Crafting Personalized Recommendations in Modern Industries

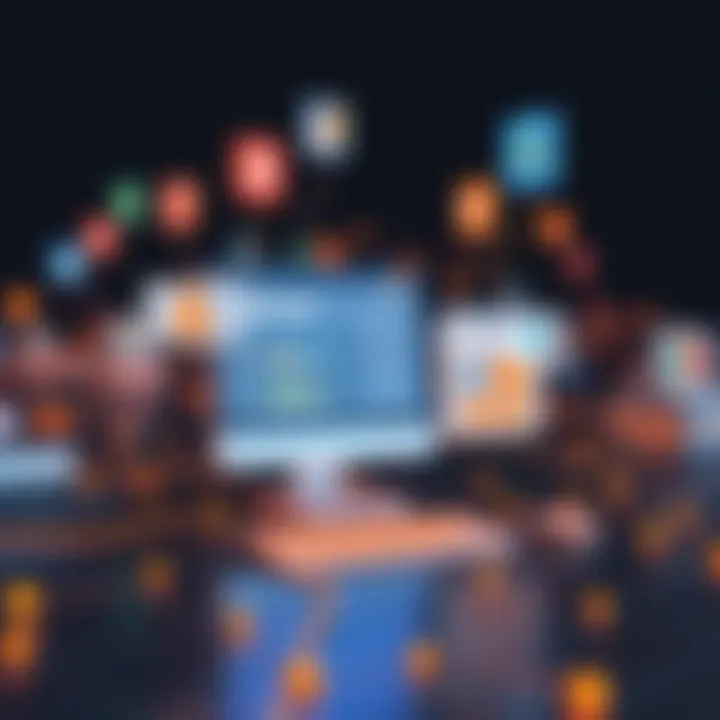
Intro
In the modern landscape of technology, entertainment, and design, the art of crafting tailored recommendations has transformed how we interact with content and products. Tailored recommendations are not just a passing trend; they are an essential part of how companies engage customers and shape their experiences. From Netflix deciding which shows will keep you glued to your screen, to Spotify curating playlists based on your listening habits, personalization is everywhere.
This intricate system of recommendations relies on complex algorithms and data analysis that strive to understand individual preferences and behaviors. The ultimate aim? To create an engaging and seamless user experience that anticipates needs and desires before they even enter the conscious thought. By exploring key aspects of this topic, we will see how personalized recommendations shape our choices, impact industries, and redefine user engagement.
Technology Insights
Latest Tech Trends
As personalization takes the digital world by storm, several tech trends stand out. Machine learning, for instance, is at the forefront. This technology allows platforms to analyze vast amounts of user data to identify patterns and preferences. Itâs not just about collecting dataâit's about converting that data into actionable insights.
Moreover, the rise of artificial intelligence is reshaping recommendation systems. AI can adjust to user behavior over time, creating an evolving experience that feels uniquely tailored. Users today expect their favorite apps to understand them much like a friend would. Whether this is by suggesting new gadgets, apps, or services, AI-driven recommendations are undoubtedly here to stay.
Innovation in Tech
Innovation manifests not just in the development of new technologies, but also in the application of existing ones. Consider the growth of voice-activated assistants like Amazon's Alexa or Apple's Siri: these technologies are now capable of providing recommendations based on previous interactions. For example, if you often ask for book suggestions, your assistant learns and begins to provide tailored lists that may surprise you.
Product Reviews
When it comes to recommended products, trusted reviews enhance the decision-making process. Websites like Reddit and specialized forums play a big role, as community feedback can offer more nuanced insights than traditional advertising. This organic word-of-mouth is a powerful catalyst for products to reach the right audience.
Entertainment Highlights
Movie Reviews
In the realm of entertainment, tailored recommendations have dramatically transformed the way audiences discover films. Services like Netflix and Hulu leverage sophisticated algorithms that analyze viewing patterns, allowing them to suggest movies that align with a user's taste. This means not just the top hits, but hidden gems that viewers may have never considered.
Music Releases
Similarly, Spotify stands out with its Discover Weekly feature that learns from your listening habits. Each week, a fresh playlist tailored specifically for you appears, introducing you to new artists and tracks, often so in tune with your musical preferences that it feels like magic. As listeners grow accustomed to personalized experiences, platforms must continuously innovate to keep their offerings fresh and engaging.
Celebrity News
The consumption of celebrity news has also been impacted. Social media platforms, notably Facebook and Instagram, utilize algorithms that pick up on what content you engage with the most, guiding you towards news that feels curated just for you, be it celebrity gossip, fashion trends, or significant life events. The digital age has enabled a more direct line between celebrities and fans, reinforcing loyal followings.
