Innovative Approaches to Flower Detection Technologies
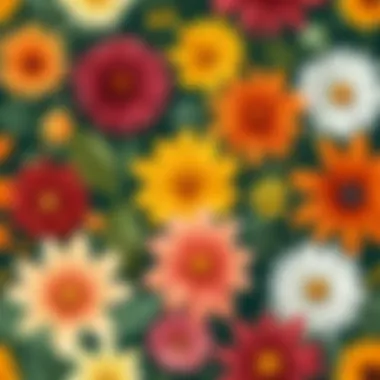
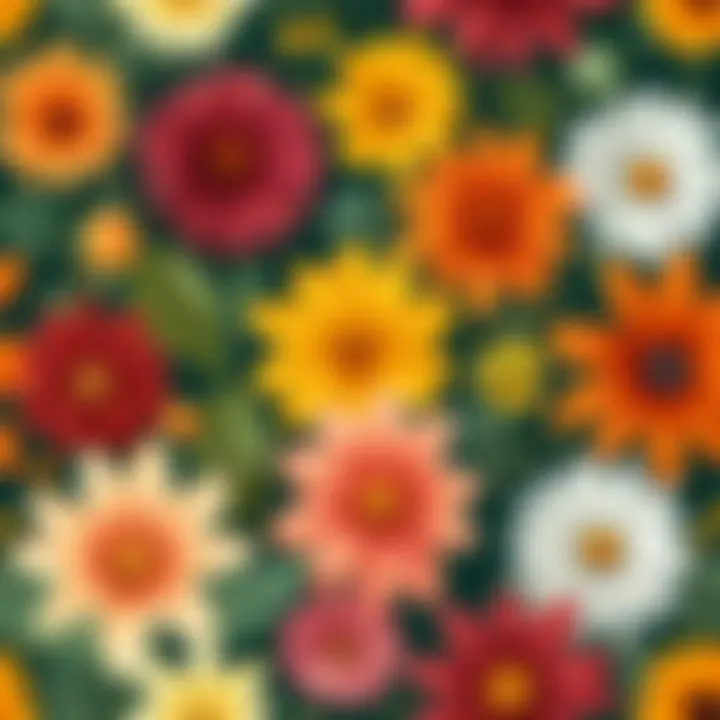
Intro
Flower detection is an area of growing significance. Understanding flora goes beyond just aesthetic appreciation. This procedure engages a number of benefits for agriculture, conservation, and biodiversity research. Today, advanced methodologies have come into play.
Technology Insights
Latest Tech Trends
Recent years have seen profound developments in the technology behind flower detection. Computer vision and image recognition represent two main branches of this evolution. Researchers employ advanced algorithms to enable systems to distinguish between various flower species accurately. Instead of images requiring extensive manual interpretation, artificial intelligence streamlines this process considerably. Additionally, drones equipped with cameras utilize high-resolution photographs to identify flowers in vast areas efficiently.
Innovation in Tech
Interesting innovations include deep learning techniques which grasp patterns within large sets of data. For instance, Convolutional Neural Networks (CNNs) demonstrate superior accuracy in morphological recognition. These innovative approaches often outperform earlier statistical models and encourage greater reliability in detecting flowers automatically. Furthermore, tools combining smartphone applications and AI assistance allow amateur botanists and nature enthusiasts to explore local flora with unprecedented ease.
Product Reviews
When discussing technology for flower detection, several top-tier products arise. Apps such as PlantSnap provide interactive capabilities for smartphone users. This application recognizes numerous species, maintaining a comprehensive database to ensure frequent updates. A more specialized alternative is Flora Incognita, aimed at delicate fieldwork, incorporating research-level precision methods to catalog flora.
Advancements in technology are shaping the landscape of flower detection by combining diverse methodologies and enhancing awareness of our natural environment.
Understanding the Importance of Flowers
It is crucial to comprehend why flower detection is essential. Flowers play a pivotal role in ecosystems—ensuring pollination, attracting pollinators, and maintaining the intricate food webs. The identification of plant species often implies a greater significance within the broader ecological context as well.
