Mental Health's Evolving Dynamics with AI Technology
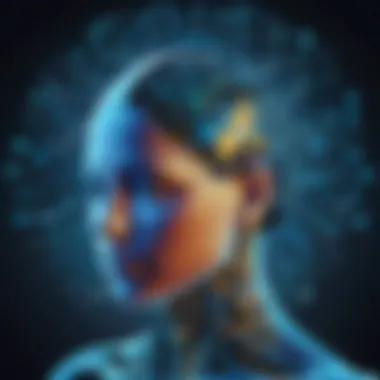
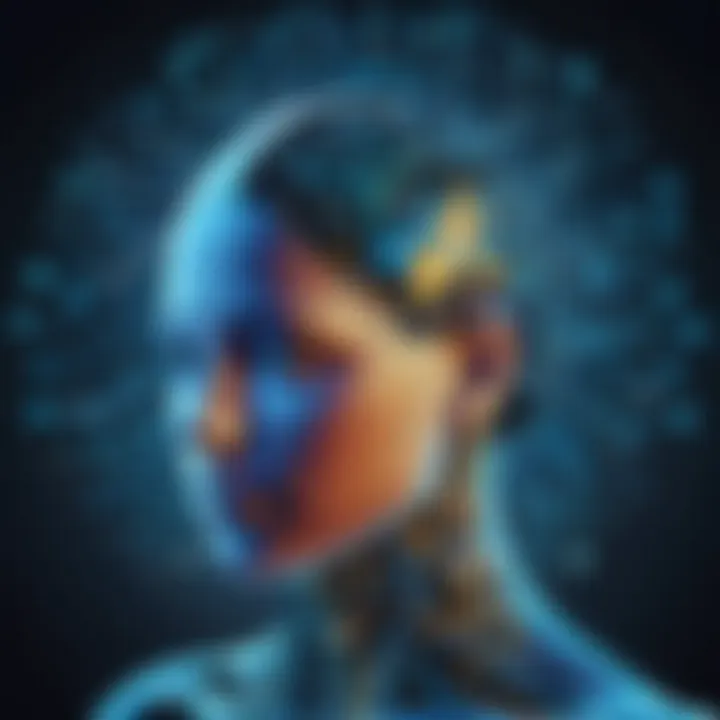
Intro
As we forge deeper into the 21st century, the landscape of mental health care is evolving at an unprecedented pace. The challenges associated with mental health have remained a persistent issue across various demographics, and they are more pressing than ever in our increasingly digital world. Enter artificial intelligence, a burgeoning field that is beginning to reshape the way we approach mental health diagnostics and treatments. The question lingers: How can AI both alleviate and exacerbate concerns within the mental health realm?
In this exploration, we will examine the multifaceted dynamics between mental health and AI, shedding light on its potential benefits and unforeseen challenges. This journey will encompass the deployment of AI technologies in therapeutic environments, ethical considerations that must guide their use, and how these advancements stand to influence diagnoses across the board.
Technology Insights
Latest Tech Trends
Artificial intelligence is not just future-talk anymore; it is here, shaking up industries left and right. In the mental health domain, the blend of machine learning and data analytics offers new methods for recognizing symptoms and patterns that may help in identifying mental health disorders. Tools like chatbots and mobile applications are being integrated into conventional therapy practices, allowing for more personalized care.
- Teletherapy: Incorporating video conferencing tools, therapists capitalize on AI to enhance virtual sessions without compromising on quality.
- Predictive Analytics: Platforms are using algorithms to analyze user data, assisting professionals in making more informed decisions based on behavioral trends.
Innovation in Tech
Custom-built AI solutions like Woebot are emerging as therapeutic companions. These applications utilize conversational AI to provide cognitive behavioral therapy techniques through a friendly chatbot interface. They can boost access to care, especially for those hesitant to approach traditional therapy.
"The integration of AI in mental health has the potential to democratize access to assistance, ensuring that help isn't just reserved for those who can afford it."
This commitment to accessibility is essential, yet it does not come without its challenges. The risks of dependent relationships on technology for mental health support should not be underestimated.
Product Reviews
A critical aspect of this exploration involves evaluating tools that are currrently available, based on user feedback and effectiveness. For instance, products like Talkspace and BetterHelp have garnered attention for their ease of use and diverse therapist options available to clients. However, it is crucial to analyze whether they genuinely meet mental health needs or merely provide a facsimile of care.
Ethical Considerations
The introduction of AI in mental health services brings along a myriad of ethical questions. How do we ensure privacy when dealing with sensitive user data? What measures should be put in place to prevent bias in AI algorithms?
- Data Privacy: As AI systems gather and analyze more information, the concerns over confidentiality mount. Users want reassurance that their personal struggles are safeguarded.
- Bias Detection: It’s paramount that AI technology does not reinforce existing stigmas or biases present in traditional healthcare systems. This requires continuous evaluation and refinement of algorithms.
The ethical chronicling of AI's role in mental health necessitates a proactive approach to prevent irresponsible usage that can potentially do more harm than good. Therefore, an urgent discussion surrounding these pivotal issues is warranted.
Epilogue
Bridging the gap between artificial intelligence and mental health care comes with its own unique set of challenges and opportunities. While innovative solutions publicly promise to enhance accessibility and effectiveness of mental health support, the implications of their use require meticulous scrutiny. The future of mental health care may indeed lie at the intersection of these two realms, armed with the responsibility to prioritize human well-being above all.
Foreword to Mental Health and Artificial Intelligence
The convergence of mental health and artificial intelligence (AI) is not just a passing trend; it's a paradigm shift that holds significant implications for both fields. By understanding how AI can interact with mental health care, we can uncover new dimensions of support and intervention that traditional methods might overlook. This article aims to navigate through the intricate pathways where these two worlds collide, emphasizing the potential benefits, challenges, and ethical considerations that come along.
Defining Mental Health in the Modern Context
Mental health is often misunderstood and sometimes minimized. In today's fast-paced society, the traditional definitions of mental health—rooted in decades of psychological study—are being challenged and expanded. A contemporary understanding goes beyond the absence of mental disorders. Instead, it encompasses emotional wellbeing, resilience, and the ability to cope with the stresses of daily life. The modern context also brings layers of complexity, where factors like social media influence, workplace pressures, and family dynamics intertwine, thus affecting individuals' mental states in ways that weren't as prevalent in previous generations.
For instance, a person can seem perfectly fine on the surface, maintaining a steady job and social connections, yet struggle with hidden anxieties or feelings of inadequacy. Defining mental health now means considering these nuanced experiences and recognizing that mental wellness is a spectrum—it's not a one-size-fits-all model. This understanding sets the stage for how AI could provide support tailored to these unique journeys, moving from vague assumptions to concrete, data-driven insights.
Overview of Artificial Intelligence Technologies
AI is no longer just a futuristic concept; it's woven into the fabric of our daily lives, impacting various sectors, including healthcare, education, and entertainment. At its core, artificial intelligence encompasses a range of technologies designed to replicate or simulate human cognitive functions. Machine learning, neural networks, natural language processing, and robotics are among the most discussed technologies today.
- Machine Learning (ML): This involves algorithms that can learn from and make predictions based on data. In mental health, it can assist in identifying patterns associated with different mental illnesses.
