Understanding Data Washing: Techniques and Implications
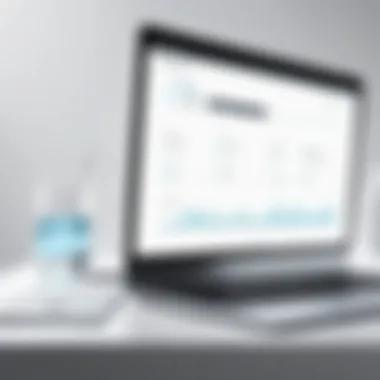
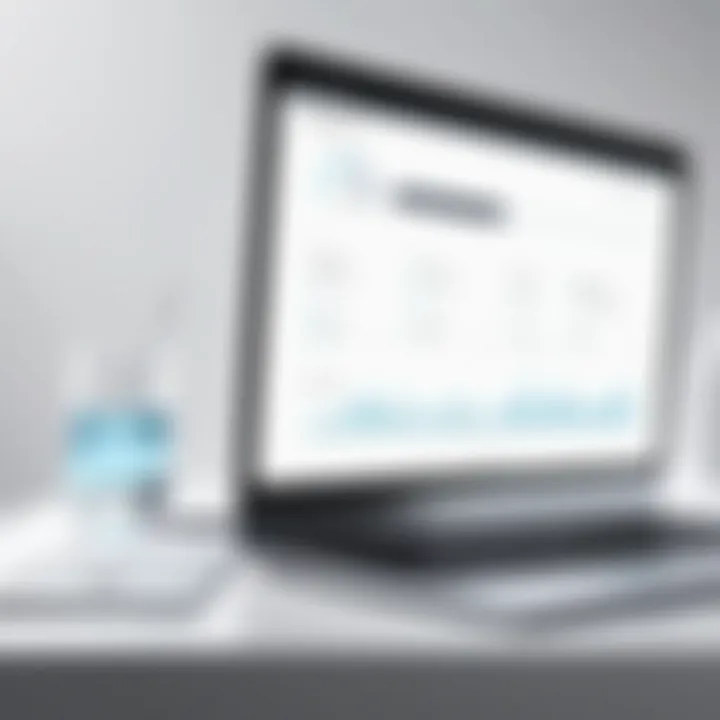
Intro
Data washing is a pivotal component of effective data management. It encapsulates methods that involve the cleaning, validating, and transforming of data to uphold its accuracy and reliability. In today's analytics-driven landscape, where data flows incessantly, scrutinizing the integrity of information becomes more important than ever.
Technology Insights
The Role of Technology in Data Washing
In a world overwhelmed by data, technology serves as the backbone of data washing practices. Various innovations have transformed how organizations approach data integrity. Technologies enabling automatic data auditing and updates allow faster and more efficient cleaning processes. Accordingly, businesses are shifting resources toward advanced data management technologies to minimize risks and improve operational reliability.
Latest Tech Trends
Recent trends in data management systems emphasize cloud solutions, artificial intelligence, and machine learning. These trends are reshaping data washing methodologies. Cloud-based platforms like Amazon Web Services and Google Cloud offer comprehensive solutions that allow firms to collaborate and streamline their data processes globally. Similarly, machine learning algorithms assist in identifying anomalies and inconsistencies in data sets, enabling more accurate assessments and timely interventions.
Innovation in Tech
Emerging tech solutions also play a critical role in developing better data washing techniques. For instance, the introduction of real-time data validation tools gives organizations the capability to address data inaccuracies immediately as they arise. This virtually eliminates common errors associated with manual updating and transformation. Tools like Apache Beam and Talend are worth mentioning. They enable automated workflows which lead to enhanced data credibility while conserving valuable resources.
Keywords Integrated
Understanding terms like data validation, data transformation, and real-time data analysis can improve knowledge within this domain, boosting SEO’s value.
Theframes of a Comprehensive Data Washing Strategy
Data washing is more essential now than ever, given the mounting pressures of an information-heavy environment.
Best Practices for Effective Data Washing
The significance of data washing is underscored by established best practices:
- Regular Audits: Conducting scheduled audits ensures data health.