Conservation Efforts
Identifying and classifying flowers enables more informed conservation strategies. Close monitoring trends in flower species could signal ecosystem changes influenced by climate shifts. Thus, effective flower detection contributes to overall ecological preservation efforts.
Cultural Significance
Flowers also hold substantial cultural meaning. Many societies recognize flowers in customs, rituals, or as symbols of certain ideals. Understanding different flower species cultivates a richer appreciation for cultural displays linked to flora.
Maintaining insight into the state of native flowers teaches us much about our interconnected existence with the environment.
Future Developments in Flower Detection
This examination must also extend to assess future possibilities in flower detection technologies. Collaborations may enhance current methodologies, merging traditional knowledge with innovative practices.
Moving forward, interdisciplinary approaches will likely yield promising outcomes.
Monitoring technological updates, being aware of sustainability tips, and tapping into community-driven botanical databases can keep individuals informed on fresh avenues in flower identification and conservation.
In summation, the synthesis of technology and environment promises to elevate flower detection methodologies significantly, paving the way for both ecological benefit and cultural exploration.
Preamble to Flower Detection
Understanding methods for flower detection is increasingly relevant in many fields, from biodiversity research to agriculture. This introduction aims to present the critical importance of identifying flowering species, not only for ecological balance but also for cultural understanding. Proper identification acts as a catalyst for preserving species, which in turn enrich our ecosystem.
Importance of Flower Identification
Flora identification holds significance in various sectors:
- Biodiversity Preservation: Accurate counting of flowering species fosters conservation efforts. This is particularly crucial as habitats face constant disturbances.Grouping species can facilitate much smoother initiatives.
- Agricultural Optimization: Recognizing flowers enables targeted approaches to pollination. Farmers benefit from understanding which plant species thrive together, cultivating an environment supportive of pollinators like bees.
- Medicinal Research: Some flowers hold untapped medicinal properties. By identifying these flowers, potential for discovering new treatments increases.
HEIGHTENING the necessity for flower recognition requires integrating advanced methods and technologies. This process will pave ways for exploring various tools and insights shaping contemporary practices.
Overview of Current Technologies
The evolution of technology has significantly impacted flower detection. The following technologies play vital roles:
- Computer Vision: Utilizing specialized algorithms to interpret images and recognize flower species through distinct patterns and features.
- Machine Learning: Training models with extensive datasets maximizes accuracy in classification and prediction of flower identification.
- Mobile Applications: Apps like PlantNet and PictureThis enable immediate flower recognition through user-friendly interfaces, broadening accessibility.
- Remote Sensing: This technology gathers data from afar. Drones equipped with cameras offer researchers the ability to survey wide areas, gathering insights on flora distribution.
The intersection of technology and nature opens profound possibilities, connecting knowledge seekers with the natural world in dynamic, impactful ways.
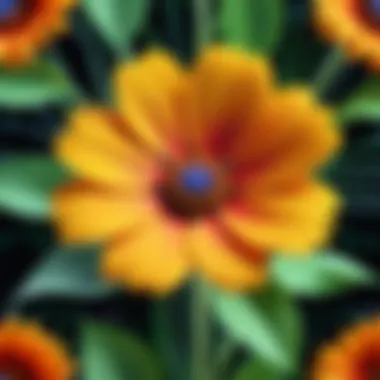
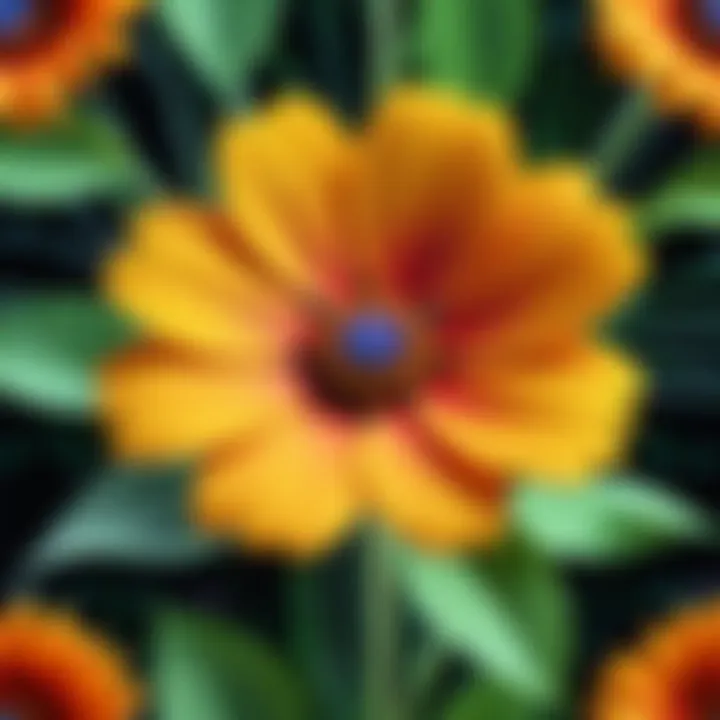
Implementing these technologies not just enhances data collection, but also serves ecological aims, making flower detection methods more efficient and insightful.
