Exploring the Intricacies of Autonomous Driving Technology
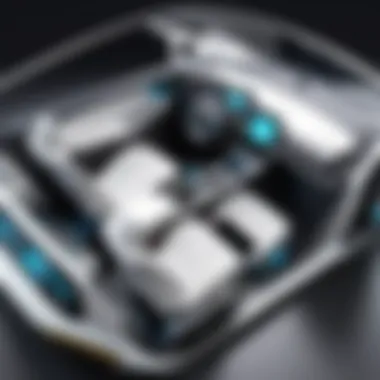
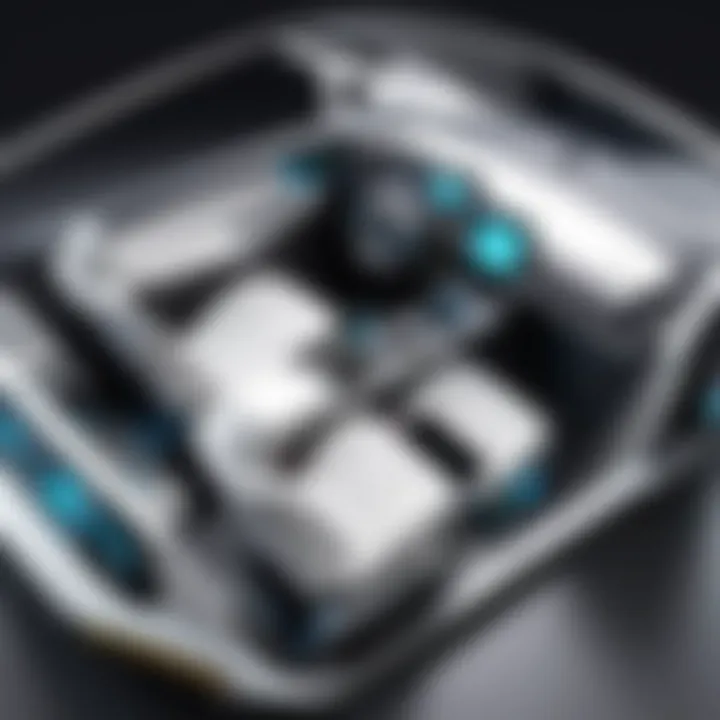
Intro
As we stand on the cusp of a new era in transportation, understanding the mechanisms that power autonomous driving becomes imperative. This technology is not merely about replacing the human driver with a machine; it’s a complex ballet of sensors, algorithms, and vast pools of data working in harmony. The world of self-driving vehicles is rapidly evolving, and grasping its intricacies is essential for a myriad of stakeholders—from tech enthusiasts to policy makers and everyday commuters.
The labyrinthine systems behind autonomous vehicles integrate sophisticated technologies that allow them to perceive their environment, make decisions, and navigate safely. Whether you’re an avid follower of tech trends or simply curious about what’s at the heart of automated driving, this exploration will delve into various components that make up this revolutionary mode of transport.
As we unfold this narrative, we’ll highlight how these systems converge to create vehicles that are not only capable but also safe enough for public use. Furthermore, the conversation will extend to the challenges that have yet to be overcome, as well as the developments we can anticipate in the near future. This is not just a technical discussion but a facet of societal change, affecting how we think about, interact with, and utilize transportation.
As we kick off this journey into the mechanics of autonomous driving, let’s dive into the technological insights that shape this fascinating field.
The Evolution of Autonomous Driving Technology
The journey of autonomous driving technology is nothing short of remarkable, a tapestry woven through decades of aspiration, innovation, and incremental advancements. Understanding this evolution is crucial, as it sets the stage for comprehending the current mechanisms at play and the promising future ahead. Each rustle of history offers nuances that shape today’s automotive landscape, presenting lessons in trials, triumphs, and transformative technological strides.
Historical Overview
The historical aspect of autonomous driving spans back to the mid-20th century. While the concept of driverless cars might seem like a recent marvel, the seeds of this idea were planted long ago. In the 1920s, a small leap of imagination resulted in the first self-driving vehicle prototype developed by a Belgian engineer named Jules Allard. This was essentially an electric carriage devoid of human control.