- User Training: Provide proper training as user error often leads to data inefficiencies.
- Robust Systems: Implement strong data management systems that allow smooth data operational flows.
As data washing continues evolving, tech-savvy individuals and organizations must ensure they stay abreast with innovations, trends, and methodologies in order to enhance their operational strategies. Data credibility not only lies in having data but having reliable data, making exams of washing crucial for success.
Preamble to Data Washing
Data washing represents a foundational process in data management, fundamentally delineating steps aimed at creating high-quality and reliable datasets. Understanding data washing is critical, especially in a time where the flood of information poses serious risks to decision-making processes in various industries. This article will examine deeper implications of the data washing process, illustrating not just its components, but also its overarching importance in the accuracy and dependability of data that organizations source in today’s technologically driven environment.
Definition of Data Washing
Data washing can be defined as a systematic and continuous process through which organizations clean, validate, and transform their data sets. It involves techniques that provide assurance that data is fit for its intended use. Poorly maintained datasets can lead to inaccurate reporting, which in turn affects strategic decisions, operational efficiency, and ultimately business outcomes.
The data washing process typically includes:
- Data Cleaning: Removing duplicate records, correcting errors, and filling in missing values, ensuring data set integrity.
- Data Validation: Employing validation checks to ensure that data conforms to predetermined standards for quality and correctness.
- Data Transformation: Modifying and restructuring data into a format that is suited for analysis and reporting.
This can significantly enhance data integrity, making understanding the process vital for organizations operating in diverse fields, from health care to finance.
Historical Context of Data Washing
The practice of data washing has not always been mainstream. It has evolved over time, emerging alongside advancements in information systems, data technology, and the ever-increasing importance of data analytics. As early as the 1970s, organizations began to recognize that the introduction of technology would lead to broad data accumulation. However, the realization about the presence of data flaws emerged later.
In the following decades, as the volume of accessible data expanded through the rise of PCs and, subsequently, the internet, the importance of ensuring data quality became unavoidable. Early methods utilized basic statistical analysis, but intricate algorithms and automated tools have since developed to address the growing complexities within datasets. Institutional measures began to unfold, making data washing not just beneficial but necessary for effective data governance.
The Importance of Data Integrity

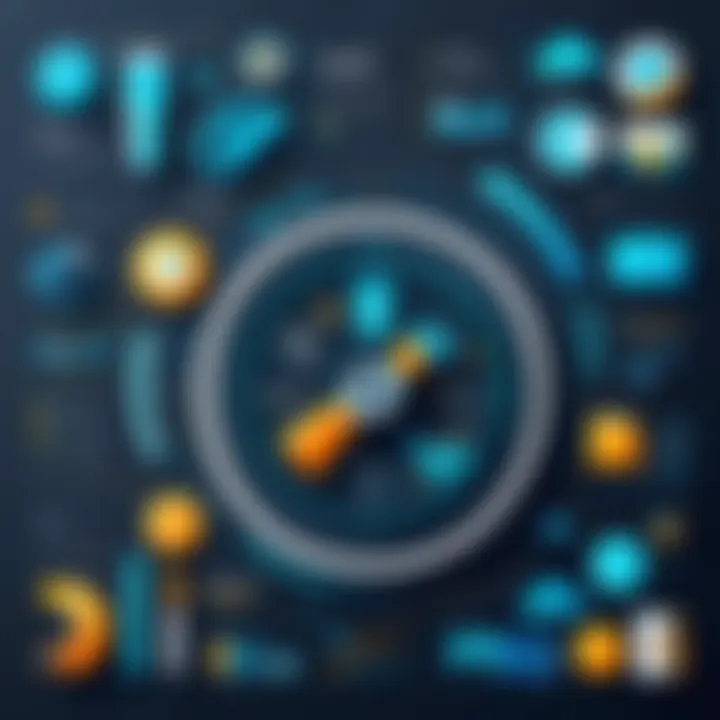
Data integrity is a fundamental concept in data management. It represents the accuracy and consistency of data throughout its lifecycle. Data integrity ensures reliability and trust in the data we depend on. This is critical for businesses, particularly in the realms of analytics and decision-making. Poor data integrity can result in misguided strategies and significant financial loss.