- Natural Language Processing (NLP): This enables machines to understand and respond to human language. It could, for instance, help therapists analyze their clients’ speech patterns to detect underlying issues.
- Robotics: Though a more futuristic aspect, the integration of conversational agents into therapy sessions is gaining traction. Such robots can offer companionship and facilitate initial screenings for mental health conditions.
The potential of these technologies lies in their ability to process vast amounts of information. They can uncover correlations in data that might escape human observation, offering healthcare professionals valuable tools to enhance patient care. Yet, the challenge remains: how do we integrate these intricate systems into a system that’s already complex—our mental health care?
Thus, as we explore this integration, it's essential to maintain a balanced perspective, drawing attention to how these AI technologies can support mental health initiatives without detracting from the human elements that are vital in caregiving.
Current Trends in Mental Health Solutions
When you look at the surface of mental health solutions today, it’s easy to spot just how rapidly the landscape is changing. Traditional methods, while still valuable, are now being augmented — or sometimes replaced — by new-age technology. This fusion is essential, not just for keeping up with the times, but also for creating more robust support systems for individuals grappling with mental health issues. As emerging trends paint a new picture, understanding these transformations offers crucial insights into how mental health care is adapting and evolving.
Traditional Approaches to Mental Health Care
Traditional mental health care methods often involve face-to-face interactions between patients and healthcare professionals, like therapists or psychologists. These approaches have stood the test of time, relying on established practices like talk therapy, medication, and support groups. However, they aren't without their hiccups. Access to care can be limited, with geographical constraints and stigma acting as significant barriers. In many regions, trained professionals are few and far between, leading to long wait times for appointments.
Moreover, the one-size-fits-all nature of many therapeutic strategies often leaves people feeling unheard and misunderstood. For example, someone struggling with anxiety might find that generalized cognitive behavioral therapy (CBT) doesn't adequately address their unique triggers. In short, while traditional methods have provided a lifeline to many, they often fall short for those requiring more personalized, immediate assistance.
The Role of Technology in Mental Health
In juxtaposition, technology is stepping into the breach, filling gaps traditional care can overlook, while hopefully enhancing overall effectiveness. Digital tools — ranging from mobile apps to telehealth services — afford convenience and accessibility that simply can’t be achieved through conventional means. These technologies allow users to reach out for help anytime and anywhere, effectively lowering the barriers that once existed in mental health care.
Consider AI-driven platforms that analyze user data to tailor interventions. These platforms can learn from a user's responses, adapting in real-time to better meet their needs. For instance, a subtle shift in wording in a chatbot’s response could significantly improve user engagement and trust. This tailored approach allows individuals to feel more connected, as the technology mimics the adaptive nature of human interaction, albeit in a digital form.
The role of technology also extends into larger ecosystems with AI systems analyzing trends, behaviors, and symptoms on social media platforms. Keeping a finger on the pulse of common mental health issues gives practitioners and researchers the intel needed to develop more effective outreach and prevention strategies.
Key Benefits and Considerations
- Accessibility: Tech solutions often break down geographical barriers, opening avenues for those in rural areas or regions with fewer mental health professionals.
- Scalability: Technology can reach a larger audience efficiently, allowing for more individuals to access support, even if not directly.
- User Empowerment: Mobile apps can enable users to track their moods, set reminders for medication, or even connect with a therapist through a few taps on their screens.
- Data-Driven Insights: The abundance of data collected from tech interactions can lead to improved understanding of mental health trends at the population level, shaping future care models.
As we navigate through this digital wave washing over mental health solutions, it becomes clear that blending technology with traditional care is no longer a nice-to-have but an urgent necessity.
"The future of mental health care will depend on our ability to harmonize the human element of therapy with the efficiency of technology."
Navigating these currents effectively will undoubtedly require caution and consideration, ensuring that the human-centric approach to mental health remains at the forefront, even as we embrace the benefits new technology brings.
Artificial Intelligence in Mental Health Diagnostics
The incorporation of artificial intelligence into mental health diagnostics offers an innovative approach that goes beyond traditional practices. It addresses the pressing need for accurate identification and tailored interventions for mental health disorders. With the increasing prevalence of mental health issues worldwide, leveraging AI technologies can facilitate early detection, provide insights from vast datasets, and ultimately improve patient outcomes. Moreover, embracing these advanced tools is crucial as they evolve in alignment with the growing complexity of mental health conditions.
AI Algorithms and Data Analysis
AI algorithms play a pivotal role in processing the complex data associated with mental health diagnostics. By using sophisticated statistical methods, these algorithms can analyze various types of information, such as patient histories, behavioral patterns, and even genetic markers. The efficiency of data analysis tools powered by AI means they can handle the heavy lifting of sifting through thousands of data points faster than any human can.
- Predictive Analytics: These algorithms can identify patterns that are not readily apparent, allowing for predictions about the onset of mental health issues based on early warning signs.
- Natural Language Processing: Through the evaluation of spoken or written language, AI can uncover emotional states, intent, and underlying psychological conditions that may require attention.
- Integration of Big Data: AI thrives on data; with access to extensive databases, it can continually improve, leading to more refined diagnostic tools. This is particularly valuable given that mental health disorders can manifest differently in each individual.
"The true potential of AI in mental health diagnostics lies in its capacity for continuous learning and improvement as more data gets fed into these systems."
Machine Learning Applications in Psychopathology
Machine learning, a subset of AI, is revolutionizing how we understand and diagnose psychopathologies. Unlike traditional methods, which often rely heavily on subjective assessments, machine learning models can analyze historical data to discover associations between symptoms and diagnoses.
- Visualization of Data: Machine learning can help clinicians visualize complex data trends, offering a clearer perspective on how different factors might correlate with specific conditions.
- Risk Stratification: By analyzing comprehensive datasets, these systems can classify the risk levels of patients, helping professionals prioritize cases that require immediate attention.
- Early Intervention: With the ability to detect symptoms earlier and more accurately, machine learning can support timely interventions, potentially improving long-term outcomes for individuals dealing with mental health issues.
In essence, our ability to understand mental health conditions is enhanced significantly through AI diagnostics. These advancements point to a future where personalized medicine is not just a concept but a practical reality in mental health care.
Therapeutic Applications of AI
The integration of artificial intelligence in mental health treatment is becoming increasingly prominent, evidencing a pivotal shift in therapeutic methodologies. The significance of this topic lies not only in enhancing the effectiveness of existing therapeutic approaches but also in addressing the gaps in accessibility and personalization of mental health care.
As mental health disorders continue to escalate globally, traditional therapeutic frameworks sometimes struggle to keep pace. AI technologies hold the potential to streamline and innovate therapeutic processes, thereby improving outcomes for patients and clinicians alike. Here, we’ll explore two notable applications of AI in therapy: AI-Driven Cognitive Behavioral Therapy and Virtual Mental Health Assistants.