Design Showcase
Creative Designs
In the design world, personalization fosters innovation. Websites like Pinterest allow users to create boards tailored to their taste, identifying trends and preferences. This platform enables people to discover design ideas that cater precisely to their aesthetic, leading to rich creative expression.
Architectural Trends
When it comes to architecture, recommendation systems can direct homeowners towards styles that resonate with their values. Whether opting for sustainable materials or modern minimalist aesthetics, users are bombarded with tailored suggestions that match their vision. Each design becomes a reflection of individual stories and preferences.
Graphic Design Inspiration
For graphic designers, platforms like Behance have become invaluable resources for inspiration. Here, algorithms showcase projects that align with a designerâs past interests, providing an endless stream of innovation. This not only aids in skill development but also fosters community collaboration.
Industry Spotlights
Interviews with Tech Experts
Understanding the nuances of tailored recommendations requires insights from industry experts. Interviews can reveal the challenges they face, such as the ethical implications of data privacy and the balance between personalization and user comfort.
Behind-the-Scenes in Entertainment
Behind-the-scenes insights offer an eye into how recommendations are made in the entertainment sector. From data scientists analyzing user trends to producers strategizing on content placement, this deep dive can provide a unique perspective into the operational mechanics that drive personalization.
Designers to Watch
The creative field is filled with designers pushing boundaries. Profiles of emerging talents give readers a snapshot of the future of design, while their stories highlight how personalized tools helped them achieve their unique vision and style.
Event Coverage
Tech Conferences Report
Attending tech conferences reveals a treasure trove of knowledge surrounding recommendation systems. Presentations often focus on the future of personalization in tech and user experience, making these events vital for any industry insider.
Entertainment Awards Show Recap
Entertainment awards shows provide a fascinating context for understanding audience trends. The winners often align with shifts in popular recommendations as they highlight which films and music captured the audience's heart, guiding viewers on what to engage with next.
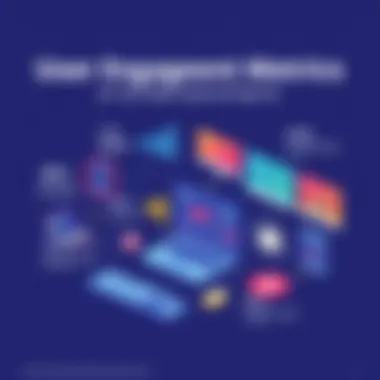
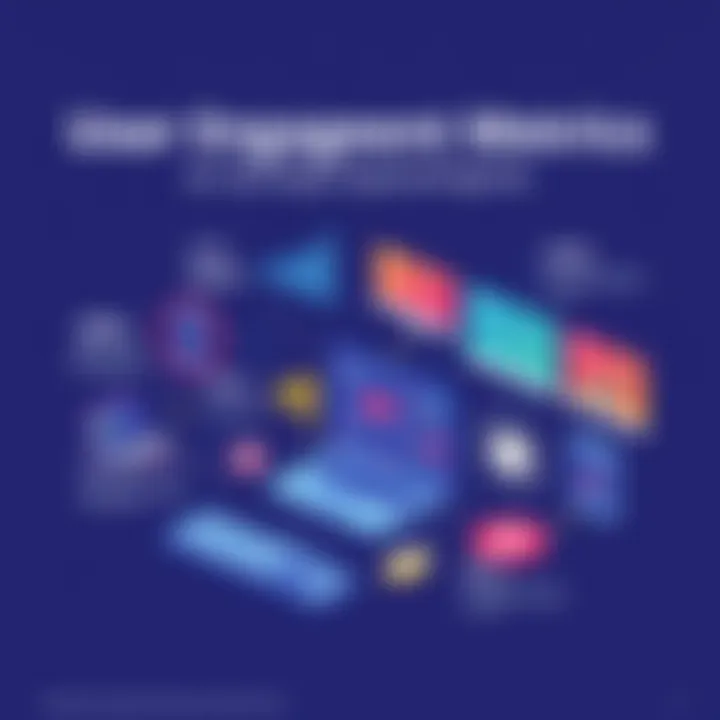
Design Exhibitions Highlights
In the design field, exhibitions showcase cutting-edge innovations. They reveal how personalized design strategies are evolving and how they interact with modern consumer behaviors, highlighting trends that attendees can directly apply to their own practices.