Fast forward to the 1980s, where the real momentum began. The Carnegie Mellon University began to see research focusing on robotics and autonomous navigation. At this point, the term "autonomous" began to be recognized, as projects like Navlab and ALV established foundational principles for self-driving technologies. Yet, it was still a realm of theoretical exploration without practical application.
By the late 20th century, external factors, like advances in computing and sensor technologies, ignited a flame in this narrative. The emergence of the internet allowed for significant data exchange that enriched the algorithms that would govern these future vehicles. These advancements transformed theoretical possibilities into tangible aspirations.
Milestones in Development
A coherent overview of milestones reveals not just advancements but pivotal moments that redirected the trajectory of autonomous driving technology. Here are some key milestones that need highlighting:
- 1995: The first high-profile autonomous vehicle demonstration occurred when the CMU's Navlab achieved a 98-mile trek.
- 2004: The DARPA Grand Challenge captured the world's attention. It challenged universities to develop autonomous vehicles capable of traversing a rugged course, igniting competition and collaboration.
- 2010: Google transformed the landscape by announcing its self-driving car experiments, focusing on overcoming congestion and enhancing road safety through technology.
- 2015: The advent of advanced driver-assistance systems (ADAS) began to surface, offering a practical blend between human control and automated assistance.
- 2020: Major automotive manufacturers like Tesla introduced features like Full Self-Driving Beta, bringing autonomous technology into everyday consumer markets.
These milestones underscore essential teachings, each contributing layers of understanding essential for navigating the future of mobility. The evolution of autonomous driving technology reflects a convergence of humans’ ambition, rigorous research, and groundbreaking advancements, a journey that continues to unfold.
Core Technologies Enabling Autonomy
The emergence of autonomous driving technology hinges on a suite of cutting-edge core technologies. They serve as the backbone of self-driving systems, enabling vehicles to interpret, decide, and act in complex environments with minimal human oversight. Grasping how these technologies work in tandem is crucial for understanding both the capabilities and limitations of autonomous vehicles.
Sensor Systems
In any autonomous driving setup, sensor systems are the vanguard. They act as the vehicle's eyes and ears, gathering vital environmental data that informs navigation and decision-making processes.
LiDAR Technology
LiDAR, which stands for Light Detection and Ranging, is a key player in the sensor realm. This technology uses laser pulses to measure distances between the sensor and surrounding objects, creating precise 3D maps of the environment. Its hallmark characteristic lies in its ability to work in diverse conditions, allowing it to function not just in daylight, but also at night or in adverse weather.
One standout feature of LiDAR is its accuracy; the technology produces detailed point clouds that effectively capture fine details in the surroundings. This level of detail is essential for pinpointing obstacles such as pedestrians or other vehicles. However, one downside is its relatively high cost, which continues to pose a challenge for widespread adoption in more budget-friendly automotive options.
Radar Systems
Radar systems operate differently, using radio waves to detect the position and speed of objects. The pivotal aspect of radar technology is its robustness against weather conditions; it can perform reliably regardless of fog, rain, or snow. This makes it invaluable in the ever-changing conditions of real-world driving.
The unique feature of radar is its superior range compared to optical systems like cameras and LiDAR, enabling detection of objects far down the road. On the flip side, radar might struggle with fine detail, such as distinguishing between different types of objects, which could limit its effectiveness in some scenarios.
Camera Systems
Camera systems round out the trio of primary sensors, bringing a visual component that is rich in detail and color. They are particularly advantageous for tasks like reading road signs or traffic lights, which require high-resolution imaging. The most appealing quality of cameras is their affordability and easy integration into existing vehicle designs.
However, cameras have their own set of constraints. They are highly dependent on lighting conditions and can be impaired by glare or heavy rain, which can hinder their effectiveness. Yet, when employed together with LiDAR and radar, cameras provide a comprehensive understanding of the vehicle's surroundings.
Computational Architecture
The computational architecture of autonomous vehicles comprises the processing units and data fusion techniques that synthesize sensor information into actionable insights. This architecture is the heart of any autonomous driving system, dictating how efficiently and effectively data is processed.
