not merely academic; it is essential for stakeholders aiming to navigate this evolving landscape responsibly.
AI Across Various Industries
The integration of artificial intelligence across various industries signifies a pivotal shift in how these sectors operate. As AI technologies evolve, they promise to enhance efficiency, reduce costs, and improve decision-making processes. Understanding the impact of AI on diverse fields helps in grasping its broader implications on society and the economy. In this section, we will explore specific industries where AI is already making an introduction, including healthcare, finance, and manufacturing.
Healthcare
Diagnostic AI Tools
Diagnostic AI tools are transforming how healthcare professionals detect and diagnose diseases. These tools apply machine learning algorithms to analyze medical data, such as images and patient records. One key characteristic is their ability to process vast amounts of data quickly. This speed allows for more accurate and timely diagnoses. The unique feature of diagnostic AI tools is their potential to assist clinicians by providing recommendations based on historical data and trends.
Some advantages include reduced human error and increased diagnostic speed. However, reliance on these tools raises concerns about data privacy and potential biases in decision-making. Understanding these elements is crucial for integrating AI effectively in medical practices.
Predictive Analytics
Predictive analytics in healthcare involves using statistical techniques and machine learning to forecast patient outcomes. This aspect plays a significant role in enhancing healthcare quality. A key characteristic is its ability to identify patterns that may predict diseases before they worsen. This proactive approach is beneficial for improving patient care and resource allocation.
The unique benefit lies in its ability to create targeted treatment plans for individuals, based on predictions. Yet, there are limitations as well, such as the need for large datasets which may not always be available. Balancing these factors is important for effective predictive healthcare.
Finance
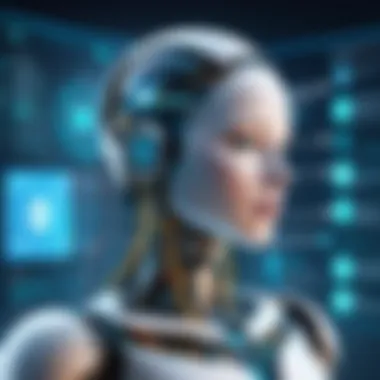
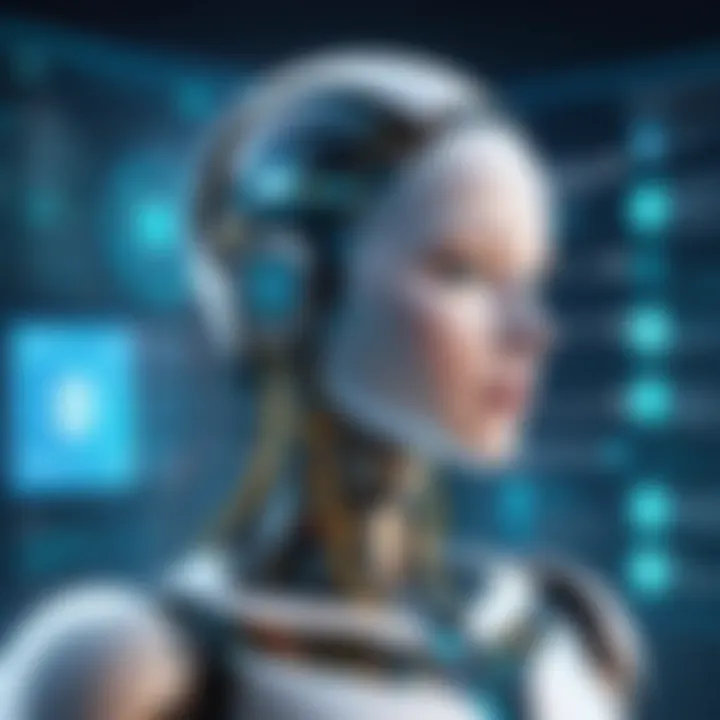
Algorithmic Trading
Algorithmic trading leverages algorithms to automate trading strategies at a speed and precision that humans cannot match. This component contributes significantly to financial markets by enhancing liquidity and reducing transaction costs. A notable characteristic is the ability to analyze complex market data in real time.
One unique feature of algorithmic trading is its reliance on historical data and advanced mathematical models to forecast market movements. While this can result in profitable trades, it can also introduce volatility into financial markets. Thus, understanding both advantages and risks is essential for stakeholders involved.