Understanding Recommendations
In the rapidly evolving landscape of technology, entertainment, and design, understanding recommendations is paramount. These suggestions serve as the bridge between consumers and the goods or services that resonate with their interests and needs. By grasping the nuances of recommendation systems, businesses can enhance customer satisfaction and bolster loyalty. Moreover, these tailored recommendations can significantly impact purchase decisions, transforming how consumers interact with products and services.
Personalized recommendations are not merely a convenience; they are a critical component in driving engagement. When done right, they can lead users down a path of discovery, subtly guiding them towards choices that complement their preferences. For example, when a user logs onto a streaming platform, the initial offerings can often dictate their viewing experience. If the recommendations align closely with their interests, it creates a positive feedback loopâa satisfied viewer returns for more.
Key Considerations about Understanding Recommendations:
- User-Centric Approach: Implementing recommendations that are based on user behavior and preferences ensures relevance and increases the likelihood of engagement.
- Cultural Sensitivity: Understanding the overarching cultural context can tailor recommendations even further, making them resonate well with distinct demographics.
- Data Utilization: Striking the balance between user data gathering and privacy concerns presents challenges, but effective recommendations are grounded in a solid foundation of data analysis.
"To know what users want is to unlock a treasure trove of opportunities that can shape businesses and enhance user experiences."
These aspects illuminate how recommendation systems serve to elevate consumer experiences in a world clamoring for personalized interactions. As industries continue to embrace and refine these practices, the understanding of tailored recommendations will remain an essential ingredient to successful engagement strategies.
Historical Context of Recommendation Systems
Understanding the historical roots of recommendation systems plays a crucial role in comprehending their present and future landscape. These systems didn't just pop up overnight; they evolved through various stages, reflecting technological advancements and shifts in consumer behavior. By exploring this context, one can appreciate the significance of tailored recommendations in our daily interactions with technology, entertainment, and design. As we dig into the annals of recommendation systems, it becomes clear that they represent an ongoing dialogue between user preferences and the algorithms designed to meet them.
Evolution Over Time
The journey of recommendation systems began in the early days of computing when the notion of personalizing user experience was still a nascent idea. The foundational concept can be traced back to simple rule-based systems that relied on user input to suggest items. These early systems, although basic, laid the groundwork for understanding how preferences could inform choices.
As technology marched forward, the 1990s introduced collaborative filteringâan approach that was a game-changer. This method looked beyond individual preferences and started analyzing the behavior of groups. Institutions like Netflix and Amazon rode this wave, utilizing collaborative filtering to drive their recommendation engines, suggesting that if two users shared similar tastes, they would likely appreciate the same films or products.
Transitioning into the 2000s, the rise of big data transformed the landscape even further. With the exponential growth of user-generated data from platforms like social media, companies began leveraging sophisticated algorithms to sift through this sea of information. This era marked a pivotal shift where micro-targeting emerged, leading to hyper-personalization and tailored content delivery.
In contemporary settings, machine learning and artificial intelligence have taken center stage. These technologies analyze complex data sets, enabling systems to predict preferences with remarkable accuracy. Nowadays, a quick scroll through a streaming service like Spotify shows how far weâve comeârecommendations appear almost intuitively but are the result of years of evolution.
From Manual to Automated Systems
The shift from manual to automated recommendation systems marks a significant paradigm shift in how we interact with technology. Initially, recommendations were largely manual. Think back to the days when a bookstore clerk would suggest titles based on your last purchase or a friend's advice would determine which movie to watch. Through human interaction, a sense of connection and trust formed, but it was inherently limited by the clerk's or friendâs knowledge.
As digital technologies grew, this model became cumbersome. Automated systems began to emerge, and while they promised efficiency, they also raised new questions about trustworthiness and satisfaction.
Some benefits of automated systems include:
- Scalability: They can suggest content to millions of users simultaneously.
- Speed: Instantaneous recommendations make for a seamless user experience.
- Data-Driven: Automated recommendations rely on vast data analysis, improving accuracy over time.
However, challenges accompany this transition. The reliance on data can lead to algorithmic biasâpreferences may skew towards more popular choices, sidelining niche interests. Moreover, the disconnection from human touch sometimes leaves users feeling like just another number on a screen.
It is crucial to strike a balance between technology and human insight. Automated systems can still use user feedback loops to enhance their efficacy, creating a more holistic approach to recommendations. This evolution is ongoing, and understanding it provides foundational knowledge as we traverse an increasingly automated future in technology, entertainment, and design.