Historical Context of Flower Detection
Understanding the historical context of flower detection is crucial for appreciating its evolution and the factors influencing its current methodologies. The ability to identify flora has been integral to various fields, from agriculture to environmental science. By different generatons, this knowledge has not just helped in the recognition of species but also contributed to biodiversity conservation efforts and artisan traditions related to plants.
Traditional Methods of Identification
Historically, flower identification relied heavily on manual methods. Early flower classification happened through close observational skills. Botanists used field guides and identification keys based on morphological features such as the shape, color, and structure of flowers.
Many guides were developed over decades, detailing local flora to facilitate specific identifications. For example, researchers relied on visual cues, including leaf arrangements and petal counts, to determine flower species. As societies grew, this method was often complemented with traditional knowledge passed down through generations.
- Local Flora Guides: The development of printed guides rooted in geographical locations served to educate enthusiasts and professionals alike.
- Herbaria: Collecting dried plants became a standard. Herbaria play a key role in biodiversity, helping to catalog what species exists and track changes over time.
While these methods had significant merit, they were not without gaps. Basic technique mistakes could lead to misidentification. Variability in flowering traits influenced reliability. Such errors dealt detriments not only to academia but also to practical applications—such as ensuring the correct plants used in agriculture.
Evolution of Identification Techniques
Over the years, advances in technology heralded a shift away from traditional methods. Enhanced tribal identities were observed through DNA barcoding. With the advent of blueprints technology, botanical analysis gains precision.
The contributions from diverse fields reshape thematics around identification.
- Genetic Markers: DNA barcoding emerged as a key technique for precision and accuracy. Samples exhibiting morphological similarities but diverging at the genetic level can be distinguished effectively through samples from botanic gardens, scientific collections, and field work.
- GIS Technologies: Geographic Information Systems changed how we look at plant details in their environments. It provides context about habitat conditions that’s critical for both conservationists and scientists.
The transition from field guides to digital methodologies under powers forensic mission—yielding higher identifying accuracy that logic demands uneasy confidence.
Computer Vision in Flower Detection
The integration of computer vision into flower detection systems marks a crucial advancement in both ecology and technology. This technology allows for a remarkably precise identification of flower species by interpreting and analyzing visual data. Leveraging this ability generates numerous benefits, such as increased efficiency in biodiversity monitoring and enhanced understanding of ecosystems. Furthermore, it can significantly assist researchers and enthusiasts in horticulture, agriculture, and conservation. In the context of this article, it reveals the interplay between technology and nature, showcasing how emerging technologies can support traditional methodologies in identifying flora.
Functional Principles of Computer Vision
Understanding the underlying principles of computer vision is vital for grasping its application in flower detection. At its core, computer vision employs various algorithms that enable machines to interpret and make decisions based on visual information from the world. Images captured through cameras undergo rigorous processing to extract pertinent features.
Typically, functional principles can be outlined as follows:
- Image Acquisition: The process begins with the capture of images either through smartphones or dedicated devices. High-resolution images promote better feature extraction.