Implications of Poor Data Quality
Poor data quality can manifest in various forms, ranging from incorrect values to incomplete entries. The consequences of poor data quality can be severe. Issues can arise in many operational aspects, including:
- Misleading Analytics: If the data is inaccurate, the insights drawn from it will also be flawed. Companies may make decisions based on incorrect statistics.
- Financial Losses: An organization can incur hefty expenses due to incorrect data affecting customer service, supply chain management, or finance reporting.
- Brand Reputation Damage: Customers' trust may be eroded if they discover discrepancies or errors in their transactions. This can hurt long-term relationships.
Confronting these implications reveals the gravity of maintaining high standards of data integrity. Continuous efforts are required to manage and assess data quality effectively, demonstrating the essential nature of data washing.
How Data Washing Enhances Decision-Making
Data washing is integral to enhancing decision-making in various fields. By engaging in data washing, organizations can ensure they rely on clean and verified data. The benefits of data washing in this context are significant.
First, with clean datasets, organizations can perform accurate analyses. This clarity can lead to better insights and allows for forecasting that is more reliable.
Second, data washing prevents reactive decision-making. Businesses that address data quality proactively can position themselves to avoid jeopardizing opportunities because of faulty data. This strategic outlook fosters a more شوارع approach to competition.
Thirdly, efficient data washing encourages collaboration within organizations. It helps in creating a unified data source, allowing different departments to access and yield reliable information. From sales strategies to marketing approaches, enhanced data empowers teams to collaborate more effectively although multiple areas of the organization interface with the data.
Techniques for Effective Data Washing
Data washing is foundational in ensuring that the data used in analyses and decision-making is reliable. The techniques involved in effective data washing make it possible to clear inconsistencies and inaccuracies from info. It is essential for tech-savvy professionals aiming to utilize data for meaningful outcomes. Let's explore the specific elements involved.
Data Cleaning Methods
Data cleaning is the process of correcting or removing incorrect, corrupted, or irrelevant data from the dataset thus making it reliable for analysis. Effective cleaning methods can lead data scientist to accurate conclusions and permissible insights.
Common data cleaning methods include:
- Removing duplicates: Together with data inconsistency, replicates can skew analyses. Data analysis applies algorithms to find and eliminate these
- **Filling in missing values:**ستخدم الأطر البرمجية لوارو، مثل بايثون ور، لإدخال قيم فارغة.
- Standardizing formats: Data might come in different formats. It is important for dealing with the successful integration of several datasets. For instance, specific date formats should match for accurate calculations.
Data Validation Processes
Validation processes ensure that the data entered or imported meets the needed requirements. Checking mechanisms confirm that info fits scenarios for use and adheres to laws or standards. Failing to validate can lead to poor decision-making based on inaccurate data.
Some crucial data validation techniques include:
- Type Checks: Verify that the values entered behave in a reasoned format such as integers for ID’s and decimal numbers for financial records.
- Range Checks: Data must have territory limits, for example; a score must always lie between zero and hundred.
- Uniqueness Checks: Design food permits to validate no identical entries should exist whether numeric or string bases. This goes into a larger framework of avoiding datatype confusingness.
Data Transformation Techniques
Data transformation improves data quality affects all downstream processes. After cleaning and validation, the next stage is changing the data into a suitable format or structure suitable for analytics. Different techniques assist data HOA and data lake organization.
Noteworthy transformation techniques include:
- Aggregation: Combining multiple data points or records to enable easier analysis without losing valuable insights from base data.
- Normalization: Scaling different data fields, providing weighted models before asses changes in sense of transformation.
- Encoding categorical variables: Standardization of text data to numeric forms suitable for machine learning operations, thus facilitating data readability and usability.
Effective techniques for data washing unveil clarity from convoluted datasets, enabling informed decision-making amidst ever-expanding volumes of generated data.