âThe future of recommendations lies at the intersection of human and artificial intelligence, weaving a narrative that is both personalized and intuitive.â
Types of Recommendation Approaches
In the realm of tailored recommendations, understanding the various approaches is pivotal for creating a personalized experience. Users expect specific content tailored to their preferences, making this aspect an essential consideration in various platforms ranging from e-commerce to streaming services. Knowing the types of recommendation approaches helps businesses enhance user engagement, improve satisfaction rates, and ultimately drive conversion rates. These systems utilize algorithms to sift through data and deliver content that resonates with individual users, ensuring that their journeys through digital landscapes are both seamless and enjoyable.
Content-Based Filtering
Content-based filtering operates on the principle that users will favor items similar to those they've liked in the past. This approach analyzes the features of the items themselvesâbe it articles, movies, or productsâto determine relevance. For example, if a user has shown interest in sci-fi films, the system discerns key characteristics such as genre or director, and then suggests other related movies.
A notable advantage of content-based filtering is its transparency; users usually understand why they are receiving certain recommendations. Additionally, it's effective in scenarios where users have distinct preferences and prior behaviors, as it doesn't depend on the actions of others. This can often lead to a more curated experience that genuinely aligns with user taste.
While content-based filtering shines in many situations, it's not without its drawbacks. One significant limitation is the so-called "filter bubble," where the system may inadvertently restrict exposure to diverse content. A user may end up only seeing similar items over time, potentially stifling discovery of new interests.
Collaborative Filtering
Collaborative filtering, in stark contrast to its content-based counterpart, leverages user behavior data rather than item characteristics. It operates on the underlying assumption that if two users have shown similar preferences in the past, they are likely to enjoy the same content in the future. The system collects vast amounts of user interaction dataâratings, clicks, and purchasesâthen identifies patterns to suggest items that other like-minded users have enjoyed.
This approach often leads to highly relevant suggestions that surprise and engage users. For instance, Netflix utilizes collaborative filtering to recommend shows and films by drawing connections between user ratings and viewing histories. When done right, collaborative filtering can vastly expand a user's horizons, introducing content they might not have considered otherwise.
Nevertheless, collaborative filtering does face significant challenges, particularly in its early stages of implementation, known as the cold start problem. When a new item is added or a new user joins a platform, there is insufficient data available to generate reliable recommendations. This can make the experience frustrating for newcomers, as it can feel like they are being overlooked.
Hybrid Systems
Hybrid systems are where the true magic often happens in recommendations. By combining the strengths of both content-based and collaborative filtering methods, these systems strive to provide a richer and more accurate personalized experience. They minimize the weaknesses of each approach by drawing from multiple data sources.
For instance, a hybrid recommendation engine can suggest new movies based on both a user's previous likes (content-based filtering) and what similar users have enjoyed (collaborative filtering). This dual approach can yield results that are far more engaging and varied, helping to keep users hooked on the platform.
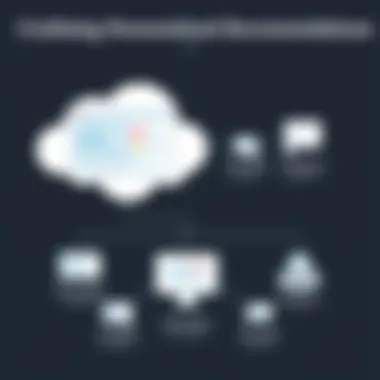
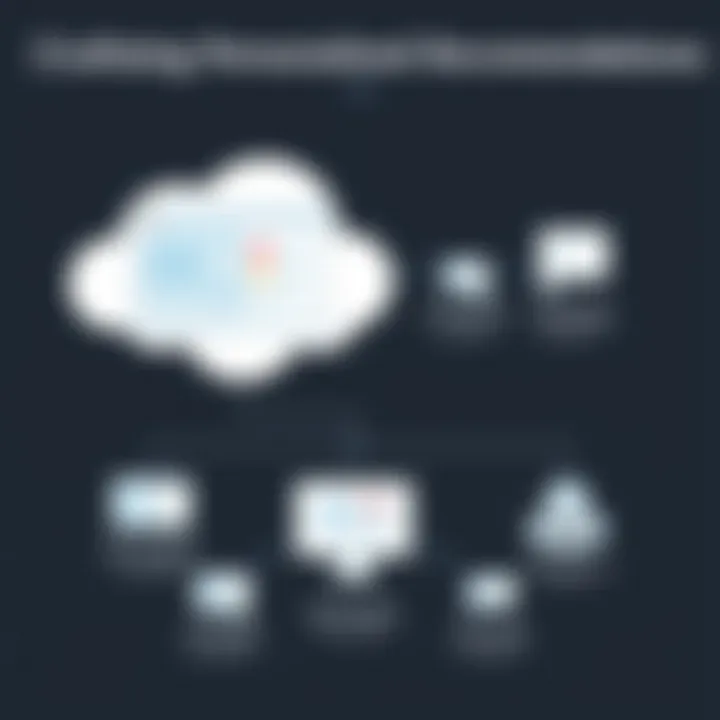
Moreover, hybrid systems can continually evolve to incorporate real-time user feedback, allowing for adjustments in recommendations based on dynamically changing preferences. This flexibility is vital in todayâs fast-paced digital environment where tastes can shift rapidly.