Processing Units
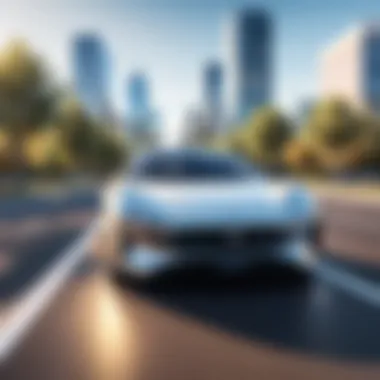
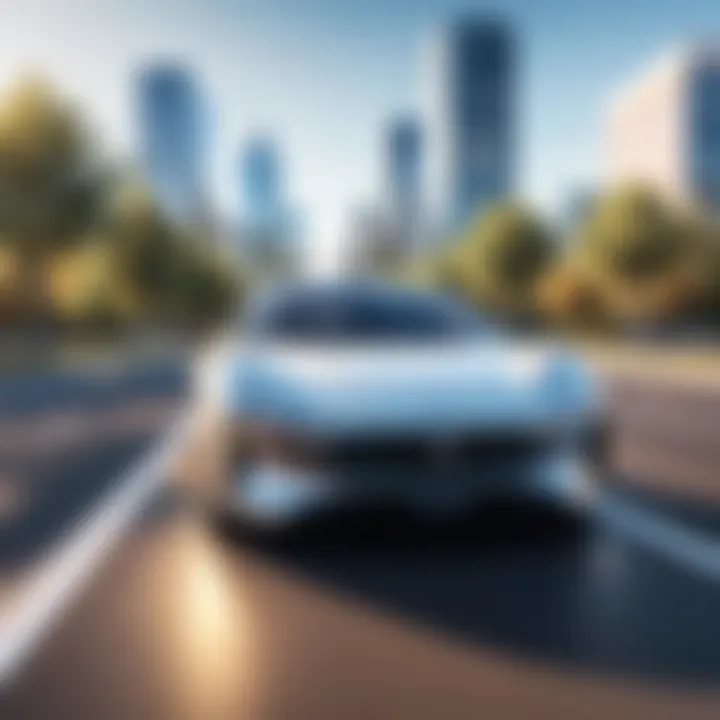
Processing units are akin to a vehicle's brain; they execute the myriad computations necessary for real-time decision-making. The key feature here is speed—drawing from powerful processors and GPUs, these units must analyze data from multiple sensors instantaneously.
Their unique advantage is the ability to handle complex algorithms and rapid data input, which are essential for safe navigation in dynamic environments. A downside, however, can be the substantial power consumption which may limit vehicle range or necessitate advanced thermal management.
Data Fusion Techniques
Data fusion techniques play a crucial role in merging data from various sensors to create a comprehensive understanding of the environment. This integration involves sophisticated algorithms that interpret overlapping data streams, allowing the system to make informed decisions.
The standout feature of data fusion is its ability to compensate for the weaknesses of individual sensors by taking a holistic view of the operational context. Nonetheless, the complexity of the algorithms and the computing power required can present significant technical challenges in the design of these systems.
Software Algorithms
Lastly, software algorithms are essential for transforming sensor data into meaningful action. They guide the vehicle in making real-time decisions, ensuring it navigates safely and efficiently.
Machine Learning Models
Machine learning models are foundational in enabling an autonomous vehicle to adapt and improve over time. These models are trained on vast datasets to recognize and predict a variety of scenarios, directly contributing to the vehicle's decision-making process. The key characteristic that makes them popular is their ability to learn from experience, refining their algorithms based on new data.
One notable feature is their capacity for recognizing subtle patterns in driving scenarios, which can enhance the vehicle's behavior in unpredictable situations. However, they can require significant data to train effectively, and there exists a risk of overfitting if not managed properly.
Path Planning Algorithms
Path planning algorithms are what help vehicles determine the safest route from point A to point B. They take into account current road conditions, traffic, obstacles, and even potential hazards. The critical aspect of these algorithms is their ability to make real-time adjustments based on new data.