Fraud Detection
Fraud detection systems utilize AI to identify and prevent fraudulent activities in financial transactions. This key aspect is critical to safeguarding consumers and institutions. A primary characteristic is the ability to recognize patterns that indicate suspicious behavior in real-time.
The unique advantage of fraud detection AI is its adaptability. As fraud strategies evolve, AI systems can learn new patterns, enhancing their effectiveness over time. However, challenges remain regarding accuracy. False positives can inconvenience genuine customers, highlighting the need for a careful balance between security and customer experience.
Manufacturing
Autonomous Robots
Autonomous robots are increasingly becoming integral in manufacturing processes. They can perform tasks ranging from assembling products to shipping inventory. This technology is characterized by its ability to operate without human intervention, significantly speeding up production.
The unique feature of autonomous robots is their capacity to work continuously without breaks. This leads to increased productivity and reduced labor costs. However, a significant drawback is the initial investment and maintenance costs associated with implementing this technology. Assessing these factors is vital for organizations considering automation.
Predictive Maintenance
Predictive maintenance uses AI to forecast when machinery will require servicing. This aspect directly contributes to reducing downtime and optimizing maintenance schedules. A key characteristic of predictive maintenance is its reliance on sensor data and machine learning to assess equipment conditions.
The uniqueness of this approach lies in its ability to extend the lifespan of machinery by preventing unexpected failures. However, it does require a significant amount of data and upfront investment in sensors and AI systems. Organizations must evaluate their readiness for such technology to reap the benefits.
"The impact of AI across industries is not just about efficiency, but also about reshaping work itself and redefining roles."
Each of these industries illustrates the growing role of AI, emphasizing both opportunities and challenges. Thorough understanding and strategic planning are essential for organizations aiming to navigate this rapidly changing landscape.
The Societal Impact of AI
The discussion on the societal impact of artificial intelligence is essential in understanding its far-reaching consequences. AI influences how we interact, work, and even think. This section aims to disentangle the multifaceted growth of AI's influence on society, touching on the most pressing themes such as job displacement and human-machine collaboration.
Job Displacement
Automation of Repetitive Tasks
Automation through AI is reshaping various sectors by taking over repetitive tasks. This aspect is crucial because it addresses efficiency and productivity, effectively streamlining workflow. Companies often adopt automation to reduce costs and increase output. For example, assembly lines in manufacturing benefit immensely from robots that perform dull, monotonous tasks.
The main characteristic of this automation is its ability to work tirelessly, improving overall productivity. However, it poses a challenge: the elimination of certain job roles. Workers in repetitive positions face the risk of being displaced. Therefore, while automation brings efficiency, it also raises concerns about employment stability in traditionally secure positions.
Emerging Job Markets
Though job displacement occurs, emerging job markets also arise from AI developments. New positions focus on managing, developing, and maintaining AI systems. This shift is significant as it encourages innovation. The unique feature of these new roles is their focus on human skills, like creativity and problem-solving, which AI cannot replicate fully.
However, this transition has its challenges. Many displaced workers may lack the skills needed for these new roles, creating a gap that needs addressing. Retraining and education programs become vital, as they can bridge this divide and prepare workers for a landscape that increasingly incorporates AI.
Human-Machine Collaboration
Redefining Workforces
A notable aspect of human-machine collaboration is the redefinition of workforces. AI assists humans to enhance their capabilities, rather than simply replacing them. This synergy is increasingly acknowledged as beneficial for achieving higher output. For instance, tools like IBM's Watson aid professionals in fields like healthcare by providing insights that support decision-making.
This collaboration changes workforce dynamics, emphasizing the necessity for humans to develop complementary skills that work alongside AI. Nevertheless, it also raises concerns about over-reliance on technology, which may inadvertently reduce human initiative and critical thinking.
Skill Adaptation
Skill adaptation is crucial in navigating the AI landscape. As technology evolves, workers must adjust their skills to meet the demands of modern roles. Re-skilling and up-skilling are now essential strategies for professionals, allowing them to work effectively with AI tools. This characteristic highlights the importance of continuous learning and adaptability in today's work environment.