"Personalized recommendations are the heart and soul of user satisfaction in modern digital experiences."
Applications in Technology
The advent of tailored recommendations has revolutionized how users interact with technology. In a world overflowing with choices, these recommendations serve as valuable guides that help individuals navigate through an overwhelming array of options. The essence of this article is to shed light on the multifaceted applications of recommendations in technology, particularly how they drive engagement, enhance user satisfaction, and improve decision-making processes.
Personalized recommendations rest on the concept of understanding individual user preferences and behaviors. This is crucial in several realms, notably e-commerce platforms, streaming services, and social media networks. Letâs take a closer look at how these applications manifest and why they matter.
E-commerce Platforms
In the bustling realm of e-commerce, tailored recommendations are paramount. Amazon exemplifies this beautifully; the platform utilizes past purchases, browsing histories, and even items people frequently glance at. This enables it to showcase products that a user is likely to consider, drastically reducing the time they spend searching for what they need. But it goes further than just boosting salesâit enhances user experience by presenting options that feel specially curated for each shopper.
Furthermore, the concept of upselling and cross-selling finds its home in recommendations. Consider the journey of a customer purchasing a new phone. They might receive suggestions for cases, chargers, or even songs popular among other users of that specific phone model. This not only enhances the customerâs buying experience but also increases average order values. In fact:
- Higher Conversion Rates: Personalized suggestions can lead to a significant uptick in sales.
- Customer Retention: Satisfied shoppers are more likely to return for future purchases.
- Reduced Return Rates: By informing customers with tailored recommendations, they may make better purchasing decisions, curbing the rate of returns.
Streaming Services
When it comes to streaming services, like Netflix, recommendations take on a whole new flavor. Algorithms meticulously analyze viewing habits, preferred genres, and even the time of day a user typically watches to create a tailored playlist unique to every individual. The line âWhat to Watch Next?â resonates deeply with usersâthese platforms strive to minimize decision fatigue by serving up shows that they know viewers are likely to enjoy.
The art of 'binge-watching' is elevated by these recommendations, as users often find themselves engrossed in series they may have overlooked without this guidance. The implications are powerful:
- Enhanced User Engagement: The more aligned the content is with the user's interests, the longer they stick around.
- Content Discovery: Users are introduced to diverse titles, expanding their viewing habits.
- Data-Driven Insights: Streaming services gain a better grasp of trends and patterns in viewership, helping with future content creation.
Social Media Networks
Social media platforms like Facebook rely heavily on tailored recommendations to cultivate meaningful online interactions. From friend suggestions to curated news feeds, the underlying algorithms assess user interactionsâlikes, shares, and commentsâto offer content that resonates. This level of personalization bewilders users because suddenly, they are presented with discussions, articles, or even events that feel relevant to them personally.
However, just like a double-edged sword, these recommendations pose challenges. Users sometimes confront a filter bubble, where they are only exposed to ideas that align with their past interactions, potentially stifling diverse experiences. However, the benefits remain significant:
- Increased User Interaction: Engaging content fosters a sense of community and encourages more participation.
- Targeted Advertising: Businesses can reach their ideal audiences through tailored ads, improving efficacy and return on investment.
- Sustained Platform Loyalty: With relevant content flowing, users are inclined to spend more time on these platforms, which is precisely what social media companies desire.
As we dissect the interplay between technology and user experience further, the next sections will delve deeper into how these systems function and the underlying technology that fuels them.
Influence on Entertainment Choices
In today's fast-paced digital landscape, the choices we make in entertainment are heavily influenced by tailored recommendations. With endless options available, consumers often find themselves overwhelmed, thus the role of recommendation systems cannot be overstated. These systems efficiently sift through vast amounts of data to provide personalized content, often becoming the guiding hand in the decision-making process.
The primary benefit here lies in enhancing user experience. By suggesting movies, shows, or music based on individual preferences, these systems tailor each child's viewing or listening experience. This level of personalization not only keeps users engaged but also saves time that could be spent browsing endless lists.