A unique feature of path planning algorithms is their integration capability with other systems, allowing for dynamic route modification in response to unexpected changes like an accident ahead. On the downside, this can sometimes lead to computational delays if the algorithms are overly complex.
Data Management in Autonomous Systems
In the realm of autonomous driving, data management stands out as a critical backbone. This intricate network of collection, storage, and real-time processing ensures that self-driving vehicles function seamlessly. When these systems gather, analyze, and act on data, the effectiveness and efficiency of autonomous operations are greatly enhanced.
Data management helps ensure that the massive volumes of information generated are used wisely. It's like how a chef organizes different ingredients before whipping up a gourmet meal—the right data, at the right place, and at the right time makes all the difference in how the vehicle navigates its environment.
Data Collection and Storage
At the heart of autonomous driving is data collection, which is carried out through various sensors embedded in the vehicle. Think of it as the vehicle's senses, constantly on the lookout for vehicles, pedestrians, road signs, and even variations in terrain. Common data sources include:
- LiDAR: Provides highly precise 3D mapping of surroundings.
- Cameras: Capture visual information essential for recognizing and understanding the environment.
- Radar: Operates effectively in all weather conditions, detecting objects and their speed.
Once gathered, this data needs to be stored efficiently for both immediate use and future analysis. Usually, it’s stored in multi-layered systems that ensure rapid access while maintaining integrity. Cloud storage, for instance, is often employed for larger datasets, allowing easy access for updates and enhancements.
This data storage facility serves multiple purposes:
- Historical Analysis: Identifying patterns in previous trips to improve decisions.
- Model Training: Feeding algorithms with data to refine their accuracy.
- Safety Reviews: Retaining records of incidents or near-misses to boost safety protocols.
Maintaining data integrity through strict security measures is paramount to prevent unauthorized access or breaches.
Real-Time Processing Needs
The name of the game in autonomous driving systems is real-time processing. Vehicles need to interpret the data they collect almost instantaneously. Any lag or delay can lead to catastrophic outcomes. Consider driving in busy traffic; the vehicle must analyze data as it comes in, much like a human making split-second decisions based on the flow of information from their senses.
Key processing requirements include:
- Latency reduction: The goal is to minimize the time from data collection to action. Vehicles constantly scan and adapt their route and decisions.
- Data fusion: Combining data from different sensors creates a cohesive understanding of the vehicle's environment. This fusion technology ensures that no single data source is relied upon solely, making the system much more robust.
- Adaptive algorithms: The software needs to adjust not just to static environments but also to dynamic conditions, such as moving objects or changing weather.
Overall, realizing these processing needs requires a sophisticated computational architecture. As autonomous vehicles become more complex, so do the demands placed on their data management processes.
"Without effective data management, the future of autonomous driving would be not just unpredictable but perilous."
Ultimately, ensuring that data is collected, stored, and processed in a timely manner is not merely about technology; it's about building trust in a system that holds the potential to reshape how society navigates the world.
Safety Protocols and Regulations
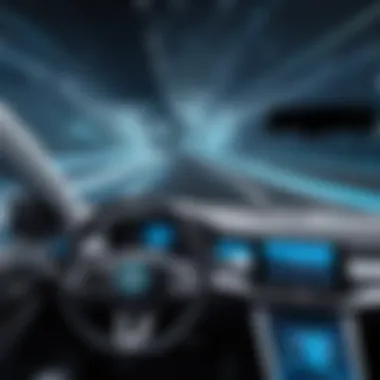
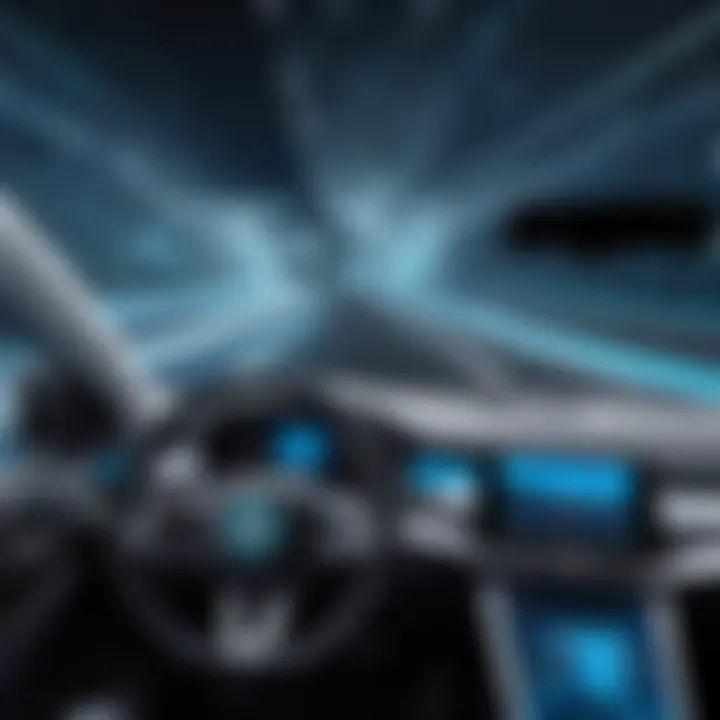