- Preprocessing: Images often undergo preprocessing steps to improve clarity and focus. This can include enhancing contrast, normalizing brightness, or applying filters to reduce noise.
- Feature Extraction: Machine learning models utilize techniques such as edge detection and segmentation to identify unique features in the image that align with known characteristics of certain flower species.
Such algorithms facilitate comparisons between a captured image and a library of images of previously classified species. As a result, computer vision systems can accurately classify a wide array of flowers based on those extracted features.
Image Processing Techniques
Various image processing techniques play a critical role in enhancing the quality of flower detection systems. Implementing the right approaches can lead to improved outcomes in classification accuracy. Below are key image processing techniques employed in this field:
- Histogram Equalization: This technique adjusts contrast using the distribution of intensity values, making subtle details more noticeable.
- Random Forests: A machine learning ensemble method that uses numerous decision trees to make predictions on image classification adds robustness to this process.
- Convolutional Neural Networks (CNNs): CNNs are a crucial subset of deep learning models tailored for image data. They identify patterns and features in layered hierarchies, thus providing more accurate flower detection.
When combined, these techniques create more nuanced image data that substantially strengthens the functionality of computer vision in flower detection.
The potential of computer vision lies in its ability to analyze vast data sets efficiently, identifying flowers with precision that manual identification may not achieve.
By embracing computer vision's capabilities within the realm of flower diagnosis and differentiation, we can drive effective conservation efforts and foster a deeper appreciation for biodiversity with minimal effort expended.
Machine Learning Algorithms
Machine learning algorithms play a crucial role in flower detection by streamlining the identification processes. Unlike traditional methods that relied heavily on manual observation and expertise, these algorithms can analyze large datasets effectively, identifying and classifying flower species quickly. The ability to learn from patterns and adapt makes machine learning a powerful tool for tackling floral diversity, allowing users to recognize various flower species based on their features, without extensive prior knowledge.
The key benefits of employing machine learning in flower detection include:
- Efficiency: Algorithms can evaluate photos far quicker than human experts.
- Scalability: Models can be trained on vast databases that include millions of flower images, enhancing accuracy.
- Automation: Reduces human bias and errors in identification, standardizing the process of detection.
- Continuous Learning: As new data becomes available, the algorithms can refine their accuracy.
In examining machine learning, the two primary approaches—supervised learning and unsupervised learning—are employed. Each provides unique advantages and capabilities valuable in floral study. Understanding these distinctions offers insights into effectively leveraging algorithms for identification.
Supervised Learning Approaches
Supervised learning approaches involve training a model on labeled datasets. Researchers and practitioners input examples of flowers that are already identified, which allows the model to learn specific features and patterns associated with each species.
A distinct feature of this approach is the ability to produce accurate predictions when the model encounters a new and unlabeled flower image. Here's how supervised learning contributes to flower detection:
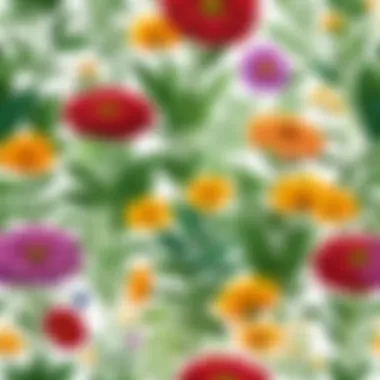
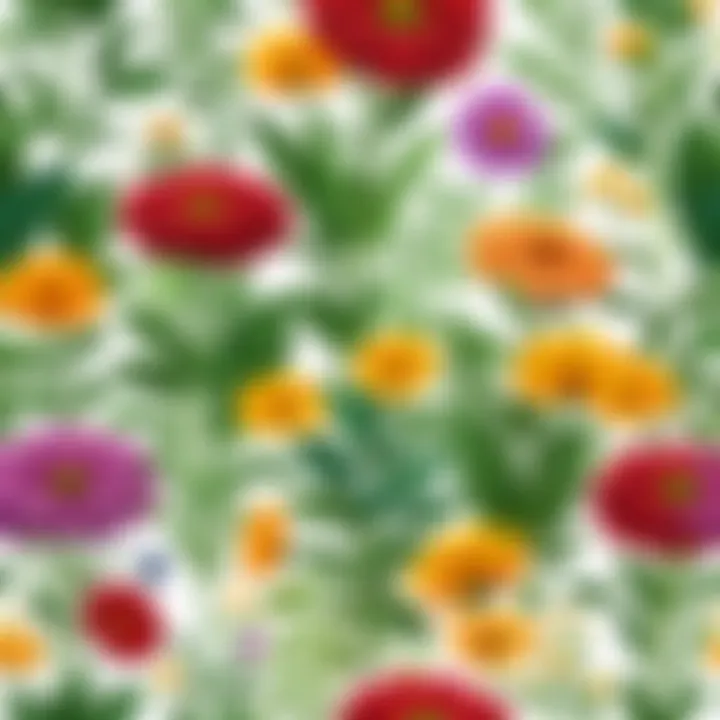
- Data Annotation: Each image must be annotated to help the model recognize different species.