While skill adaptation has undeniable advantages, such as fostering resilience in workers, it also has disadvantages. The pace at which AI advances creates pressure on individuals to keep up. Those unable to adapt may find themselves at a disadvantage, increasing inequality in the workplace.
"The future of work is not about humans vs. machines, but rather how they can work together for greater efficiency."
Understanding the societal impact of AI, especially concerning job displacement and human-machine collaboration, is critical. The interplay between these elements defines our evolving relationship with technology and outlines the core challenges that lie ahead.
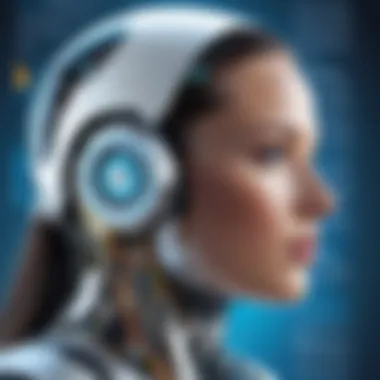
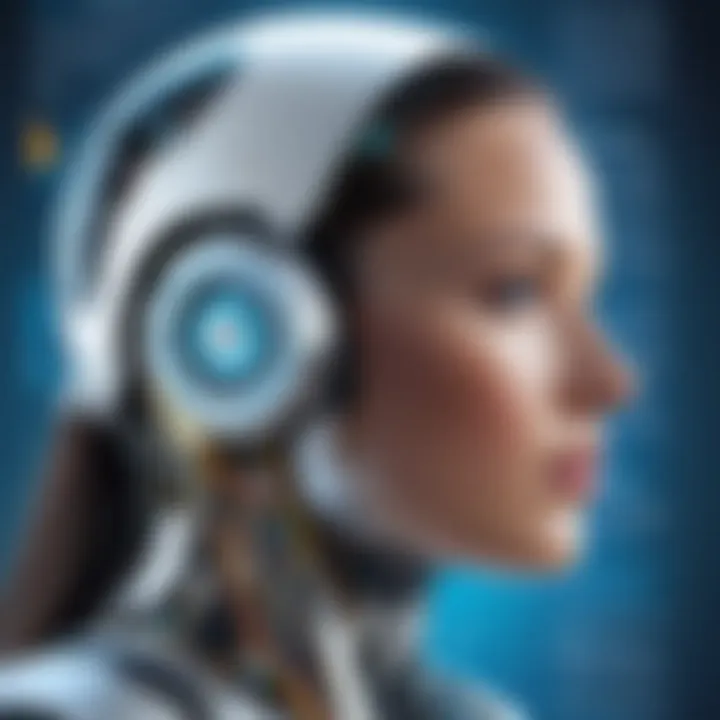
Ethical Considerations in AI
The rapid development of artificial intelligence technology brings with it significant ethical considerations. Addressing these concerns is crucial to ensure that AI systems are designed and implemented in ways that align with human values and societal standards. This section explores three key areas of ethical considerations in AI: bias and fairness, privacy concerns, and accountability and transparency.
Bias and Fairness
Bias in AI is a significant concern, as it can lead to unfair and discriminatory outcomes. AI systems often learn from historical data, which may contain inherent biases. These biases can then be perpetuated by the algorithms, resulting in skewed results that disadvantage certain groups. For instance, facial recognition technologies have demonstrated higher error rates for people with darker skin tones, raising questions about equity.
To mitigate these issues, it is essential to develop algorithms that are fair and inclusive. This can involve diverse data collection practices, regular audits of AI systems, and ongoing training to reduce bias in machine learning models. Moreover, stakeholders must prioritize equity when designing algorithms. Without a focus on fairness, AI applications can deepen existing societal inequities.
Privacy Concerns
With the proliferation of AI, privacy considerations have become more pronounced. AI systems often require large amounts of personal data for training and operation. This data, if mishandled, can lead to breaches of privacy and unauthorized surveillance. The use of AI in areas such as social media, customer service, and healthcare raises important questions about how data is collected, stored, and used.
It is vital to implement strong data protection measures and privacy frameworks to ensure that individuals' rights are respected. Regulation such as the General Data Protection Regulation (GDPR) in Europe sets a standard for data handling practices, emphasizing the need for consent and transparency. Organizations must prioritize the development of AI technologies that respect user privacy while providing valuable insights and services.