AI-Driven Cognitive Behavioral Therapy
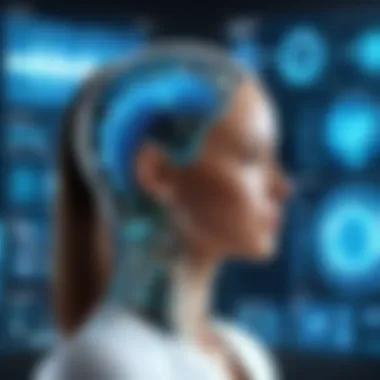
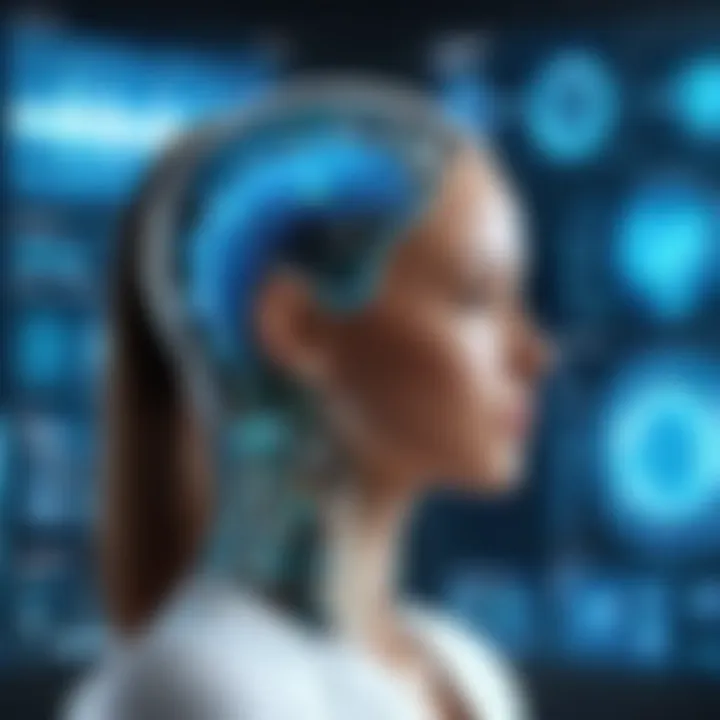
Cognitive Behavioral Therapy (CBT) is one of the most researched and effective treatment modalities for various mental health issues, including anxiety and depression. Incorporating AI into CBT can enhance its delivery and efficacy significantly.
AI-driven CBT typically leverages algorithms to provide tailored therapeutic interactions based on user data, thereby personalizing the treatment experience. The emotional insight and real-time feedback that AI can offer might prove invaluable. For instance, an AI platform can analyze a user’s input during therapy sessions to adjust the session’s focus in real-time, ensuring that the treatment is as relevant as possible.
One important benefit is scalability. Traditional therapy often faces barriers in availability, such as geographical limitations or therapist shortages. AI-powered applications can reach users well beyond those confines. This is particularly beneficial for individuals in remote areas or those who may hesitate to seek traditional help due to stigma.
Nonetheless, it's paramount to consider the limitations and ethical implications. The AI must respect patient confidentiality and data privacy, and although the technology can mimic empathetic responses, it lacks the human touch essential in therapy.
"The intersection of AI and mental health is not about replacing therapists but enhancing and expanding the therapeutic reach."
Virtual Mental Health Assistants
In recent years, we’ve also witnessed the rise of virtual mental health assistants—AI systems designed to support mental wellness outside of a hospital or clinic setting. These tools offer a plethora of functionalities, from basic emotional support to nudging users towards healthier coping mechanisms.
Such assistants, like Woebot or Wysa, utilize natural language processing to interact with users conversationally. They can recognize when a user exhibits signs of distress and guide them through difficult times with tailored coping techniques. This essentially affords users immediate support, available at any hour, making mental health care more accessible than ever before.
However, just as with AI-driven CBT, there are considerations to weigh. User adherence to these platforms can vary widely; some may find solace in having a "friend" to talk to, while others may feel uncomfortable opening up to a non-human entity. It's important for these tools to complement, rather than substitute, the human elements of therapy.
In summary, the therapeutic applications of AI represent a promising frontier in mental health care. Both AI-driven CBT and virtual mental health assistants deliver enormous potential for revolutionizing how we approach mental wellness, yet they demand a careful examination of ethical and practical considerations.
Ethical Considerations in AI and Mental Health
The intersection of artificial intelligence and mental health is not merely about transforming care; it introduces a minefield of ethical dilemmas that must be navigated with care. As AI technologies increasingly integrate into therapeutic tools and mental health diagnostics, understanding the ethical frameworks becomes pivotal. This section examines two key concerns: privacy and data security, alongside biases in AI algorithms—each a cornerstone in the broader conversation about responsible AI use in mental health care.
Privacy and Data Security Concerns
Managing sensitive information is like walking a tightrope. When it comes to mental health, privacy concerns amplify, as individuals seeking help often disclose deeply personal data. The crux of the matter lies in how this data is utilized and stored.
In a world where AI systems process oceans of data, ensuring data security becomes a formidable challenge. The potential for data breaches raises alarm bells; a leak could lay bare vulnerabilities that patients wish to keep under wraps. Regulatory frameworks like GDPR in Europe set high standards, but compliance can be a headache for many tech firms, especially startups with limited resources.
- Potential data breaches can lead to:
- Loss of Trust: If patients fear their data may not be secure, they might hesitate to seek help.
- Stigmatization: Exposure of mental health issues could lead to societal repercussions.
- Legal Ramifications: Inadequate data protection could precipitate lawsuits and damage reputations.
As such, transparency in AI algorithms that process this sensitive data should be paramount. Users have the right to know how their data is used and whether it contributes to improving treatment modalities. The challenge is not just about securing data; it's about instilling a sense of confidence in AI's role in mental health.
Bias in AI Algorithms
Not all that glitters is gold, and AI systems are no exception. Surprisingly, biases inherent in AI algorithms can skew the outcomes of mental health treatment recommendations, sometimes leading to misguided conclusions. These biases can stem from various sources, including the data sets used for training AI systems. If these sets lack diversity, the algorithms might inadvertently favor certain demographics while neglecting others.
Consider the following implications of biased AI:
- Misdiagnosis: Algorithms might misinterpret symptoms based on the predominant demographics in the training data, leading to inappropriate treatment suggestions.
- Limited Access: Underrepresented groups could end up receiving subpar care if algorithms fail to address their unique needs.
- Widening Healthcare Disparities: A biased approach risks exacerbating existing inequalities in mental health services, leaving vulnerable populations adrift.
It's crucial for developers and practitioners to engage in ongoing research to identify and mitigate biases in AI systems. Platforms should ensure a diverse range of data is included in training processes, and regular audits of the algorithms are a must to rectify any biases that emerge post-deployment.
"Ethics in AI is not just about technology; it's about ensuring that our humanity is preserved in the face of increasing automation." - Anonymous
Concluding Thoughts: As we traverse this complicated landscape, addressing ethical considerations surrounding privacy and bias in AI cannot be an afterthought. These issues intertwine with users' willingness to trust AI in mental health applications, directly impacting the success of these innovative solutions. Navigating this terrain with integrity will pave the way for more responsible, effective mental health interventions.
Challenges in Implementation
The journey of integrating artificial intelligence into mental health care is riddled with hurdles that need precise navigation. This section digs deep into the nuances of implementation, emphasizing how crucial these challenges are in reaping the potential benefits of AI technologies in this sensitive field. To harness AI effectively, one must address several obstacles, vary from technology limitations to human factors in existing care systems.