- Training Phases: Significant amounts of data require multi-phase training where models adjust based on feedback.
- Accuracy Monitoring: Performance is often fine-tuned through rigorous testing and validation.
- Feature Highlighting: Algorithms focus on attributes like color, shape, and size to differentiate species effectively.
Among the popular methods used in supervised learning for plant identification, convolutional neural networks (CNNs) have gained prominence. CNNs excel in handling visual data and can automatically extract meaningful patterns from images.
Unsupervised Learning Applications
Unsupervised learning expands the possibilities of machine learning through its ability to analyze data without predefined labels. In flower detection, it offers promising applications:
- Clustering: Algorithms can group similar flower species based on their features, helping identify natural patterns within large datasets.
- Dimensionality Reduction: It reduces the complexity of datasets, making it easier for patterns to emerge, which is particularly useful when dealing with a high degree of variability in appearance across species.
One useful unsupervised learning technique is anomaly detection, which can identify uncommon species. By exploring data variability, unusual characteristics can signal new, perhaps rare, species requiring further study.
Unsupervised learning helps to uncover hidden structures in datasets, considerably enhancing the depth of analysis in flower detection.
Datasets for Flower Detection
Datasets play a critical role in the field of flower detection. They are essential for training and testing algorithms that help identify and classify different species. Without appropriately structured data, modern technologies such as computer vision and machine learning would lack the substance they need for accurate results. In diverse environments, flowers vary in appearance, making comprehensive datasets invaluable. This ensures that machine learning models can adapt to different conditions and accurately detect unique floral species.
Publicly Available Datasets
There are multiple publicly available datasets tailored specifically for flower detection. These datasets not only serve academic interests but also support real-world applications. For instance, the Oxford Flowers 102 dataset consists of 102 flower categories, housing more than eight thousand images. Another notable resource is the Fossil Plant Database, which, although primarily aimed at paleobotany, contains details about historical species that are significant in bioinformatics studies. Additionally, the Florabase serves as a record of plant species native to Australia, against which identification models can be validated. The breadth of publicly available datasets allows researchers to explore a rich collection of floral diversity.
Importance of Diverse Datasets
Diverse datasets ensure that machine learning models are robust and can generalize well across various environments. Models trained on a narrow dataset may struggle in different settings or with unexpected variations. Several benefits arise from a diverse dataset:
- Inclusive Representation: A diverse array of images increases the probability that a model can recognize flowers in different colors, shapes, and sizes.
- Improved Performance: A model trained on a more extensive range of data is likely to perform better in real-world applications, where unexpected variables may occur.
- Robust Models: Well-rounded datasets reduce bias. By including diverse examples, researchers mitigate the risk of the model favoring particular species over others.
In summary, having access to comprehensive and diverse datasets is paramount for successful flower detection. As technology progresses, continuous updates to these datasets will be necessary to encompass the evolving nature of floral diversity and enhance identification efficacy.