Furthermore, intelligent recommendation systems encourage deeper exploration into diverse genres and niches that a user may not have considered on their own. Itâs like discovering hidden gems under thick layers of sand. When users feel understood, they are likely to return, ultimately increasing loyalty to platforms that excel in these efforts.
"Personalized recommendations are the new digital breadcrumbs leading us through the forest of content."
A crucial consideration in this domain is how recommendations impact consumption patterns. Understanding these choices allows creators to anticipate audience needs better, shaping their offerings in ways that resonate more effectively. With recommendation engines acting as gatekeepers, consumers may be exposed to more of what they enjoy, or alternatively, what they didn't know they needed. However, with great power comes significant responsibility; it's essential for companies to continually assess their algorithms to ensure they are not fostering echo chambers.
In summary, tailored recommendations mark a significant turning point in how entertainment is consumed, ensuring that the user experience remains at the forefront of digital engagement. The influence of such personalized systems is profound, steering choices and cultivating healthy entertainment habits.
Design Considerations for Recommendations
Designing effective recommendation systems isnât just about algorithms crunching numbers; it also revolves around an ergonomic design that ensures users donât feel lost in a sea of options. The art lies in creating a seamless interface and enriching user experiences that keep individuals engaged while searching for whatâs relevant to them.
In crafting tailored recommendations, several design elements must be considered: how information is displayed, the aesthetic of the interface, and the interactions user have with the recommendations. Each aspect plays a pivotal role in shaping user behavior and acceptance of the suggested content.
The benefits are manifold. A user-friendly design can improve conversion rates, enhance customer retention, and foster loyalty by making users feel understood and valued. Consider the difference between a plain text list of recommendations and a visually engaging grid with images and descriptions that draws users in. Users are more likely to engage with attractive designs because it creates a positive first impression.
The interplay between design and functionality is crucial; an elegant aesthetic can elevate user trust, making them more receptive to recommendations.
User Interface Design
The user interface (UI), which encompasses the buttons, menus, and visual layout, forms the first point of contact for users. A well-thought-out UI can guide users seamlessly through the process of discovering new content, whether in e-commerce or streaming services. Simplicity and clarity are key tenets to keep in mind.
- Visual Hierarchy: Organizing information such that important recommendations stand out promotes natural user flow. This can be achieved by adjusting sizes, colors, and placements.
- Responsive Design: With users accessing content from various devices, the interface must adapt to different screen sizes without losing functionality. Failure to do so often leads to frustration.
When users feel they can navigate the interface intuitively, they are more likely to revisit platforms. A case in point could be Netflix, which consistently provides an engaging interface that instantaneously communicates personalized options to users, thus fostering continued engagement.
User Experience Enhancements
User experience (UX) is about creating a broader narrative that resonates with the users beyond mere interaction. It transcends the interface and delves into the journey users take while they explore recommendations. Enhancing UX involves paying attention to subtle details that can greatly affect overall satisfaction.
- Personal Touch: Tailoring feedback based on user activity, such as viewing history and preferences, can increase the relevancy of recommendations. Users appreciate personalized messages that resonate with their tastes.
- Feedback Loops: Implementing simple mechanisms for users to rate recommendations fosters a two-way interaction. Providing choices not only empowers users but also allows systems to refine their models based on user preferences.
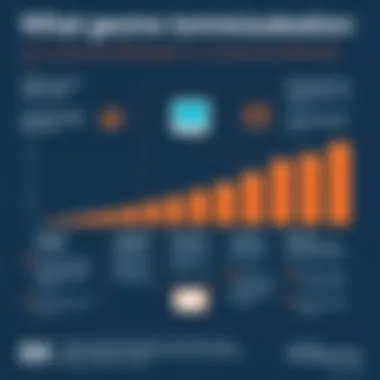
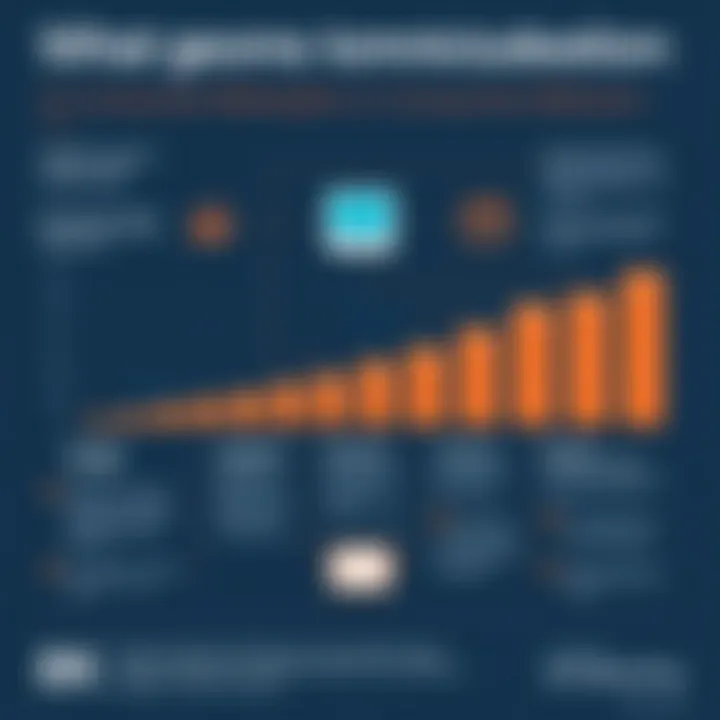
Ultimately, the goal should be to make users feel as though the content was just meant for them. An effective recommendation system can increase interactivity and retention significantly, leading to an enriched user journey. A well-designed recommendation interface coupled with meaningful enhancements can create a powerful ecosystem that encourages exploration and connection.