Tools for Data Washing
Data washing relies heavily on the right tools to maintain the integrity of information. The process requires software that effectively cleans, validates, and transforms data. Selecting the appropriate tools can dictate the success of data initiatives within an organization. Thus, understanding these tools is essential.
Popular Data Washing Software
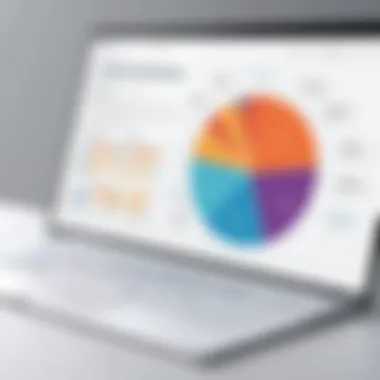
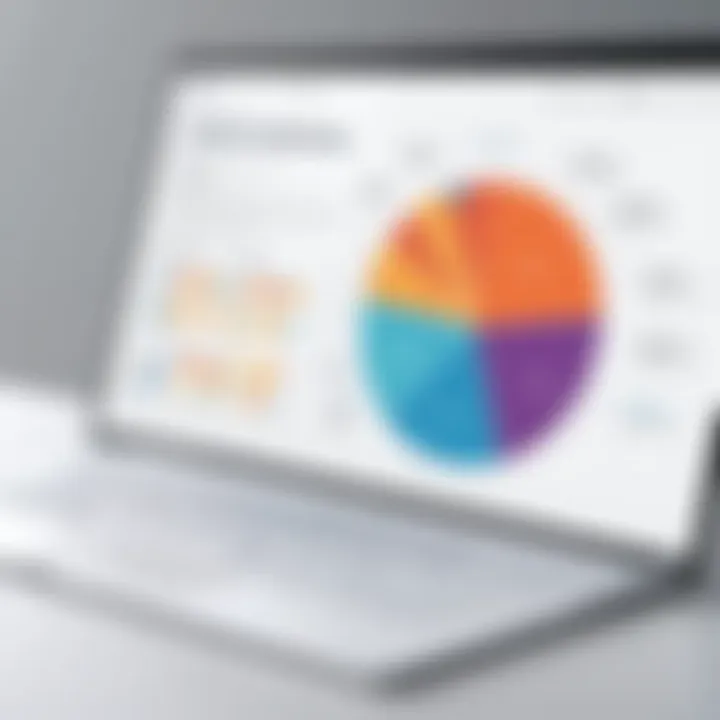
Several software options designed for data washing minimize the manual effort needed in data cleaning. Notable among them are:
- Talend: An open-source data integration tool that allows for routine data cleaning procedures.
- Trifacta: This tool provides a user-friendly interface for data wrangling and shaping.
- OpenRefine: Mainly supports cleaning messy data sets, it breaks down data for easier manipulation.
- WinPure: Focuses on data deduplication and cleaning, particularly for contact databases.
- SAS Data Quality: While more used in larger enterprises, this offers a spectrum of features encompassing data clean-up and governance techniques.
These tools perform functions like identifying duplicates, correcting spelling errors, and validating formats. User requirements differ, thus due diligence in selecting software substantiates decision-making.
Evaluating Tools for Your Needs
Assessing the right tool entails identifying organizational goals and specific data needs. The following factors should guide the evaluation:
- Data Source Compatibility: Ensure the tool works seamlessly with your databases, cloud storage solutions, or data warehouses.
- Ease of Use: A user-friendly interface supports faster adoption across teams, saving training time.
- Scalability: Choose tools that can expand with your data needs. As companies grow, their data requirements evolve. It's wise to invest in scalable solutions.
- Support and Community: Confirm the availability of support systems and user communities - troubleshooting and best practices can often be found through them.
- Cost-effectiveness: Analyze both upfront costs and ongoing expenses like maintenance and upgrades. Striking a balance between cost and functionality will lead to long-term satisfaction.