Integration into Traditional Care Settings
The transition from established therapeutic methods to AI-enhanced approaches poses significant hurdles. Traditional mental health care, dominated by human interaction and nuanced understanding, often views technology with skepticism. When introducing AI applications, the challenge lies not only in technology but also in how it fits into the already complex ecosystem of therapies, practices, and interpersonal relationships that define mental health treatment.
Here are some specific elements that make integration particularly challenging:
- Workflow Adaptation: Care providers often have set routines, making them apprehensive about altering their workflows. AI tools must seamlessly integrate into their existing processes without disrupting care continuity.
- Training Requirements: Professionals need proper training to utilize AI tools effectively. This training should encompass not just the technical aspects, but also the nuances of interpreting AI-driven insights, as misinterpretation could lead to inadequate care.
- Interdisciplinary Collaboration: Mental health care often involves a variety of practitioners, from psychologists to social workers. A unified understanding of how AI complements existing practices is crucial for fostering a collaborative environment, which is often easier said than done.
User Acceptance and Trust Issues
Trust is foundational in the mental health realm. For successful implementation of AI, patients must feel comfortable and safe while interacting with technology that makes health-related decisions. User acceptance often hinges on their perception of AI's reliability and efficacy. Here are crucial considerations that come into play:
- Data Security Concerns: Patients tend to worry about how their sensitive information is handled. Assurance of strict data protection practices is essential for building trust. If AI solutions cannot guarantee confidentiality, user acceptance will dwindle.
- Understanding AI Decision-Making: People have a natural tendency to question how decisions are made, especially when it involves something as personal as mental health. If AI systems operate as "black boxes," where the rationale behind decisions isn’t clear, individuals might be less inclined to trust these tools.
- Historical Context: With the proliferation of misinformation and some negative media portrayals of technology, many have developed reservations about AI in health care settings. Addressing these perceptions and emphasizing successful case studies is vital in shifting attitudes.
Integrating AI into traditional care won't happen overnight. It requires thoughtful dialogue, transparency, and an ongoing commitment to improving both technological and human elements of mental health care.
In summary, these implementation challenges are not mere roadblocks; rather, they reflect the complexities of human health care and the need for careful consideration of various aspects involved. Addressing these challenges thoughtfully lays a solid foundation for AI to function meaningfully within mental health services.
The Impact of Social Media on Mental Health
The interplay between social media and mental health is a complex one, interwoven with threads of both potential and peril. In our digital age, social media platforms have become the go-to space for connection, yet they also act as a double-edged sword, bringing about unique challenges related to mental well-being. Understanding this impact is vital not only for individuals navigating these online realms but also for developers and healthcare professionals aiming to leverage technology for better mental health outcomes.
Social media, with its vast reach and immense user engagement, presents an impactful avenue for sharing information, connecting with others, and seeking support. However, the importance of examining its psychological effects cannot be overstated. Here are some key elements to consider:
- Community Building: Social media has enabled users to find and connect with others facing similar struggles. It creates a sense of belonging that can foster resilience in the face of mental health challenges.
- Access to Resources: A plethora of mental health resources, such as articles, support groups, and self-help tools, are readily available through social media. This easy access can equip individuals with knowledge and coping strategies.
- Awareness and Reduction of Stigma: By generating conversations about mental health, social media can aid in challenging societal stigma. Awareness campaigns often go viral, encouraging users to share their experiences, thus promoting acceptance.
Yet, alongside these benefits lie significant considerations. Misuse of social media can trigger or exacerbate mental health issues, such as anxiety and depression. Factors such as cyberbullying, unhealthy comparisons with others, and addictive usage patterns contribute to negative psychological outcomes. To navigate this digital landscape wisely, understanding AI's role in social media monitoring is essential.
AI's Role in Social Media Monitoring
Artificial intelligence can be harnessed to monitor social media interactions and track mental health trends. By analyzing patterns in user behavior, sentiment, and engagement, AI serves as a powerful tool in identifying potential crises and aiding in preventive measures.
For instance, AI algorithms can sift through vast data sets to pick up on keywords and phrases relevant to mental distress. These can range from mentions of feeling suicidal to indicators of anxiety or depression. By flagging such content, organizations can proactively reach out to at-risk individuals with interventions or resources, thereby potentially saving lives. Moreover, AI can help discern the impact of social media campaigns aimed at reducing stigma and improving mental well-being, allowing for data-backed adjustments in strategy.
Negative Psychological Effects of Online Platforms
While social media platforms offer opportunities for connection and support, they come with notable psychological risks. The very features that make these platforms engaging can also lead to detrimental effects on mental health.
- Comparison Culture: Scroll through any social media feed, and one can't help but notice a relentless stream of seemingly perfect lives. This highlights a phenomenon known as social comparison, where individuals evaluate themselves against curated representations of others, often leading to feelings of inadequacy and low self-esteem.
- Fear of Missing Out (FOMO): The constant stream of posts can foster a fear of missing out on experiences or connections, which may lead to anxiety or feelings of exclusion. This pressure to stay involved can encourage compulsive scrolling behavior, further impacting mental health.
- Addiction to Likes and Validation: Many users become fixated on social approval through likes and comments, creating a cycle that relies on external validation. This need for affirmation can be particularly challenging for individuals already struggling with self-worth.
- Cyberbullying: The anonymity provided by online platforms can embolden negative behaviors, leading to harassment and bullying. The psychological toll of cyberbullying can manifest in depression, anxiety, and a plethora of negative emotional responses.
As we move forward, it becomes crucial to seek a balanced approach. Encouraging mindful social media usage, supported by AI-driven monitoring, may pave the way for a healthier relationship with these platforms. The journey towards integrating technology and mental health lies in understanding both the blessings and burdens that come with our digital lives.
Future Prospects of AI in Mental Health
The future landscape of mental health care is increasingly intertwined with advancements in artificial intelligence. As technologies evolve, they not only promise improved patient outcomes but also raise essential questions regarding ethical practices and implementation strategies. The intersection of these fields holds immense potential for developing innovative solutions tailored to the needs of individuals facing mental health challenges.
Innovations on the Horizon
The next wave of innovations in AI promises to reshape mental health services profoundly. For instance, imagine AI-driven tools that can analyze patterns in speech and behavior to detect early signs of mental health issues. Such systems might leverage natural language processing to examine language cues that indicate anxiety or depression levels. These tools can be crucial in preventing more severe mental health episodes before they escalate.
- Predictive Analytics: By utilizing huge sets of health data, AI can identify trends that lead to mental health conditions. This predictive capability can help in risk assessment and intervention planning, enabling more proactive care.
- Wearable Technology: Wearable devices that monitor physiological data—such as heart rate variability or sleep patterns—can also play a significant role. When integrated with AI algorithms, they can offer real-time feedback based on an individual’s mental state, thus fostering an environment of self-awareness.
- Teletherapy Enhancements: AI can optimize teletherapy sessions by matching patients with therapists based on their unique needs and issues. This could revolutionize access to mental health care, particularly in underserved areas.
"The integration of AI in mental health is paving the way for nuanced approaches to care, allowing practitioners to connect with patients like never before."
As we peer into this prospective future, it's clear that the innovations emerging may streamline therapy, enhance diagnostics, and facilitate a deeper understanding of mental health.