Safety protocols and regulations serve as the backbone of autonomous driving technology. As vehicles transition from human-operated systems to autonomous ones, the importance of stringent safety measures cannot be overstated. These regulations are pivotal not only for the safety of passengers but also for pedestrians and other drivers on the road. They create a framework that ensures vehicles can operate in a predictable and secure manner while minimizing risks associated with malfunction or human error.
Understanding the mechanisms of safety protocols is critical for manufacturers and policymakers alike. It involves a careful examination of several essential elements:
- Standardization of Testing: Developing uniform testing procedures ensures that every autonomous vehicle meets established safety benchmarks before hitting the road.
- Liability Determination: In incidents involving autonomous vehicles, it must be clear who is responsible—whether it is the manufacturer, software provider, or vehicle owner.
- Public Trust: Through rigorous regulation and transparent safety records, consumer confidence can be bolstered, encouraging widespread acceptance of autonomous vehicles.
Testing Standards
At the core of ensuring safety in autonomous driving are solid testing standards. These standards dictate how vehicles are evaluated before they can be deemed safe for public usage. Different test scenarios must be created to simulate a multitude of driving conditions.
For example, vehicles must be tested in varied environments:
- Urban streets with complex traffic patterns
- Rural areas with less predictable variables, such as wildlife
- Nighttime and adverse weather conditions like rain or snow
Moreover, testing should not be a one-and-done situation. Continuous updates to the software and hardware in these vehicles necessitate ongoing evaluations to adapt to new challenges and security concerns that may arise. Adopting a policy of iterative testing helps identify areas that require improvement, enhancing overall safety.
Legal Frameworks
The legal frameworks surrounding autonomous vehicles are still very much in development. Governments are faced with the task of crafting laws that govern the operation of these vehicles while addressing various public safety concerns. Key components of these legal structures include:
- Registration and Licensing: Similar to traditional vehicles, autonomous vehicles must be registered. How this is done for vehicles without a human driver is still under consideration.
- Insurance Regulations: Insurance policies need to adapt to accommodate situations involving autonomous vehicles, particularly in defining coverage in accident scenarios.
- Data Privacy Laws: As autonomous vehicles collect vast amounts of data, it is vital to put laws in place that protect consumer privacy, making sure individual data is kept secure and used ethically.
As society gradually shifts toward the acceptance of self-driving vehicles, the intersection between safety protocols and legal regulations will be crucial in fostering a safe and responsible environment for all road users. The intricate balance of these elements supports the overall aim of autonomous driving technology: to provide a safe, efficient, and trustworthy transportation future.
Challenges Facing Autonomous Vehicles
The journey towards fully autonomous vehicles is anything but smooth. There are numerous challenges facing autonomous vehicles that need to be ironed out for broad adoption. Understanding these difficulties allows us to appreciate the complexities of crafting reliable self-driving technology. It goes beyond the simple idea of a car that drives itself; instead, it encompasses a wide array of technical, ethical, and societal considerations.
Technical Limitations
First and foremost, the technical limitations of autonomous vehicles are significant. The technology must address various issues but isn’t quite there yet. For instance,
- Sensor Reliability: Sensor systems like LiDAR, cameras, and radar have limitations in certain environments, like heavy rain or fog. Imagine driving in a torrential downpour where visibility is next to zero; sensors can struggle to provide the necessary data.