Challenges in Data Washing
Challenges in data washing are crucial because they affect the overall effectiveness of data management practices. Effective data washing enhances the quality, consistency, and reliability of data. However, various obstacles exist that can complicate this process. Here, we will explore common issues faced in data cleaning and look into data governance problems. Each of these aspects has significant implications for organizations that rely on accurate data for decision-making and operations.
Common Obstacles in Data Cleaning
Numerous obstacles can hinder the data washing process. These include:
- Data Volume: Large datasets make it difficult to identify quality issues promptly. Managing this data can require substantial computational power and time.
- Data Variety: Data comes from various sources and in numerous formats. Reconciling these differences while ensuring compatibility can pose a significant challenge.
- Incomplete Data: Often, collected data will be missing critical pieces, which compromises quality. Filling in these gaps without losing context or accuracy is a delicate task.
- Duplication: Duplicate entries are common, especially in systems that aggregate data from multiple sources. Identifying and resolving these redundancies can be labor-intensive.
Furthermore, organizations may not possess the best travel and navigation tools to target data anomalies. Even advanced systems have limitations, which creates significant risks of overlooking errors.
Data Governance Issues
Data governance is fundamental in establishing rules and protocols for using data effectively. However, poor data governance can lead to widespread issues in data washing. Here are some key hurdles:
- Lack of Clear Policies: Without guidelines on data handling, organizations may fail to clean and standardize data adequately, thereby maintaining dirt-quality information.
- Ownership Challenges: Unclear ownership rights over data create confusion. When responsibilities are not well-defined, tasks like cleaning and updating suffer setbacks.
- Compliance Regulations: Organizations often face stringent regulations regarding data storage and protection from entities like the GDPR in the EU. Complying with these regulations while enabling effective washing can be perplexing.
- Insufficient Training: Staff may need adequate training in data quality practices andtools. Without proper education on standards, cleaning efforts can be inconsistently applied.
Data washing keeps organizations from swimming in murky waters. It is critical to address common obstacles and data governance issues to ensure accuracy.
To manage these challenges effectively, organizations need a holistic approach tailored to their unique data ecosystem. Practical tools, robust policies, and informed personnel are paramount for achieving high standards in data handling.
Best Practices for Data Washing
Effective data washing requires careful planning, consistent execution, and a well-defined strategy. Best practices are critical in ensuring that data remains accurate, reliable, and ready for decision-making. The importance of these practices goes beyond mere compliance; they are foundational for building trust in data across organizations.
Establishing a Data Quality Framework
A robust data quality framework serves as the cornerstone of data washing. It includes various policies, measurements, and standards governing the data lifecycle. By establishing clear quality criteria, organizations can ensure data accuracy and completeness. Several key elements should be part of this framework:
- Defined Data Standards: Develop clear definitions for each data type relevant to your business processes. This clarity should include acceptable ranges, formats, and values.
- Metrics for Quality Assessment: Implement meaningful metrics that help measure data quality. Common metrics include accuracy, completeness, and consistency.
- Roles and Responsibilities: Clearly define who is responsible for managing data at various stages. This may involve data owners, data stewards, and system administrators.
Integrating these elements fosters accountability. It allows individuals to understand how they can contribute to maintaining data quality throughout its lifecycle.
Regular Audits and Maintenance
Even the best data can degrade over time without regular monitoring. Frequent audits are essential to identify issues early, ensure compliance with the established quality framework, and adapt practices to changing conditions. Regular maintenance activities would usually involve:
- Scheduled Reviews: Establish a systematic schedule for data quality assessments. Depending on data criticality, these reviews can occur monthly, quarterly, or annually.
- Addressing Data Issues: Develop an action plan to resolve issues identified during audits. This could mean correcting inconsistencies, updating missing values, or purging incorrect data.
- Feedback Loops: Create mechanisms to continually refine processes based on audit findings. Use input from diverse stakeholders to ensure the changes made facilitate greater data quality.
Organizations that commit to regular audits experience a tangible improvement in data reliability and decision-making efficacy.
Implementing these audit and maintenance practices ensures the data stays useful over time. This, in turn, minimizes risks associated with reliance on inaccurate or outdated information, thus solidifying the organization’s data credibility.