Potential for Personalized Mental Health Care
Personalization is becoming a crucial element in mental health treatment, and AI is at the forefront of this shift. Tailored interventions can be offered through AI platforms that learn from individuals' behaviors and feedback over time.
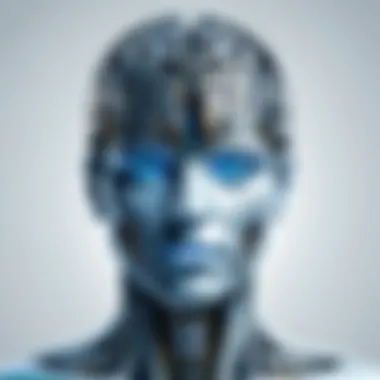
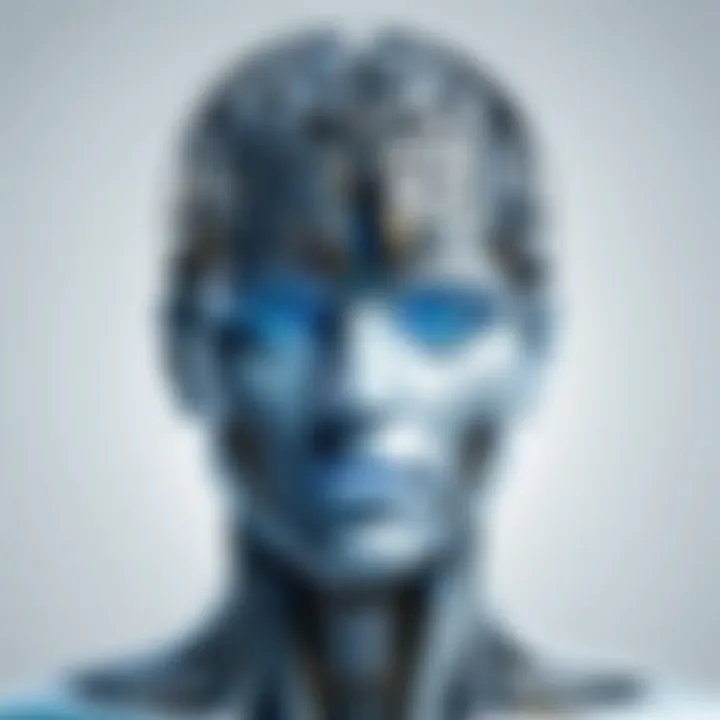
- Dynamic Treatment Plans: AI can assist in developing dynamic treatment plans that evolve based on a person’s progress. For instance, if a specific intervention seems to work well, the AI can recommend increasing its focus.
- User-Centric Applications: Mental wellness applications powered by AI can analyze user interactions to suggest content, activities, or exercises designed to improve their mental state. This type of customization addresses the unique experiences of each individual, as everyone’s mental health journey is inherently personal.
- Integration with Medical Records: By correlating AI findings with electronic health records, practitioners can gain insights into how various treatments have historically performed with similar demographics or conditions.
Through these modalities, AI stands to usher in a new era of personalized mental health care. The goal is not a one-size-fits-all solution but rather a comprehensive approach that acknowledges and caters to individual differences in mental health experiences.
Case Studies of AI in Mental Health
In the realm of mental health, truly understanding the impact of artificial intelligence calls for a closer look at real-world applications. Case studies shed light on the capabilities of AI and help us grasp its role in enhancing mental health services. Through specific examples, we can explore both the potential benefits and the pitfalls associated with AI implementations in this sensitive field.
Successful Implementations
Several noteworthy projects illustrate the vibrant intersections of AI and mental health. One shining example is Woebot, a chatbot designed to provide emotional support and cognitive behavioral therapy. Users interact with Woebot to track their moods and discuss thoughts and feelings. The positive feedback from numerous users highlights its effectiveness in identifying mental health issues early and promoting positive coping strategies. It's like having a personal coach available 24/7, ready to offer advice at a moment's notice.
Another success story involves the AI platform, Wysa, which employs natural language processing to recognize patterns in user conversations. It can adapt responses based on the emotional state of the user, creating a more personalized experience. This adaptability can be a game changer in mental health care, engaging users more effectively by understanding their unique perspectives and feelings.
"Case studies demonstrate that AI can fill critical gaps in mental health support, especially for underserved populations."
Moreover, researchers at Stanford University developed a machine learning algorithm capable of predicting depression from social media activity. By analyzing users' postings, likes, and interactions, the system can flag those who might be at risk of developing mental health issues. This proactive approach could redefine early intervention strategies, ensuring individuals receive help when they need it most.
Lessons Learned from AI Failures
While success stories are inspiring, the awareness of failures is equally crucial. One of the alarming cases comes from a well-known mental health app that used AI to analyze voice patterns in order to detect depressive symptoms. Initial results were promising; however, further scrutiny revealed that the algorithm struggled with different accents and dialects, leading to misinterpretation of behaviors. This highlighted a significant oversight: the need for diverse datasets to ensure inclusivity and accuracy in mental health applications.
Another stark lesson arises from an AI-driven program intended to assist therapists in diagnosing mental health disorders. The system, though sophisticated in its intent, exhibited biases based on the training data used. This not only skewed results but also raised concerns about the equity of AI interventions in mental health care. If these biases remain unaddressed, they could inadvertently deepen existing disparities in mental health services.
Reflecting on such lessons is critical for future developers and investors in the field. A thoughtful approach to algorithm design, emphasizing inclusion and robustness, ensures that AI technologies serve a wide audience rather than create further divides.
The exploration of these case studies, both successful implementations and the lessons learned from failures, serves to highlight the intricate dance between innovation and caution. In navigating the future of AI in mental health, stakeholders must embrace transparency and continuous improvement, fostering a system that genuinely benefits all.
AI and Mental Health Research
The confluence of artificial intelligence (AI) and mental health research has ushered in a fresh era for psychological studies and therapeutic approaches. This intersection holds significant promise for refining existing methodologies, enhancing diagnostics, and broadening the horizons of treatment modalities. With AI's capabilities, analyzing vast datasets becomes feasible, enabling researchers and practitioners to glean insights that would have previously taken years of laborious study.
The importance of focusing on AI in mental health research lies in its potential to revolutionize how we understand and respond to mental health challenges. AI can assist in identifying patterns in mental health disorders that may not be immediately evident through traditional research methods. For instance, analyzing behavioral data from mobile apps or social media activity can unveil trends linked to anxiety or depression, allowing for timely interventions.
Additionally, AI technologies can lead to more personalized care, a crucial aspect in mental health treatment. By leveraging machine learning algorithms, researchers can tailor therapeutic approaches to individual needs, ensuring better outcomes. The integration of AI in this field raises essential considerations regarding ethical practices, data privacy, and the need for robust regulatory frameworks.
"Emerging technologies in mental health research are not just about increasing efficiency but also transforming lives by providing more effective and personalized care."
Advancements in Research Methodologies
The advancement of research methodologies through AI is profound and multifaceted. Traditional research can be likened to sailing in a small boat; slow and sometimes turbulent. But with AI, it’s more akin to cruising in a state-of-the-art vessel. AI introduces tools that analyze behavior, neural patterns, and emotional responses with unprecedented precision.
- Big Data Analysis: AI facilitates the analysis of extensive datasets derived from clinical studies, surveys, and real-time behavioral tracking, detecting correlations and generating insights at a scale that humans alone could not manage.