Real-World Applications
The field of flower detection has expanded well beyond academic research and theoretical applications. It plays a vital role in various industries and practices. Understanding how flower detection technologies manifest in real-world situations is crucial for recognizing their value and potential. Among the significant domains impacted are agriculture, conservation efforts, and urban planning.
Agriculture and Horticulture
In agriculture, flower detection systems are crucial for modern farming practices. These technologies can aid farmers in identifying flowering patterns in crops and wild plants. This knowledge helps in optimizing pollination processes, crucial for enhancing yield. For example, precision agriculture utilizes sensors and computer vision to monitor plant health automatically. Recognizing the growth stages of flowers can lead to more targeted fertilizer applications and pest control measures. Furthermore, horticulturists benefit by efficiently cataloging different flower species, making it easier to meet market demands and maintain biodiversity within their gardens.
Conservation Efforts
Conservation organizations utilize flower detection systems to monitor and support biodiversity. As many species face extinction, understanding their distributions and relationships with ecosystems becomes increasingly crucial. Automating flower identification through machine learning can assist researchers in gathering data from remote regions, often hiding diverse and at-risk plant species. This technology helps safeguard native plants and contributes to ecological surveys aimed at regeneration.
Additionally, using flower detection in conservation planning lowers costs and labor. Tracking changing habitats is vital for successful wildlife conservation, making the integration of technology even more important.
Urban Planning and Landscaping
Flower detection also finds its way into urban development and landscaping. As cities evolve, planners recognize the importance of green spaces infused with natural biodiversity. Appropriate flower detection can support in cultivating thought-out planting schemes for urban areas. Integrated with IoT programs, flower detection enhances monitoring ecosystem health within cities. Understanding which flowers thrive in urban environments assists in preventing issues such as invasive species.
Landscapers and city planners find ICT applications especially useful. Monitoring how plants adapt in various local contexts makes it possible to choose flowers better suited for public areas while promoting local flora. Ultimately, investing in flowering detection plays a critical role in enhancing urban sustainability.
Technological advancements in flower detection significantly influence many aspects of daily life, enhancing everything from agricultural practices to conserving crucial ecosystems.
In summary, the real-world applications of flower detection emphasize its capacity to contribute to agriculture, conservation, and urban environments. By harnessing technology and methodologies, these systems enrich our understanding of plant life and support various industries.
Challenges in Flower Detection
Detecting flowers involves various complexities that can hinder accurate identification. These challenges must be acknowledged and addressed to enhance the effectiveness of current detection methodologies. A deep understanding of these factors ultimately aids in improving flower detection systems. The identification process is inherently influenced by internal and external variables, which complicate the accurate recognition of flower species.
Variability in Species Appearance
One primary challenge lies in the variability among different flower species. This includes differences in size, shape, color, and patterns. Due to this variation, many species may look alike at casual observation. Moreover, environmental modifications can impact how flowers appear. Take two flower types that are closely related—under different lighting conditions or between seasons, they may exhibit markedly diffrent traits.