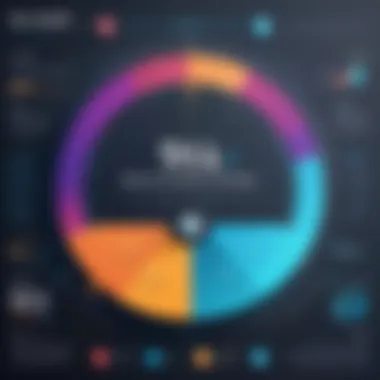
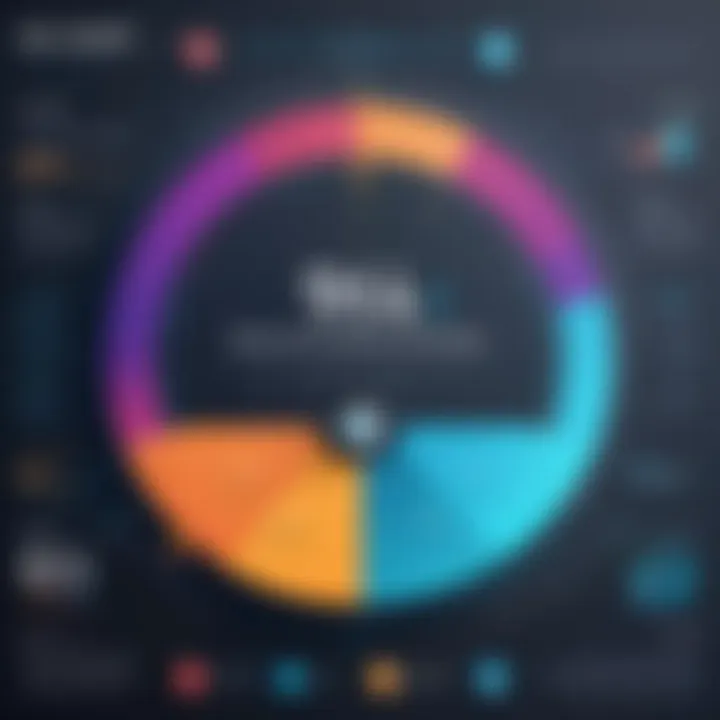
Case Studies of Data Washing
Case studies are an effective means to understand the implications of data washing in various industries. They provide real-world examples that illustrate how data washing techniques play a significant role in ensuring the accuracy and reliability of data. Analyzing specific case studies allows one to glean valuable insights into best practices, common pitfalls, and the potential benefits of rigorous data management processes. Furthermore, examining these cases across sectors, including finance and e-commerce, reveals how tailored data washing strategies can solve unique issues and optimize overall performance.
Examples from the Financial Sector
The financial sector serves as a prime example where data integrity is paramount. Financial institutions handle vast amounts of sensitive data daily, including transactions, investments, and customer information. An exemplified case involves ABC Bank, which faced severe accuracy issues in its transaction records. The data washing process identified duplicates and anomaly in entries, leading to incorrect account balances. Through rigorous cleaning, validation, and transformation techniques, ABC Bank managed to restore its data integrity.
The methods used in this case include:
- Automated data cleaning tools: These tools helped identify errors and duplicates efficiently.
- Regular audits: Continuous monitoring of data quality proved crucial in leaving no stone unturned.
- Collaboration with regulators and compliance teams: Ensuring alignment with upcoming market regulations required periodic data reviews.
Following the data washing, the bank recorded a 20% reduction in discrepancies. This resulted not only in enhanced customer trust but also yielded tangible savings by diminishing fraud incidents. Thus, effective data washing translates into better decision-making for financial entities and alllows sustained operational excellence.
Applications in E-Commerce
In the e-commerce domain, having accurate and reliable data is essential for maintaining customer loyalty, personalizing user experiences, and ultimately driving sales. An illustrative case involves XYZ Online Retailer, which was struck by high customer churn rates due to misleading product information and lengthy navigational bottlenecks on their site.