- Automation of Research Tasks: Repetitive tasks such as data entry and preliminary analysis can be automated, freeing researchers to focus on higher-level insights and deeper analysis. This efficiency does not compromise quality—instead, it enhances the overall research process.
- Predictive Modeling: Utilizing AI in predictive analysis allows researchers to forecast mental health trends and potential crises by processing historical data along with current variables.
- New statistical methods led by AI algorithms help refine the parameters essential for understanding different mental health conditions.
- For example, an AI system trained on historical patient records might predict which individuals are at elevated risk for anxiety episodes based on subtle behavioral changes.
Collaborative Efforts Between Tech and Mental Health Fields
The collaboration between technologists and mental health professionals is essential to harness the full potential of AI in research and clinical practice. This interdisciplinary approach fosters innovation that benefits both sectors. Here’s how these partnerships are taking shape:
- Joint Research Initiatives: Universities and tech companies are joining forces to explore new AI tools for mental health assessment and treatment, leading to pilot programs that test ground-breaking methodologies.
- Conferences and Workshops: Regular events focusing on AI applications in mental health are being organized, providing platforms for knowledge sharing and professional development across disciplines. These interactions can spark new ideas and validate existing hypotheses.
- Open Source Platforms: Tech firms are developing open-source frameworks for AI tools that can be modified and utilized by mental health researchers. This inclusivity accelerates innovation and expands access to cutting-edge resources.
Through these collaborative efforts, the fusion of AI and mental health care not only enhances research capabilities but also aims to create solutions that are both economically and socially beneficial.
Public Perception of AI in Mental Health
Public perception of AI in mental health is a crucial aspect of the broader discussion surrounding the integration of technology in care. Understanding how society views AI can illuminate not just the acceptance of AI-driven solutions but also the potential barriers to their effective implementation. The potential benefits of AI, such as improved access to resources and personalized interventions, can only be realized if the public is sufficiently informed and trusting of these technologies.
As AI systems are increasingly used in mental health diagnostics and treatment, public attitudes can shape the future landscape of mental health services. Concerns regarding privacy and efficacy—how well these tools actually help—must be navigated thoughtfully. Overall, a balance must be struck between innovation and the need for public assurance about the safety and reliability of AI applications in mental health.
Surveys and Studies on Public Attitudes
Recent surveys reveal a nuanced public attitude toward AI in mental health. People are generally optimistic about AI's potential to enhance mental health care, but there are significant concerns that underpin this optimism. For instance, a study by the American Psychological Association (APA) found that:
- 62% of respondents believe AI could improve access to mental health services.
- 45% express concerns over data privacy when using AI tools.
- 53% are uncertain about how effective AI technologies might be compared to human therapists.
These statistics show a clear divide; while many are eager to embrace new technologies, others remain apprehensive due to unresolved issues.
Furthermore, demographic factors also play a role. Younger individuals, often more technologically savvy, tend to view AI advancements more favorably than older generations who may be less comfortable with technology. The effectiveness of outreach and education campaigns will also be determinative in shaping favorable perceptions.
The Influence of Media Depictions
Media portrayal significantly shapes public perception of AI in mental health. Positive representations can demystify technologies and foster greater trust, while negative or sensationalized depictions can instill fear and skepticism.
Popular shows and films often depict AI as a replacement for human interaction, which can skew public understanding. For example, a show where an AI therapist is portrayed as infallible might lead viewers to believe that AI can entirely replicate human empathy and understanding. Such representations overlook the nuances of human emotion, which makes mental health care so intricate and personal.
Conversely, documentaries or informative segments that highlight success stories—showing AI assisting professionals rather than replacing them—are likely to foster a more positive outlook. To improve public acceptance, clear, factual depictions of AI's role in mental health care should be prioritized over dramatized accounts.
"As technology advances, shedding light on the real-world implications of AI in mental health is vital for dispelling myths and fostering trust among users."
In summary, public perception is pivotal to the advancement of AI in mental health. Continuous research and careful media representation must work hand-in-hand to ensure that society grasps the complexities of technology without falling prey to misconceptions.
Regulatory and Policy Frameworks
Regulatory and policy frameworks are like the backbone of mental health solutions that use artificial intelligence. They establish guidelines, ensure safety, and promote interoperability between systems, making sure every player in the field acts responsibly. Given the sensitive nature of mental health data, it's crucial to have a robust set of regulations. They help mitigate risks related to privacy, security, and bias in AI algorithms, which can profoundly impact patients’ lives. This not only protects users but also instills trust in these technologies, ensuring that clients feel safe engaging with AI-driven resources.
Current Regulations Governing AI in Health
The current regulatory landscape governing AI in health is quite fragmented, as the laws tend to vary from country to country, and even within states or regions. In the United States, for example, the Food and Drug Administration (FDA) has started to lay down policies specifically for digital health applications. For instance, software that acts as a diagnostic tool or treatment advisor may require rigorous testing and approval, similar to traditional medical devices.
In the European Union, the General Data Protection Regulation (GDPR) plays a significant role in shaping how data is collected and used, especially when it involves personally identifiable information. This law demands transparency from AI systems, requiring that users are informed about how their data will be used.
Moreover, frameworks may also undulate in response to developments in technology. The draft of the EU AI Act outlines classification for AI systems according to risk levels, ensuring that high-risk systems, such as those used in mental health care, undergo stringent evaluation before they're allowed on the market.
- Data Protection: Regulations safeguard patient data by enforcing strict data management practices.
- Quality Assurance: Standards ensure that AI-driven tools meet qualitative benchmarks before reaching the public.
- Patient Safety: Regulatory bodies assess the impact of AI on patient care, ensuring the minimization of risks.
Future Policy Recommendations
Looking ahead, there are several noteworthy policy recommendations that could enhance the integration of AI in mental health services.
- Harmonization of Regulations: To foster innovation while ensuring safety, a more unified regulatory framework across countries and regions is needed. This would simplify compliance for companies working in multiple jurisdictions.
- Involvement of Stakeholders: Policymakers should involve mental health professionals, tech developers, and patients in the regulatory process. Their insights can inform guidelines that balance innovation with ethical considerations.
- Adaptive Regulations: As technology evolves, so should the regulations governing it. A regulatory framework that is flexible and adaptable can ensure that AI technologies remain safe and effective without stifling innovation.
- Funding for Research: Increased funding for research into the efficacy and safety of AI mental health tools is crucial. This would help gather essential data to inform future regulations and create a more robust understanding of AI's impact on mental health services.
"The intersection of regulation and innovation offers a fertile ground for advancements in mental health, provided that data privacy and user safety remain paramount."
With a proactive approach towards regulatory frameworks, the challenges posed by AI in mental health can be transformed into opportunities for deeper care and connection.
Comparative Analysis with Other Technologies
In the realm of mental health, understanding the comparative strengths and limitations of AI technology alongside traditional therapeutic methods is vital. This analysis not only sheds light on the emerging trends in the mental health sector but also highlights the unique role that AI can play in enhancing therapeutic efficacy and outcomes.
AI technologies have proven useful in streamlining diagnostic processes and personalizing treatment. By evaluating vast amounts of data, these systems can identify patterns that might escape human observation.