Several factors influence this variability:
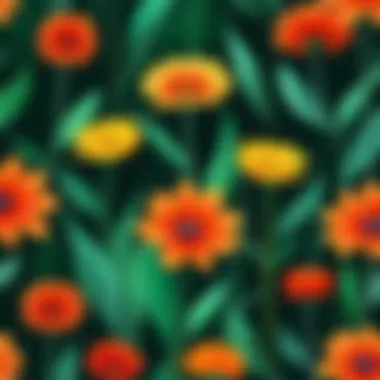
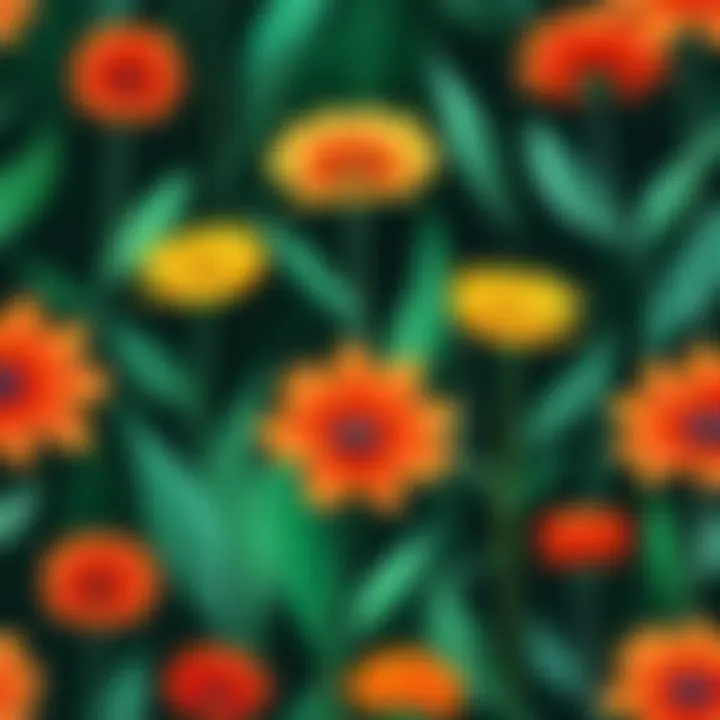
- Genetic Diversity: Different flowers of the same specied seem to show distinctions due to genetic predispositions.
- Phenotypic Plasticity: The environmental context may lead flowers evolving distinct traits. Exceptional changes include the way they bloom based on climate.
- Morphological Changes: Arrangements like petals and sepals may adjust according to their habitat. Variational aspects become pivotal to consider while developing accurate algorithms.
Environmental Factors Affecting Detection
Environmental conditions also greatly shape the detection of different flower species. Variations in light, climate, and terrain can all contribute to the difficulty in identifying flowers. The following conditions are particularly significant:
- Lighting Conditions: Lack of aqua colors and brighter shades easily myself distort the framing, making it difficult to capture the true colors during detection.
- Weather Conditions: Rain or high humidity levels may create fog or filtered brightness. These obstacles can affect camera sensors, thus leading to degraded image quality.
- Soil Type and Geography: Specific flowers may only grow in certain types of soil or regions, complicating predictions at the landscape level.
Understanding the interplay between these environmental factors and flower appearances is crucial. As research widens in the areas of machine learning and computer vision, tackling these challenges needs to refine algorithms and approaches for better identification rates. Technology constantly evolves but must keep addressing hurdles for optimum identification efficiency.
"Effectively addressing these challenges can lead to more robust flower detection methodologies."
Future Directions in Flower Detection
The field of flower detection is rapidly evolving, shaped by technological advancements and growing ecological awareness. The future directions in this domain hold significant prospects for improved plant identification and conservation efforts. These enhancements are not just a matter of convenience; they represent a deep integration of technology into our understanding of biodiversity.
Advancements in Deep Learning
Deep learning has emerged as a crucial pillar in flower detection. Leveraging layered neural networks, deep learning algorithms analyze vast amounts of floral imaging data. Techniques such as convolutional neural networks (CNNs) are providing better accuracy in identifying flower species. The advantage of using deep learning lies mostly in its ability to learn from examples. The more data the system has access to, the better it becomes at discerning subtle variations between different species. This increased precision greatly supports botanists and researchers in their quest for accuracy.
Advanced methods facilitate real-time identification and can contribute significantly to environmental monitoring as well. By incorporating robust deep learning techniques, we can elevate automated systems that monitor species while providing insights into population dynamics and habitat changes.
Integrating IoT Technologies
The internet of things (IoT) is progressively merging with flower detection methodologies, creating endless possibilities. With the integration of IoT, sensors placed in natural environments can collect vital information about climate, soil conditions, or even pollution levels. When paired with software utilizing computer vision or deep learning, a comprehensive understanding of a habitat's health can be achieved easily.
IoT devices can also enable remote monitoring of plant health and disease outbreaks dynamically. For instance, deploying sensor networks in fields could automate flower detection apps responsible for sending alerts concerning plant anomalies. This functions almost like a 'smart garden' system. The interplay of IoT devices with advanced identification methods can radically shift how we interact with and care for natural ecosystems.