The retailer initiated a comprehensive data washing campaign using the following strategies:
- Data curation and enrichment: They simplified product listings and ensured detailed specifications, minimizing mismatches.
- User behavior tracking: Identifying patterns in customer navigation unveiled necessary changes sniped based on data insights.
- Feedback loops with customers: Engaging directly with users helped collect valuable input regarding data accuracy.
After implementation, XYZ Online Retailer experienced a boost in conversion rates by 30%. Customer satisfaction also improved as accurate product descriptions reduced return rates. This example concludes that regular and systematic data washing paves the way to innovation, not just preserving reliability but reshaping habitats to suit evolving customer preferences.
Effective case studies underline the operational relevance of data washing and showcase how different sectors can customize their approaches for maximum impact.
The Future of Data Washing
The future of data washing is poised to be shaped by rapid advancements in technology and changing data management paradigms. As organizations become increasingly reliant on data for decision-making, the integrity of that data will be paramount. Hence, understanding future trends and analyzing the growing role of artificial intelligence is essential for anyone dealing with data today.
Trends in Data Management
Data management is continuously evolving. Emerging trends highlight several key areas:
- Automation: Many tasks that involve data cleaning and validation are now being automated. Tools are integrating algorithms to detect anomalies and errors without human intervention.
- Cloud-based solutions: More companies move to cloud platforms for data storage. This shift allows them to use advanced data washing tools that are scalable and accessible from anywhere.
- Real-time data processing: The demand for instant analysis grows. Organizations seek solutions that enable real-time data washing, ensuring accuracy without delays.
- Data privacy regulations: With new legislation around data privacy, like GDPR, organizations must ensure their data washing practices comply with regulations. This adds a layer of complexity but drives standards for quality and security.
Monitoring these trends provides insight into how data washing practices must adapt. Companies need to stay vigilant to meet both internal and external needs. Organizations that embrace these changes will likely possess an edge in data-driven environments.
The Role of AI in Data Washing
Artificial intelligence will revolutionize the field of data washing. Machine learning algorithms are capable of identifying patterns that may not be visible to human analysts. Key contributions of AI include:
- Enhanced cleaning processes: Machine learning models can learn from datasets and create internal rules for identifying irregularities, speeding up the cleaning process significantly.
- Predictive analytics: AI systems can anticipate errors before they occur, which proactively maintains data quality.
- Advanced validation: AI tools validate data against multiple sources, increasing confidence in the results. This ability shifts the paradigm from reactive maintenance to proactive management.
- Scalability: AI applications can handle growing volumes of data, meaning businesses can wash data at unprecedented speeds and volumes, useful in dynamic environments.
The integration of AI in data washing represents not just a step forward but a leap toward sophisticated data management.
End
The conclusion of this article offers a critical synthesis of the key themes surrounding data washing. It highlights the significance of maintaining data quality as a strategic asset in a world reliant on accurate, reliable information. It serves as a reflection on the methods and practices crucial for effective data cleaning, validation, and transformation.
Summary of Key Points
The article outlined several pivotal aspects:
- Definition and Importance: Data washing is integral for ensuring the accuracy and reliability of data.
- Techniques Employed: Various methods like data cleaning, validation, and transformation were discussed.
- Tools and Software: The availability of systems dedicated for data washing enhances the capability to maintain high data integrity.
- Challenges Ahead: Questions of governance and compliance currently present obstacles in data management practices.
- Future Trends: Emerging trends highlight the increasing role of artificial intelligence in shaping data management methodologies.
Final Thoughts on Data Washing
Despite the apparent complexity of data washing, the benefits far outweigh the challenges. Quality data informs used of resources, guides strategic decisions, and fosters innovation in various sectors. As organizations increasingly depend upon quality information, the focus on implementing robust data washing practices is not merely a choice, but an imperative. To remain competitive and agile, adaptive and effective data management strategies must evolve continuously.
Effective data washing is, without doubt, foundational for sustaining trust in data-driven initiatives. It reflects organizations' ultimate commitment to achieving excellence amid the vast oceans of information that rise daily.
Maintaining high-quality data is indispensable in navigating today’s data-rich landscape.