- Complex Environments: Urban settings present a unique challenge with pedestrians constantly moving about, unpredictable cyclists weaving through traffic, and construction zones cropping up out of nowhere. These scenarios demand an agile response from the vehicle's algorithms. The ability of self-driving cars to navigate these complexities is still a work in progress.
- System Integration: Even when individual components work well, the integration of these systems can lead to hiccups. If your car's sensors are sending conflicting messages, which one do you trust? This internal commotion can affect performance and safety.
While companies are continuously improving technologies, the reality is that fully autonomous vehicles face frustrating hurdles. The technology is not yet foolproof, and public trust hangs in the balance.
Ethical Considerations
Ethical considerations in autonomous driving warrant serious attention. These vehicles will invariably operate in environments filled with other humans, each with their own set of choices and actions. These situations present moral dilemmas that current technology cannot navigate effectively. A few key issues that arise include:
- Decision-Making in Crises: Imagine a scenario where the vehicle must make a split-second decision in an unavoidable accident. Will it favor the occupants at the expense of pedestrians? Such scenarios raise profound ethical questions that remain unresolved in the design and programming of self-driving cars.
- Accountability: When an autonomous vehicle is involved in an accident, who holds the blame? Is it the manufacturer, programmer, or possibly the car itself? This ambiguity complicates liability laws and acceptance of technology in society.
"The evolution of legislation surrounding autonomous vehicles could take decades as we attempt to decipher these ethical quandaries."
- Public Perception: The acceptance of self-driving technology hinges on ethics as well. If the public feels that the vehicles could potentially jeopardize lives, skepticism will linger. Companies need to earn the trust of their consumers, and transparency in how these vehicles operate could play a crucial role.
Ultimately, overcoming these ethical challenges is essential for the widespread adoption of autonomous vehicles. The tech-savvy individuals, gadget lovers, and early adopters of technology may see the promise in these innovations, but they are also aware of the implications surrounding this technology. As the details become clearer, society will have to engage in a much wider discourse about the pathway ahead.
Future Directions in Autonomous Driving
As the landscape of transportation continues to shift, the future directions in autonomous driving hold significant importance. This chapter sheds light on innovative approaches that not only enhance the technology but also influence how we interact with our urban environment. The drive toward a more interconnected and sustainable transport system is becoming a prevalent focus for both developers and city planners. Technology plays a critical role in shaping how we view mobility in the years to come.
Integration with Smart Cities
The concept of smart cities provides a fertile ground for the advancements in autonomous driving systems. Here, technology and infrastructure blend seamlessly. This integration facilitates smoother communication between vehicles and their urban surroundings, leading to efficient transportation networks. By utilizing real-time data, vehicles can better navigate through traffic, optimize routes, and minimize congestion.
- Collaboration among Systems: Smart traffic signals, weather monitoring systems, and local infrastructure can communicate vital data. For instance, traffic lights might adjust their timing based on the flow of autonomous vehicles, leading to improved travel times.
- Energy Efficiency: With a growing concern for environmental impact, vehicles can connect with the energy grid to optimize energy use. This may include charging during off-peak hours or utilizing renewable energy sources.
- Safety Enhancements: Through integration, potential hazards such as road construction or accidents can be communicated to vehicles even before the driver sees them, increase the overall safety of the driving experience.
Integrating autonomous vehicles within smart cities means not just having a self-driving car, but using them to serve larger societal needs. This cooperation extends beyond personal transportation; it can revolutionize public transport as well by synchronizing bus schedules with traffic flows to ensure timely arrivals and departures.