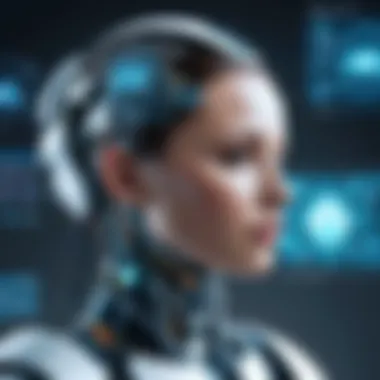
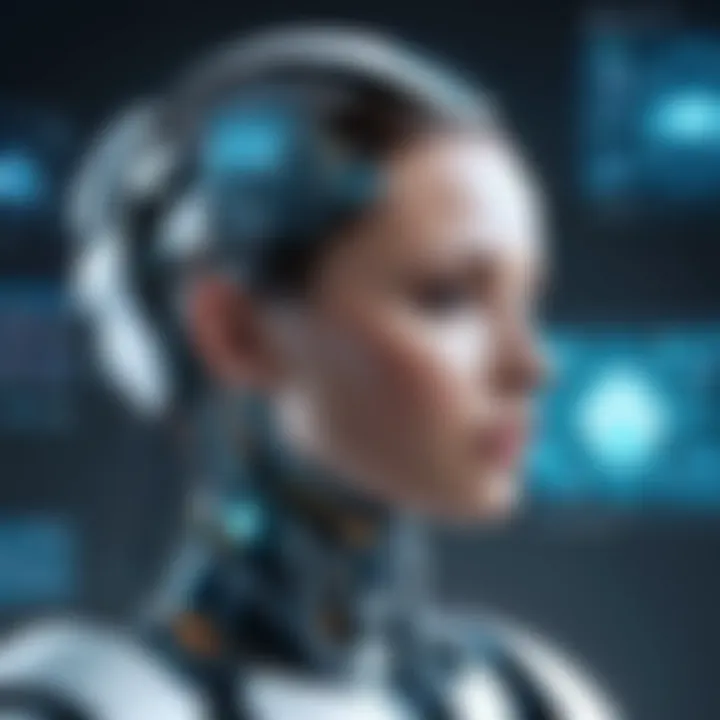
However, juxtaposing AI with traditional therapeutic tools raises important questions:
- Effectiveness: AI might analyze a slew of data, but can it truly connect with patients the way human therapists do? The human touch remains indispensable in many therapeutic scenarios.
- Accessibility: AI has the potential to break down geographic barriers, making mental health care accessible to remote or underserved communities. Traditional tools often falter in these areas due to logistical constraints.
- Cost: The financial implications of AI can be favorable. In many cases, AI solutions offer more cost-effective alternatives to conventional therapy, which often involves a longer time commitment and higher expenses.
- Training: Using AI tools requires a certain level of tech-savviness. Therapists must adapt to the evolving landscape, understanding how to integrate these tools into their practice without losing the human element.
The necessity to analyze the strengths of AI alongside traditional treatments helps to tailor approaches that cater to diverse patient needs and preferences.
"AI's potential lies not in replacing human therapists but in augmenting their capabilities, enhancing overall patient care."
AI Versus Traditional Therapeutic Tools
When you stack AI against traditional therapeutic tools, it’s like comparing an old-fashioned radio to a sleek streaming service. While radio has its charm and a loyal audience, streaming offers on-demand access and tailored playlists based on listening habits.
In the same way, traditional therapy happens in a face-to-face context where human connection takes center stage. Therapists possess empathy, body language appreciation, and the nuances of real-life scenarios. These elements are difficult to replicate in AI-driven platforms
However, as technology advances, hybrid models that include AI tools can augment therapy sessions, providing therapists with valuable data insights.
AI may assist therapists in:
- Predicting patient outcomes based on historical data
- Monitoring patient engagement outside of therapy
- Suggesting personalized mental health strategies based on individual behavior patterns
Examining Synergies with Other Modalities
The potential for synergy between AI and other therapeutic modalities like mindfulness and art therapy can expand therapeutic horizons significantly. Technologies, such as virtual reality and biofeedback devices, are paving the way for multifaceted treatment modalities where AI plays a central role.
For example, imagine a scenario where a patient engaged in art therapy is also using AI to track facial expressions and emotional response during sessions. The AI can analyze this data to suggest patterns or changes, helping therapists adjust techniques in real-time to maximize efficacy.
Benefits of such synergistic approaches include:
- Enhanced Data Collection: Multiple modalities can generate richer datasets that provide insight into the patient's progress.
- A Holistic Approach: Combining various therapeutic styles caters to the diverse needs of patients.
- Innovation Drive: The fusion of different techniques can lead to innovative therapies that redefine mental health interventions.
In summary, examining how AI interfaces with traditional therapeutic tools and other modalities uncovers a landscape ripe for advancement. Such comparative analyses help to anchor the understanding of AI's role, paving the way for responsible and effective integration into mental health practices.
Personalization of Mental Health Strategies Using AI
The integration of artificial intelligence into mental health care offers a sea change in individualizing treatment approaches. Personalization of mental health strategies emerges as not just an innovation, but a necessity in an age where mental health issues are pervasive and varied. Unlike one-size-fits-all treatments from yesteryears, AI paves the way to craft tailored interventions that resonate with the unique experiences and symptoms of each individual. Doing so addresses the nuances of mental health disorders, which are often deeply entwined with personal history, environment, and even genetic predispositions.
Tailored Interventions through Data Insights
AI excels at sifting through mountains of data to extract relevant insights that can inform targeted interventions. For instance, consider the myriad data points collected through various channels: clinical records, self-reported symptoms, and even social media activity. The ability of AI to analyze this data is not just a technical marvel; it’s an essential step to formulating personalized treatment plans.
Imagine a scenario where AI-driven platforms analyze patterns from collected data: it might identify that a user experiences heightened anxiety during particular times of the year or in specific social settings. By recognizing such trends, these platforms can recommend tailored cognitive behavioral therapy exercises or mindfulness activities specifically designed to mitigate those stressors. This level of customization means that individuals aren’t merely following a set protocol; they’re engaging in a therapeutic process that is dynamically adjusted to their day-to-day lives.
"The future of mental health care is personalized care fueled by AI, bridging the gap between individual needs and clinical expertise."
- The trend is clear: patients who receive customized care often report better outcomes and increased engagement with their treatment regimen. Personalized AI solutions empower clinicians to allocate their resources effectively and foster an inclusive treatment environment.
The Rise of AI-Based Mental Wellness Applications
As the digital realm expands, we're witnessing a proliferation of AI-based mental wellness applications designed to support individuals on their personal journeys toward better mental health. Solutions such as Woebot and Wysa utilize natural language processing to interact with users in a conversational manner, providing support tailored to their emotional states. The algorithms behind these applications learn from user interactions, enhancing their ability to offer relevant advice and coping strategies.
Moreover, the accessibility of these applications has democratized mental health resources. Where in-person therapy might have been cost-prohibitive or logistically challenging, mobile apps provide an avenue for instant support. Users can seek guidance and interventions at their fingertips, any time of day.