Technologies Driving Recommendations
The landscape of tailored recommendations has been revolutionized by cutting-edge technologies. These advancements enable platforms to analyze vast amounts of user data, ensuring that suggestions are not just random but finely tuned to individual preferences. In a world where choices abound, the ability to receive curated content can make or break user engagement. Technologies such as machine learning and big data analytics form the backbone of this personalization, enhancing user satisfaction and contributing to overall success in tech, entertainment, and design sectors.
Machine Learning Techniques
Machine learning is a linchpin in the development of recommendation systems. It empowers applications to learn from data patterns over time, refining their suggestions based on user behaviors and interactions. Here, algorithms are not simply programmed to execute tasks; they evolve by learning from the feedback loop created by user actions. For example, Netflix employs algorithms that analyze what shows users watch, how long they watch them, and even their ratings. This information feeds back into the system, enabling it to suggest similar content with increased accuracy.
Key components of machine learning techniques include:
- Supervised Learning: Models like collaborative filtering use existing user-item interactions to predict what a new user might like based on others with similar tastes.
- Unsupervised Learning: Clustering techniques help group similar items or users, finding unseen relationships within the data.
- Reinforcement Learning: Systems can also adapt dynamically based on user interactions in real-time, optimizing recommendations as users engage with content.
"The art of recommendation lies in not just what you say, but in how well you listen to your data."
In practical terms, this means that platforms can offer increasingly relevant suggestions tailored to individual needs. Furthermore, as machine learning models improve, they become capable of understanding subtle intricacies in user preferences, allowing for even finer recommendations.
Big Data Analytics
Big data analytics complements machine learning by providing the raw materials needed for these systems to function effectively. The sheer volume of data generated by users interacting with technology, media, and design platforms offers a treasure trove of insights. This data can encompass everything from clicks and likes to time spent on specific pages and demographic information.
Key advantages of leveraging big data analytics include:
- Enhanced User Profiles: Analyzing data points helps construct detailed user profiles, helping services understand customers at a granular level. This results in personalized advertising, content, or product recommendations that resonate uniquely with each individual.
- Trend Prediction: By parsing through large sets of data, businesses can spot emerging trends, allowing them to stay ahead of the curve and offer timely recommendations.
- Behavioral Insights: With the ability to track user behavior over time, companies can better understand not just what users want, but why they prefer certain content, allowing for a more immersive experience.
Combining machine learning and big data analytics creates a potent synergy in recommendation systems. While machine learning hones the algorithms to provide tailored suggestions, big data supplies the essential information. Together, they form the bedrock of an effective and engaging user experience, ensuring that technology, entertainment, and design platforms remain relevant and user-centric.
Challenges in Recommendation Systems
The arena of tailored recommendations involves much more than simply feeding data into an algorithm. Itâs a double-edged sword: while these systems offer opportunity for personalization, they also grapple with significant hurdles. Understanding these challenges is crucial for any tech-savvy individual or industry professional aiming to improve user experience or foster engagement. Here, weâll dive into common issues such as data privacy and algorithmic bias, highlighting why they matter and how they can impact the design of recommendation systems.
Data Privacy Concerns
Data privacy is at the forefront of discussions surrounding personalized recommendations. As technology becomes pervasive, consumers increasingly leave behind digital footprints across various platforms. These data pointsâranging from search history to purchase behaviorâare the gold mines that recommendation engines rely on. However, the quest for personalization raises serious concerns about user consent and data protection.