Advancements in AI
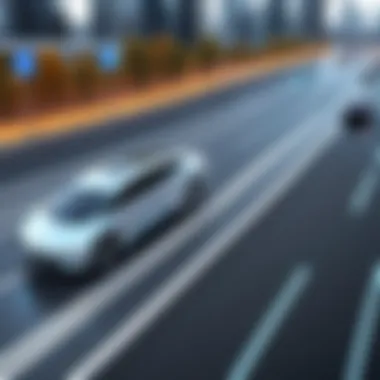
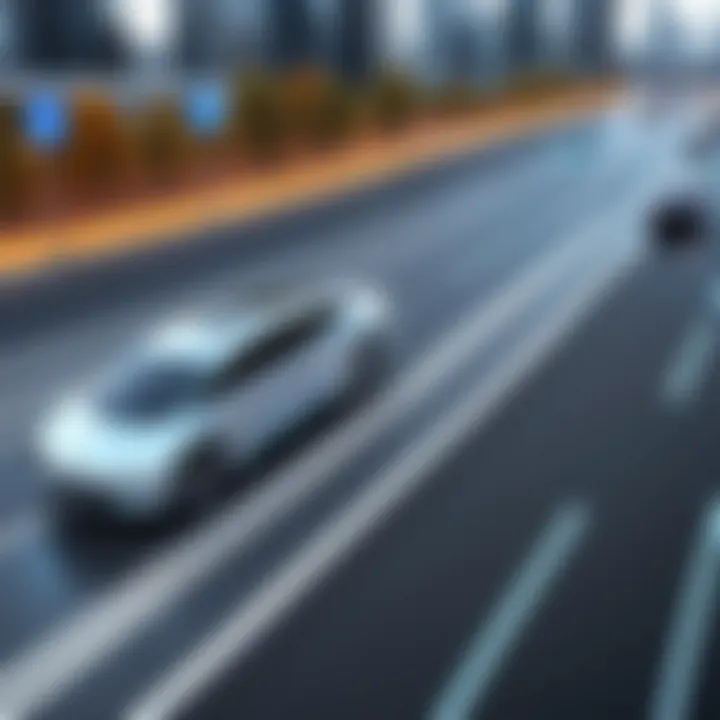
Artificial Intelligence is at the heart of what makes autonomous driving a reality. As AI technology advances, we expect to see remarkable enhancements in driving capabilities, enabling vehicles to adapt to more complex scenarios. The crux of this technology lies in its ability to learn and grow, becoming increasingly proficient in making decisions.
- Deep Learning and Neural Networks: These advancements allow vehicles to better recognize patterns in their environment. For example, through deep learning algorithms, an autonomous vehicle can improve its understanding of pedestrian movement, ensuring it responds accurately in real-time situations.
- Predictive Analytics: AI incorporates predictive modeling, allowing vehicles to foresee potential incidents based on historical data. If it knows that a particular intersection usually experiences heavy foot traffic at a certain time, it could slow down or alter its route accordingly.
- Vehicle-to-Everything (V2X) Communication: This technology allows cars to communicate with other vehicles, infrastructure, and even pedestrians. For instance, if a car ahead suddenly applies brakes, the AI system can prompt the following vehicles to react almost instantaneously.
“The future of autonomous driving is not just about replacing human drivers; it’s about integrating vehicles into a smarter, more sustainable urban ecosystem.”
In summary, as we look to the horizon of transport development, the integration of autonomous vehicles into smart cities and advancements in AI will shape the future of mobility. The synergy between these two elements promises a revolutionary approach to urban life, where safety, efficiency, and sustainability take precedence.
The Impact of Autonomous Driving on Society
The impact of autonomous driving technology stretches beyond the realms of engineering and innovation; it touches the very fibers of society. This section examines how self-driving vehicles are set to reframe various aspects of our daily lives, from economic structures to urban living. As we delve into these influences, it becomes evident that the transition towards autonomous driving is more than a mere advancement in automotive technology—it's a significant societal shift.
Economic Implications
The introduction of autonomous vehicles (AVs) brings with it a myriad of economic considerations. First off, there's the potential for job displacement in sectors such as trucking, taxi services, and delivery. Many laborers who currently rely on driving jobs may find themselves needing to adapt to a changing job landscape. But it ain't all doom and gloom; new jobs could emerge in technology development, maintenance, and oversight of these autonomous systems.
"The true cost of AVs isn’t just how much they save on labor; it's the innovation they trigger across multiple sectors."