Accountability and Transparency
Accountability in AI refers to the responsibility of developers and organizations for the actions of their AI systems. As AI technologies become more autonomous, determining accountability for their decisions becomes complex. This challenges existing legal frameworks and necessitates a new approach to responsibility.
Transparency is equally important. Users should understand how AI systems make decisions, especially when those decisions significantly impact their lives. Clear communication regarding the workings of AI applications fosters trust and ensures informed consent from users.
"The challenge of ensuring accountability in AI systems requires collaboration across sectors, involving technologists, ethicists, and policy-makers."
To enhance accountability and transparency, organizations can implement clear guidelines for AI deployment. Regular assessments and disclosures regarding the decision-making processes of AI systems are also beneficial. Providing explanations for AI-driven decisions can help mitigate the potential for misuse and enhance user confidence.
Future Projections for AI
Future projections for AI hold significant weight in the ongoing discussions about its influence on society and innovation. Understanding these projections enables stakeholders to prepare for upcoming challenges and opportunities. This section investigates technological advancements and governance, which are key to navigating the AI landscape effectively.
Technological Advancements
Technological advancements in AI will likely redefine the boundaries of what is possible in various sectors. Improved algorithms will enhance the capabilities of machine learning and natural language processing, making AI systems more efficient and capable.
The focus on explainable AI will become more pronounced. As AI systems grow complex, the need for transparency in how decisions are made will rise. This is crucial for trust, especially in industries such as healthcare or finance.
Some forefront areas include:
- Quantum computing's potential to solve problems unimaginable today.
- Enhanced human-machine interactions, where AI can better understand context and emotion.
- The integration of AI with Internet of Things (IoT) technologies, leading to smarter cities and homes.
These advancements will create both opportunities and risks. While efficiency increases, ethical considerations raise concerns regarding data privacy and job displacement. It is vital to find a balance that fosters innovation while protecting values.
Global AI Governance
Global AI governance is an emerging field that focuses on establishing regulations and frameworks for AI deployment. As AI technology breaks borders, a unified approach will be necessary. Several important aspects are emerging in this strain of thought.
The international community must consider:
- Creating standards for AI ethics and data handling.
- Encouraging collaboration among nations to prevent a fragmented regulatory environment.
- Establishing accountability measures to ensure AI technologies are developed and used responsibly.
Collaborative efforts play a key role. Consistent guidelines across jurisdictions can help mitigate the risks of AI, such as misuse or bias. Countries like the European Union are taking steps, suggesting regulations that could influence global norms.
"The technology will soon outpace the policies if governments do not act collaboratively to govern AI."
Epilogue
In the discourse on artificial intelligence, conclusions are critical for understanding its broader implications on society. This section synthesizes the key points while emphasizing the importance of approaching AI integration with caution and foresight. The advancements in AI technology are rapid and transformative. However, they also come with challenges, requiring significant consideration of ethical impacts and societal changes.
Summary of Key Insights
The first insight to note is the profound effect AI is having on various industries, such as healthcare, finance, and manufacturing. Each sector is experiencing disruption, with AI tools enhancing efficiency and outcomes.
Moreover, the societal implications of AI deployment are significant. Job displacement due to automation is a pressing concern. Many traditional roles may diminish, paving way for new job categories that embrace cutting-edge technology.
In terms of ethics, ensuring fairness and accountability in AI systems is paramount. Bias in algorithms can reinforce existing inequalities and perpetuate discrimination. Addressing these concerns requires a commitment to transparency and responsible innovation.
Finally, global governance frameworks are essential to manage the trajectory of AI evolution and ensure that its benefits are widely shared. This involves collaboration among policymakers, technologists, and communities.
Call for Responsible AI Innovation
As we move forward into an era shaped by AI, responsible innovation becomes imperative. It is crucial to adopt practices that prioritize ethical considerations alongside technological advancements. Stakeholders must engage in discussions around the implications of AI and work toward frameworks that uphold fairness, accountability, and transparency.
Businesses and developers have the responsibility to scrutinize the impact of AI on jobs, privacy, and social structures. This also extends to ensuring equitable access to AI tools, preventing a divide between those who have and those who do not.