“Integrating IoT with flower detection systems opens pathways to unprecedented levels of ecological insight.”
Overall, exploring these future directions, such as advancements in deep learning and the incorporation of IoT technologies, will yield profound impacts for conservationists and curators of biodiversity. The affirmative movements towards improved identification processes will guide both academic research and practical applications, where understanding plant life becomes poised at that technologically engaged equilibrium.
Cultural Significance of Flowers
Flowers carry a profound meaning across different societies and settings. They are not just objects of beauty; they symbolize emotions, beliefs, and cultural practices. Understanding this cultural significance is essential in appreciating the wider impact of flower detection technologies and methodologies that we discuss in this article. The role flowers play in varied contexts can enhance biodiversity understanding and conservation efforts.
Flowers in Art and Literature
Throughout history, flowers have held a prominent place in art and literature. They are infused with symbolism that often transcends simple representation. For example, roses are commonly associated with love and passion, while lilies may symbolize purity and renewal. Artists such as Vincent van Gogh captured the striking beauty of blossoms in works like "Sunflowers," which evoke emotions through their vibrance.
Literature also fosters a rich tapestry of floral symbolism. Poets like William Wordsworth have used flowers as metaphors for deeper themes, such as the transient nature of life. In the realms of storytelling, flowers often serve as important motifs too. They symbolize love, death, and rebirth, reflecting human experiences and emotions. A study of these connections not only enriches our understanding of art and narrative but also shapes how technology can further unlock this cultural heritage.
Traditional Uses Across Cultures
Flowers have multifunctional roles depending on the culture. In many societies, they are used in ceremonial occasions. For instance, marigolds feature prominently in Hindu traditions during celebrations like Diwali, symbolizing light and joy. Similarly, native cultures have used local flowers for medicinal purposes, showcasing their importance in traditional healthcare.
Moreover, flowers interconnect with various celebrations and rituals worldwide. Wedding bouquets symbolize love and commitment, while funeral arrangements represent solemnity and grieving. Understanding these traditional uses helps reinforce ecosystems' significance and motivates targeted detection strategies in conservation contexts.
Studies show that recognizing cultural aspects of flowers can contribute to more effective community engagement in biodiversity conservation efforts.
In essence, the significance of flowers in cultural contexts accumulates profound insights that impact the fields of art, literature, religion, and conservation. Their diverse applications across cultures emphasize the relevance of accurate identification methods. The continued exploration of this topic paves the way toward better integration of technology with cultural practices.
Ending
The conclusion of this article encapsulates the importance of contemporary techniques used in flower detection. Flower identification is vital for multiple reasons: ecological balance, biodiversity conservation, and cultural appreciation. The growing relevance of technology in this field enhances not only our understanding of ecological systems but also the methods used in improving agricultural productivity and managing urban landscapes.
Summary of Key Insights
Throughout this article, several critical insights have emerged regarding flower detection methodologies. Key points include:
- The importance of building accurate identification tools for both technological and ecological success.
- The role machine learning and computer vision play in advancing flower detection capabilities.
- A look into datasets that fuel refined algorithms, enhancing real-world applications.
- An understanding of challenges such as variability in species appearances and environmental influences on identification accuracy.
These elements symbolize the intertwined nature of technological advancement and environmental stewardship. Addressing both bottlenecks and breakthroughs lays down a foundation for further investigations in this field.
Looking Ahead: The Role of Technology
As we gaze into the future of flower detection, the integration of advanced technologies stands out. Some specific trends include:
- Deep Learning Advances: Increasingly sophisticated models are becoming adept at recognizing subtle differences in flower species, leading to even more accurate identification. Enhanced computational power and data availability should support myriad applications and research possibilities.
- IoT Technologies: Employing sensor-based devices can provide real-time data on various environmental conditions. The merging of flower detection tech with Internet of Things advances is set to provide unprecedented insights fostering beneficial alphabetical practices.
There is enormous potential in the fusion of biology and technology. Therefore, engaging with fresh insights continuously drives both conservation efforts and technological progress. Maintaining vigilance on developments in this realm enables informed decision-making for the larger good.