The Economic Impact of AI on Mental Health Services
The integration of artificial intelligence into mental health services is not just a technical phenomenon, it stands to reshape the economic landscape of mental health care. Understanding this impact is crucial for stakeholders across the board, from healthcare providers to policymakers and patients. As we navigate this intersection, it's imperative to analyze the cost implications, funding trends, and the overall economic benefits that AI can bring to this field.
Cost-Effectiveness of AI Solutions
When it comes to mental health treatment, costs can quickly balloon—often without corresponding improvements in patient care. Traditional therapeutic approaches often overwhelm healthcare budgets, and this is where AI solutions step in. By employing algorithms for diagnosis, automated therapy, and continuous patient monitoring, healthcare providers can achieve significant cost savings.
- Reduced Labor Costs: AI can handle routine tasks like initial assessments, freeing up mental health professionals to focus on more complex cases. This not only boosts efficiency but also reduces the overall staffing expenses.
- Improved Resource Allocation: AI can analyze patient data to identify which resources are most needed. For instance, if a batch of patients exhibits similar symptoms, treatment may be standardized, eliminating unnecessary expenses.
- Lowered Long-Term Costs: By catching mental health issues early and offering timely interventions, AI can potentially reduce the need for more expensive, long-term care down the road.
These cost savings do not merely help institutions; they can also enable fairer access to services, which is an important consideration in mental health care. If costs can be minimized, more resources can be directed toward low-income patients who often struggle to obtain quality care.
Funding and Investment Trends
The surge in AI technologies has piqued the interest of investors and governmental agencies alike, leading to notable trends in funding for mental health services. Financial backing is crucial for research, development, and implementation of AI systems, and several factors are driving investment in this sector.
- Public Funding Initiatives: Many governments are recognizing the benefits of incorporating AI into mental health and are launching grants to fund research and pilot programs. The intent is clear— to improve public health outcomes while simultaneously managing budget concerns.
- Venture Capital Interest: There's a growing interest among venture capitalists in mental health tech firms. Startups that marry technology with therapeutic practices are attracting significant investments, signaling confidence in the potential of AI to disrupt traditional mental health paradigms.
- Collaborative Funding Models: Partnerships between tech companies and mental health agencies are becoming more prevalent. By pooling resources, these collaborations can amplify the impact of their innovations, leading to more robust funding channels.
The investment landscape is evolving, with numerous entities recognizing that integrating AI can yield substantial financial returns while enhancing care quality. However, for sustainable growth, it is vital that investments are coupled with rigorous evaluations to ensure their efficacy and ethical application in mental health practice.
The economic implications of AI in mental health extend beyond immediate financial metrics—they represent a shift in how care can be delivered, making it more accessible and effective.
Technological Challenges in AI Implementation
As artificial intelligence becomes a driving force in mental health solutions, several technological hurdles arise that can impede progress. Addressing these challenges isn't just a minor detail; it's crucial for developing effective and reliable AI applications in mental health care. The importance of overcoming these technological challenges resonates on multiple levels, including promoting user acceptance, ensuring accurate diagnostics, and enhancing therapeutic outcomes.
Data Quality and Availability Issues
When it comes to AI in mental health, the old adage "garbage in, garbage out" rings particularly true. The efficacy of any AI system hinges on the quality of the data that feeds it. If the underlying data is skewed, sparse, or of low quality, the results will be anything but reliable. A common scenario is when user-provided data lacks context or is incomplete due to privacy concerns. As a result, mental health algorithms might misinterpret symptoms, leading to potentially harmful outcomes for patients.
Access to relevant and diverse datasets is another mountain to climb. Mental health conditions often span cultures and demographics, and the data needed for a comprehensive understanding is fragmented. AI systems may struggle to learn effectively when the training data is not robust or representative of the broader population. Tantrums of bias can creep in, resulting in therapeutic models that don’t cater to all individuals, undermining the fundamental goal of mental health care.
- Strategies for Improvement:
- Collaborations among healthcare providers, researchers, and tech companies to aggregate large, high-quality datasets.
- Utilizing anonymization techniques to encourage data sharing while respecting patient privacy.
- Regularly updating algorithms to adapt to new data sources and findings in mental health research.
Technical Limitations of Current AI Systems
Even as we explore advanced AI technologies, it's important to recognize their limitations, particularly in mental health applications. Traditional AI models often operate as black boxes, producing results without transparency in their logic. This lack of interpretability makes it hard for mental health professionals to trust AI-driven diagnoses and recommendations. After all, mental health care is inherently personal and nuanced; a one-size-fits-all approach simply won't cut it.
Moreover, current AI systems may struggle to handle the complexity of mental health conditions. Conditions such as anxiety, depression, or PTSD can present with a wide range of symptoms, and they don’t always follow a predictable path. AI systems trained on straightforward datasets may underperform when faced with reality's messiness.
"AI systems can only be as nuanced as the data they are trained on, and mental health is anything but linear."
- Current limitations include:
- Over-reliance on quantitative metrics, often ignoring qualitative insights from patient interactions.
- Difficulty in recognizing the socio-cultural factors impacting mental health, which can lead to misdiagnosis.
- Slow adaptation to new treatment methods or psychological insights, stifling innovation in this evolving field.
As we look deeper into the integration of AI in mental health, addressing these technological challenges is not merely advisable; it becomes imperative. Ensuring data quality and overcoming technical limitations plays a critical role in building trust and efficacy in AI-facilitated mental health care.
Closure: Navigating the Future of Mental Health and AI
Summarizing Key Insights
Throughout this article, key insights have emerged that shed light on how AI is transforming mental health initiatives. Here’s a brief summary:
- Enhanced Diagnostics: AI algorithms are being utilized in diagnostics, offering more precise assessments, which may lead to early interventions.
- Therapeutic Innovations: The rise of AI-driven therapies, such as virtual assistants and cognitive behavioral therapy apps, provide accessible solutions to those who might not otherwise seek help.
- Ethics in Technology: Privacy and data security continue to be paramount, posing a dilemma that must be navigated with care as we integrate these technologies into everyday practice.
- Public Perception Matters: The way AI technologies are depicted in media and public discourse shapes user acceptance. Negative portrayals could hinder the adoption of beneficial tools.
- Economic Considerations: Cost-effectiveness is a growing narrative, suggesting that AI could help alleviate some financial burdens associated with traditional mental health services.
These insights reinforce the notion that while AI holds great promise, it also requires cautious and informed implementation.
The Critical Path Forward
Moving forward, a critical path is necessary to ensure that the integration of AI in mental health does not merely serve profit-driven motives but champions mental wellness. Important considerations include:
- Collaboration Across Disciplines: There's a need for ongoing collaboration between tech experts, mental health professionals, and policymakers. This ensures that innovations prioritize the wellbeing of users rather than just technical prowess.
- Establishing Robust Regulations: Clear regulatory frameworks must be developed to govern AI applications in mental health. This includes defining responsibilities regarding data privacy and treatment efficacy.
- User-Centric Design: Tools and applications must be designed with end-users in mind. Understanding user needs and experiences can inform better development processes, fostering trust.
- Ongoing Research and Feedback: Continuous research is vital to monitor how these AI solutions impact mental health outcomes. Listening to feedback from users will be instrumental in making iterative improvements.
In sum, as we navigate the future landscape of mental health and AI, being proactive rather than reactive will allow us to unlock the full potential of these technologies while safeguarding human dignity and privacy.