- User Trust: As users interact with e-commerce platforms, streaming services, and social networks, they often remain unaware of how their personal information is being harvested. Lack of transparency can lead to distrust, ultimately driving users away from platforms that donât prioritize data security.
- Regulatory Frameworks: Global regulations such as GDPR in Europe and CCPA in California have established strict guidelines for how user data can be collected and used. Companies must navigate these laws carefully or face hefty fines, which complicates the design of recommendation systems.
"Trust and transparency are the bedrock of effective recommendation systemsâa product can only recommend well if it has the userâs trust."
To tackle data privacy issues, developers and companies must prioritize user education, enhancing transparency by informing users about data usageâwhy it's collected, how it's stored, and for what purposes itâs employed. Balancing personalization with privacy is no small feat; yet, itâs a necessary art to master for successful recommendation systems.
Bias in Algorithms
Another critical challenge in creating effective recommendation systems lies in biasâboth in the data and the algorithms that analyze it. When biases seep into recommendations, they can misrepresent user preferences and skew results. This is particularly relevant in sectors like entertainment and social media, where the effects of bias can be pronounced.
- Data Input: Algorithms are trained on historical data, which might carry the weight of societal biases or limitations. For instance, if a music recommendation system is trained on a dataset lacking diversity, it may reflect this in its output, favoring certain genres or artists over others.
- Reinforcement of Echo Chambers: Algorithms often recommend content similar to what users have previously engaged with. While this may feel convenient, it can lead to reinforced echo chambers, isolating users from varied perspectives and experiences.
To combat algorithmic biases, developers should focus on creating diverse datasets and learning models that can account for various user demographics and preferences. Striving for inclusivity in recommendation engines is not only ethical, but can also enhance overall user satisfaction and broaden engagement.
In essence, addressing these challenges is essential for the evolution of tailored recommendation systems. As the technology and its applications grow, understanding the intricacies of data privacy and bias in algorithms will remain paramount in creating systems that are not only effective but also responsible.
Future Trends in Recommendations
Understanding the future trends in recommendation systems is essential for grasping how technology will evolve in its effort to tailor user experiences. The advancements in this field are not just about enhancing efficiency but also about reimagining how users interact with platforms across technology, entertainment, and design. The significance of these trends lies in their potential to reshape customer engagement and satisfaction, thus reshaping entire industries.
Increasing Automation
The drive towards increased automation in recommendation systems is a game changer. Companies are leveraging automation to enhance the agility of their response to user preferences. Machine learning algorithms are evolving to automate the analysis of user data without requiring extensive human intervention. This shift is crucial in an age where large volumes of data are generated every second. Automating the recommendation process allows for more timely and relevant suggestions to users.
Imagine you just finished a thrilling series on a popular streaming platform like Netflix. Instead of waiting for your next favorite show to be programmed or curated to you, the system automatically understands your viewing habits. It can even predict your next watch based on your mood and preferences derived from previous interactions.
The key benefits of incorporating automation into recommendation systems include:
- Efficiency: Automated systems process information quickly, providing suggestions almost instantaneously.
- Personalization at Scale: They can analyze thousands of user profiles simultaneously, tailoring unique experiences for each individual.
- Reduced Operational Costs: Less need for manual curation can save companies significant resources, allowing them to invest in other areas.
As automation integrates itself into recommendations, user interaction will shift from passive consumption to active engagement.
Impact of AI Development
The growth of artificial intelligence is revolutionizing recommendation systems and, by extension, user experience. AI empowers systems to learn from data dynamically, which means algorithms can adapt to changing user tastes over time. This adaptability introduces a new layer of personalization that traditional methods couldn't achieve.
Consider a music streaming service like Spotify, which employs AI to create tailored playlists. The platform doesn't just rely on past downloads or listens; it gauges your listening habits each day, factoring in new releases, seasonal changes, or even trending music worldwide. AI algorithms can analyze your reaction to various genres and themes and refine recommendations accordingly.
The implications of AI in recommendation systems are vast:
- Enhanced Predictive Accuracy: AI systems can predict user preferences with far greater accuracy than simple algorithmic approaches.
- Real-Time Adaptation: These systems can constantly adjust recommendations in real time, ensuring that the content remains relevant to the user.
- User Retention: By consistently providing intuitive and relevant content, businesses can enhance user loyalty and keep audiences coming back for more.
The integration of artificial intelligence in recommendation systems not only enhances personalization but also fosters a deeper connection between users and platforms.
In summary, as we navigate the path into the future, the trends in recommendations will highlight the need for continual adaptation and improvement. With increasing automation and the rise of AI, users can expect a more seamless interaction that curates experiences tailored specifically to them.