Additionally, the economics of transportation could shift dramatically. With reduced need for personal vehicle ownership due to ride-sharing capabilities of AVs, families might opt to forgo traditional car expenses, driving demand for car manufacturers to reconsider their business models. This could lead to a boom in service-based economic models, pivoting from product ownership to vehicle usage based models.
Consider also insurance premiums. If AVs can demonstrate a lower accident rate due to their sophisticated sensors and algorithms, we might see a plunging cost for insurance policies, benefiting consumers while simultaneously challenging traditional insurance paradigms.
- Impacts on Business Models:
- Shift from ownership to sharing.
- Rise of subscription models for mobility.
- Increased demand for in-vehicle services like entertainment and connectivity.
Changes in Urban Mobility
Autonomous vehicles have the potential to revolutionize urban mobility as we know it. One immediate change is the possibility of reducing traffic congestion. With smart coordination and communication among vehicles, AVs can optimize routes and manage traffic flow, potentially leading to fewer bottlenecks in city centers.
Urban design itself might see a facelift. As parking demands alter, city planners could repurpose parking spaces for more green areas or community spaces instead of just parking lots. Cities may become more pedestrian-friendly as the reliance on owning personal vehicles diminishes.
Effective management of urban spaces could also lead to better public transit integration with autonomous technologies. Imagine bus systems that adapt schedules in real-time or shared autonomous shuttles enhancing transport networks.
The implications go further; a reduction in vehicles might lead to lower emissions and improved air quality, aligning urban mobility with environmental goals.
- Potential Transformations:
- Reduction in vehicle ownership.
- Enhanced pedestrian zones.
- Integration between public transport and AVs.
In summary, autonomous driving technology is poised to reshape the fabric of society, influencing economies and urban environments alike. As we take this journey into a future intertwined with self-driving vehicles, the challenges and fluffs of adapting to these changes will require careful navigation, innovation, and essential discussions across various sectors.
Ending: The Road Ahead
As we reflect on the journey through autonomous driving technology, it's clear that we are on the cusp of a transportation revolution. The current advancements indicate not just the promise held within self-driving systems but also the profound implications for society and economics. Understanding where we stand today and where we are heading is essential for tech enthusiasts and early adopters alike, as the road ahead is both filled with opportunities and challenges.
Summarizing Key Insights
To comprehend the future, one must grasp the threads of innovation knitting the fabric of autonomous driving. Key insights include:
- Technological Synergy: The combination of sensors, algorithms, and data management creates a robust system capable of navigating our complex environments.
- Safety and Regulations: Establishing stringent safety protocols is paramount. Without proper regulations, the scaling of autonomous vehicles cannot proceed.
- Community Transformation: Autonomous vehicles are set to reshape urban landscapes, reducing congestion and rethinking public transportation. This alteration needs careful planning to ensure it benefits society as a whole.
These insights reveal that the journey toward full autonomy is multifaceted, requiring collaboration across technology, society, and legislation.
Looking Toward the Future
As we gaze into the horizon, exciting possibilities unfold. Here are several strong trends likely to shape the future of autonomous driving:
- Integration with Smart Cities: As cities grow smarter, autonomous vehicles will play a pivotal role in how we navigate urban spaces. Imagine cars seamlessly communicating with traffic signals, optimizing routes in real-time, and reducing energy consumption.
- Advancements in AI: The continuous evolution of artificial intelligence will refine machine learning models, making cars not just reactive but predictive. Understanding driver behavior and adapting to local conditions will become routine.
- Ethical Framework Development: As technology advances, discussions surrounding ethics must also progress. Establishing clear ethical guidelines about decision-making in life-threatening scenarios will become crucial.
Reflecting on these trends paints a picture of an integrated and harmonious future, where vehicles, cities, and human needs align. With dedication and careful planning, the full potential of autonomous driving can be realized, reshaping our world into one that promotes safety, efficiency, and sustainability.
"The journey of a thousand miles begins with one step" - Lao Tzu
In the grand scheme, everyone is part of this journey.
Related Resources
For those looking to dive deeper into the intricacies of autonomous tech, consider exploring resources like Wikipedia for broad overviews or discussions on platforms like Reddit to engage with communities passionate about the subject.